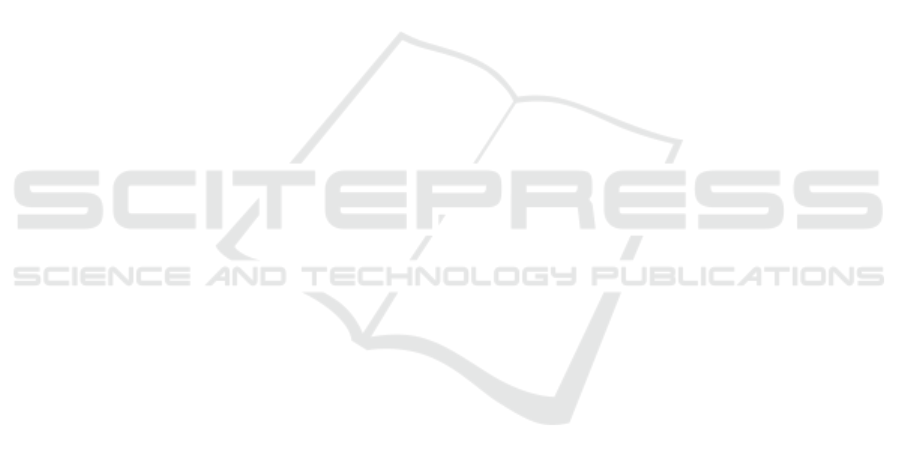
Dekel, S., Sochen, N., and Avidan, S. (2013). Incremental
Level Set Tracking. Springer.
Douglas, B. R., Charboneau, J. W., and Reading, C. C.
(2001). Ultrasound-guided intervention: Expand-
ing horizons. Radiologic Clinics of North America,
39(3):415 – 428.
Farneb
¨
ack, G. (2003). Two-frame motion estimation based
on polynomial expansion. In Image Analysis, pages
363–370. Springer.
Frey, B. J. and Dueck, D. (2007). Clustering by
passing messages between data points. science,
315(5814):972–976.
Gautama, T. and Van Hulle, M. M. (2002). A phase-based
approach to the estimation of the optical flow field us-
ing spatial filtering. Neural Networks, IEEE Transac-
tions on, 13(5):1127–1136.
Geman, S. and Geman, D. (1984). Stochastic relaxation,
gibbs distributions, and the bayesian restoration of
images. Pattern Analysis and Machine Intelligence,
IEEE Transactions on, (6):721–741.
Guerrero, J., Salcudean, S. E., Mcewen, J., Masri, B., Nico-
laou, S., et al. (2007). Real-time vessel segmenta-
tion and tracking for ultrasound imaging applications.
Medical Imaging, IEEE Transactions on, 26(8):1079–
1090.
Harris, E. J., Miller, N. R., Bamber, J. C., Symonds-Tayler,
J. R. N., and Evans, P. M. (2010). Speckle tracking
in a phantom and feature-based tracking in liver in the
presence of respiratory motion using 4d ultrasound.
Physics in medicine and biology, 55(12):3363.
Holzer, S., Ilic, S., Tan, D., Pollefeys, M., and Navab, N.
(2015). Efficient learning of linear predictors for tem-
plate tracking. International Journal of Computer Vi-
sion, 111(1):12–28.
Isard, M. and Blake, A. (1998). Condensationconditional
density propagation for visual tracking. International
journal of computer vision, 29(1):5–28.
Kalal, Z., Mikolajczyk, K., and Matas, J. (2012). Tracking-
learning-detection. Pattern Analysis and Machine In-
telligence, IEEE Transactions on, 34(7):1409–1422.
Keall, P. J., Mageras, G. S., Balter, J. M., Emery, R. S.,
Forster, K. M., Jiang, S. B., Kapatoes, J. M., Low,
D. A., Murphy, M. J., Murray, B. R., et al. (2006). The
management of respiratory motion in radiation oncol-
ogy report of aapm task group 76a). Medical physics,
33(10):3874–3900.
Kondo, S. (2014). Liver ultrasound tracking using long-
term and short-term template matching. Challenge on
Liver Ultrasound Tracking CLUST 2014, page 13.
K
¨
onig, L., Kipshagen, T., and R
¨
uhaak, J. (2014). A
non-linear image registration scheme for real-time
liver ultrasound tracking using normalized gradient
fields. Challenge on Liver Ultrasound Tracking
CLUST 2014, page 29.
Kovesi, P. (2003). Phase congruency detects corners and
edges. In The australian pattern recognition society
conference: DICTA 2003.
Lediju, M., Byram, B. C., Harris, E. J., Evans, P. M., Bam-
ber, J. C., et al. (2010). 3d liver tracking using a matrix
array: Implications for ultrasonic guidance of imrt.
In Ultrasonics Symposium (IUS), 2010 IEEE, pages
1628–1631. IEEE.
Liu, C. (2009). Beyond pixels: exploring new representa-
tions and applications for motion analysis. PhD the-
sis, Citeseer.
Lubinski, M., Emelianov, S. Y., O’Donnell, M., et al.
(1999). Speckle tracking methods for ultrasonic elas-
ticity imaging using short-time correlation. Ultra-
sonics, Ferroelectrics, and Frequency Control, IEEE
Transactions on, 46(1):82–96.
L
¨
ubke, D. and Grozea, C. (2014). High performance on-
line motion tracking in abdominal ultrasound imag-
ing. Challenge on Liver Ultrasound Tracking CLUST
2014.
Matthews, I., Ishikawa, T., and Baker, S. (2004). The tem-
plate update problem. IEEE Transactions on Pattern
Analysis & Machine Intelligence, (6):810–815.
Mei, X., Zhou, S. K., and Porikli, F. (2007). Probabilistic
visual tracking via robust template matching and in-
cremental subspace update. In Multimedia and Expo,
2007 IEEE International Conference on, pages 1818–
1821. IEEE.
Mercier, L., Langø, T., Lindseth, F., and Collins, L. D.
(2005). A review of calibration techniques for free-
hand 3-d ultrasound systems. Ultrasound in medicine
& biology, 31(2):143–165.
O’Shea, T., Bamber, J., and Harris, E. (2014). Liver fea-
ture motion estimation in long high frame rate 2d ul-
trasound sequences. Challenge on Liver Ultrasound
Tracking CLUST 2014.
Rattani, A., Freni, B., Marcialis, G. L., and Roli, F. (2009).
Template update methods in adaptive biometric sys-
tems: a critical review. In Advances in Biometrics,
pages 847–856. Springer.
Rothl
¨
ubbers, S., Schwaab, J., Jenne, J., and G
¨
unther, M.
(2014). Miccai clust 2014: Bayesian real-time liver
feature ultrasound tracking. Challenge on Liver Ul-
trasound Tracking. MICCAI, pages 45–52.
Shirato, H., Shimizu, S., Kitamura, K., and Onimaru, R.
(2007). Organ motion in image-guided radiotherapy:
lessons from real-time tumor-tracking radiotherapy.
International Journal of Clinical Oncology, 12(1):8–
16.
Siegel, R. L., Miller, K. D., and Jemal, A. (2015). Cancer
statistics, 2015. CA: A Cancer Journal for Clinicians,
65(1):5–29.
Somphone, O., Allaire, S., Mory, B., and Dufour, C. (2014).
Live feature tracking in ultrasound liver sequences
with sparse demons. Challenge on Liver Ultrasound
Tracking CLUST 2014, page 53.
Thirion, J.-P. (1998). Image matching as a diffusion pro-
cess: an analogy with maxwell’s demons. Medical
image analysis, 2(3):243–260.
Tomasi, M., Barranco, F., Vanegas, M., D
´
ıaz, J., and Ros,
E. (2010). Fine grain pipeline architecture for high
performance phase-based optical flow computation.
Journal of Systems Architecture, 56(11):577–587.
Wang, Z., Bovik, A. C., Sheikh, H. R., and Simoncelli, E. P.
(2004). Image quality assessment: from error visibil-
VISAPP 2016 - International Conference on Computer Vision Theory and Applications
532