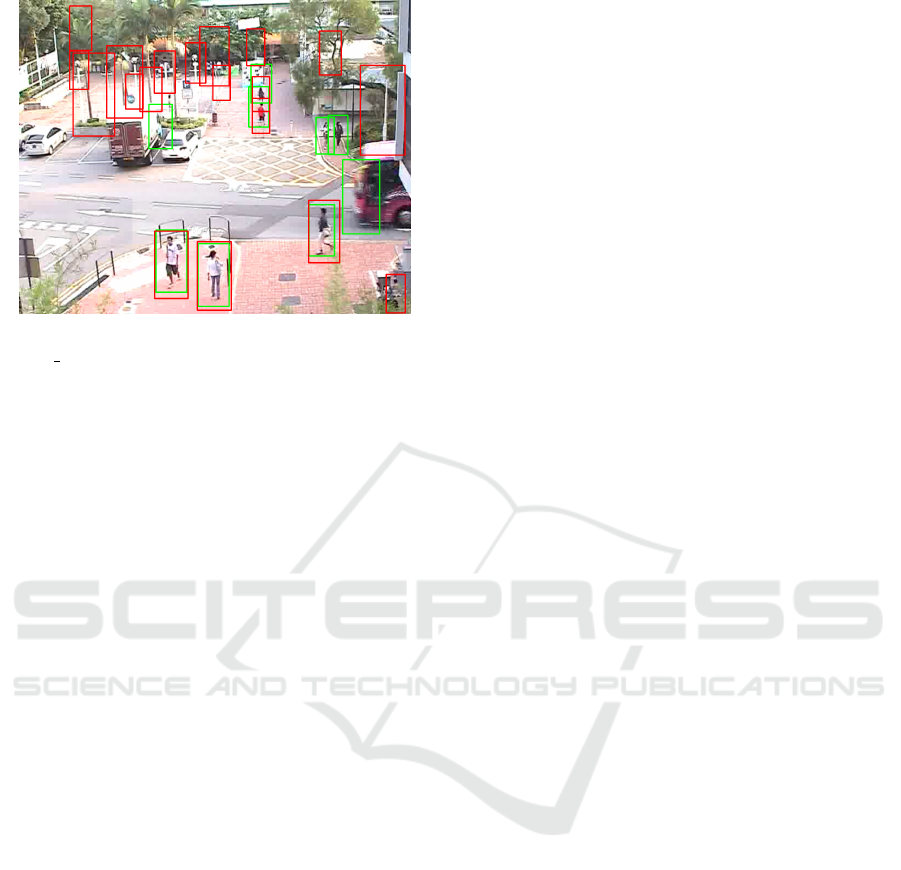
Figure 8: Illustration of the specialization result on the
CUHK Square dataset. The red bounding boxes and the
green bounding boxes present the outputs of the generic and
specialized detector, respectively.
the HOG-SVM classifier. The experiments have
shown that the proposed specialization framework has
good performances from the early iterations on two
public datasets.
Future works will deal with an extension of the
algorithm to a multi-object framework. Furthermore,
the observation function may be ameliorated with
more complex visual cues like tracking, optical flow
or contextual information.
ACKNOWLEDGEMENTS
This work is supported by a CIFRE convention with
the company Logiroad and it has been sponsored
by the French government research programme
”Investissements d’avenir” through the IMobS3
Laboratory of Excellence (ANR-10-LABX-16-01),
by the European Union through the programme
Regional competitiveness and employment
2007-2013 (ERDF Auvergne region), and by
the Auvergne region.
REFERENCES
Aytar, Y. and Zisserman, A. (2011). Tabula rasa: Model
transfer for object category detection. In ICCV, pages
2252–2259. IEEE.
Chesnais, T., Allezard, N., Dhome, Y., and Chateau, T.
(2012). Automatic process to build a contextualized
detector. In VISAPP, volume 1, pages 513–520.
SciTePress.
Dai, W., Yang, Q., Xue, G.-R., and Yu, Y. (2007). Boosting
for transfer learning. In ICML, pages 193–200. ACM.
Dalal, N. and Triggs, B. (2005). Histograms of oriented
gradients for human detection. In CVPR, volume 1,
pages 886–893. IEEE.
Doucet, A., De Freitas, N., and Gordon, N. (2001).
Sequential Monte Carlo Methods in Practice.
Springer.
Everingham, M., Van Gool, L., Williams, C. K., Winn, J.,
and Zisserman, A. (2010). The pascal visual object
classes (VOC) challenge. IJCV, 88(2):303–338.
Isard, M. and Blake, A. (1998). Condensationconditional
density propagation for visual tracking. IJCV,
29(1):5–28.
Levin, A., Viola, P., and Freund, Y. (2003). Unsupervised
improvement of visual detectors using cotraining. In
CV, pages 626–633. IEEE.
Lim, J. J., Salakhutdinov, R., and Torralba, A.
(2011). Transfer learning by borrowing examples for
multiclass object detection. In NIPS.
Long, M., Wang, J., Ding, G., Sun, J., and Yu,
P. S. (2013). Transfer feature learning with joint
distribution adaptation. In ICCV, pages 2200–2207.
IEEE.
Mei, X. and Ling, H. (2011). Robust visual tracking and
vehicle classification via sparse representation. PAMI,
33(11):2259–2272.
Nair, V. and Clark, J. J. (2004). An unsupervised, online
learning framework for moving object detection. In
CVPR, volume 2, pages II–317. IEEE.
Pan, S. J., Tsang, I. W., Kwok, J. T., and Yang, Q. (2011).
Domain adaptation via transfer component analysis.
NN, 22(2):199–210.
Pan, S. J. and Yang, Q. (2010). A survey on transfer
learning. KDE, 22(10):1345–1359.
Pang, J., Huang, Q., Yan, S., Jiang, S., and Qin, L. (2011).
Transferring boosted detectors towards viewpoint and
scene adaptiveness. IP, 20(5):1388–1400.
Quanz, B., Huan, J., and Mishra, M. (2012). Knowledge
transfer with low-quality data: A feature extraction
issue. KDE, 24(10):1789–1802.
Rosenberg, C., Hebert, M., and Schneiderman, H. (2005).
Semi-supervised self-training of object detection
models. In WACV. IEEE Press.
Salakhutdinov, R., Tenenbaum, J., and Torralba, A. (2010).
One-shot learning with a hierarchical nonparametric
bayesian model. JMLR - UTL, 27:195–207.
Smal, I., Niessen, W., and Meijering, E. (2007). Advanced
particle filtering for multiple object tracking in
dynamic fluorescence microscopy images. In BIFNM,
pages 1048–1051. IEEE.
Tang, K., Ramanathan, V., Fei-Fei, L., and Koller, D.
(2012). Shifting weights: Adapting object detectors
from image to video. In ANIPS, pages 638–646.
Tommasi, T., Orabona, F., and Caputo, B. (2010). Safety
in numbers: Learning categories from few examples
with multi model knowledge transfer. In CVPR, pages
3081–3088. IEEE.
Viola, P. and Jones, M. (2001). Robust real-time object
detection. IJCV, 4:51–52.
Transductive Transfer Learning to Specialize a Generic Classifier Towards a Specific Scene
421