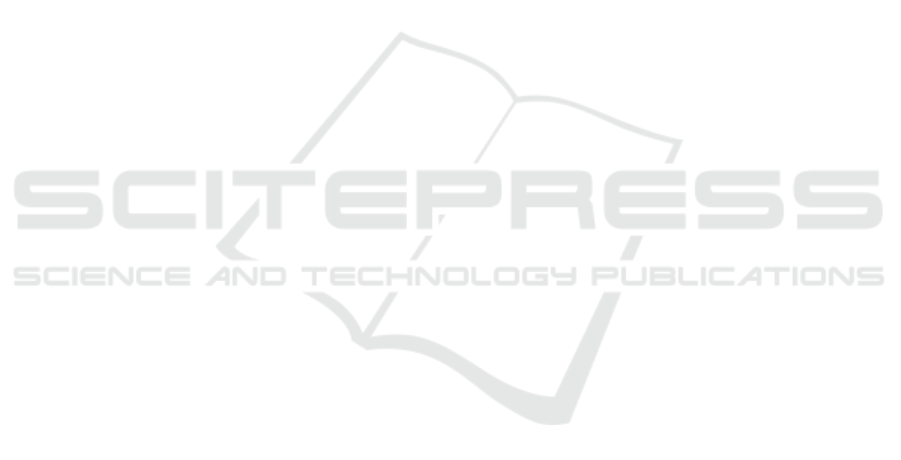
MATLAB. Human gait balance was analyzed apply-
ing static (GCOM) and dynamic (ZMP) stabilities.
Human ZMP and GCoM trajectories were calcu-
lated by two methods, which consider human body
as a simplified approximation with the models of a
single mass point and multiple mass points. Our cal-
culations demonstrated close localization of analyzed
GCoM and ZMP trajectories to the human’s footprints
and the corresponding support polygons. It means
that the human walking had static and dynamic sta-
bility, proving that the human gait was properly bal-
anced. The comparison of total ZMP errors for hu-
man body models as a single and multiple mass points
demonstrated that the later method is significantly
more accurate for the limited MoCap walking zone of
2 m length (the maximal ZMP error was 3.5 cm along
the walking direction and 0.7 cm in lateral direction)
than the former one, and thus should be preferred for
human gait estimation. Quite significant ZMP trajec-
tory deviations in the vicinity of footprints’ positions
arise from the limitations of MoCap system measure-
ment accuracy.
Finally, we use MoCap system and analyze human
locomotion to identify key features of human walk-
ing, collecting statistically significant data to create
an adequate human gait mathematical model, which
could be adapted to Russian AR-601M robot simu-
lation model, yielding its statically and dynamically
stable and more natural locomotion.
ACKNOWLEDGEMENTS
This work was supported by Russian Ministry of
Education and Science, and our industrial partner
Android Technics under Scientific and Technolog-
ical Research and Development Program of Rus-
sian Federation for 2014-2020 years (research grant
RFMEFI60914X0004). Special thanks to iPi Soft
company for providing temporary access to iPi Soft
Mocap Studio.
REFERENCES
Asano, F. and Luo, Z.-W. (2008). Energy-efficient and
high-speed dynamic biped locomotion based on prin-
ciple of parametric excitation. IEEE Transactions on
Robotics, 24(6):1289–1301.
Chalodhorn, R., Grimes, D. B., Grochow, K., and Rao, R.
P. N. (2007). Learning to walk through imitation. In
Proc. of 20th Int. Joint Conference on Artifical Intel-
ligence, IJCAI’07, pages 2084–2090, San Francisco,
CA, USA. Morgan Kaufmann Publishers Inc.
Dasgupta, A. and Nakamura, Y. (1999). Making feasible
walking motion of humanoid robots from human mo-
tion capture data. In IEEE ICRA, volume 2, pages
1044–1049.
Field, M., Stirling, D., Naghdy, F., and Pan, Z. (2009). Mo-
tion capture in robotics review. In IEEE Int. Conf. on
Control and Automation, pages 1697–1702.
Gabbasov, B., Danilov, I., Afanasyev, I., and Magid, E.
(2015). Toward a human-like biped robot gait: Biome-
chanical analysis of human locomotion recorded by
kinect-based motion capture system. In Proc. of 10th
Int. Symposium on Mechatronics and its Applications.
Goswami, A. (1999). Foot rotation indicator (fri) point: A
new gait planning tool to evaluate postural stability of
biped robots. In IEEE ICRA, pages 47–52.
Ha, T. and Choi, C.-H. (2007). An effective trajectory gen-
eration method for bipedal walking. Robotics and Au-
tonomous Systems, 55(10):795–810.
Haley, J. et al. (1988). Anthropometry and mass distribu-
tion for human analogues. In Military Male Aviators,
Volume 1, Aerospace Medical Research Lab., Wright-
Patterson AFB Ohio USA, Tech. Rep, pages 34–38.
Kajita, S., Kanehiro, F., Kaneko, K., Fujiwara, K., Harada,
K., Yokoi, K., and Hirukawa, H. (2003). Biped walk-
ing pattern generation by using preview control of
zero-moment point. In IEEE ICRA, volume 2, pages
1620–1626.
Khusainov, R., Afanasyev, I., and Magid, E. (2016). An-
thropomorphic robot modelling with virtual height in-
verted pendulum approach in Simulink: step length
and robot height influence on walking stability. In Int.
Conf. on Artificial ALife and Robotics (in press).
Kim, H., Lee, S., Lee, D., Choi, S., Ju, J., and Myung, H.
(2015). Real-time human pose estimation and gesture
recognition from depth images using superpixels and
svm classifier. Sensors, 15(6):12410–12427.
Larsen, J. C. and Stoy, K. (2011). Energy efficiency of robot
locomotion increases proportional to weight. Proce-
dia Computer Science, Proc. 2nd European Future
Technologies Conference and Exhibition, 7:228 – 230.
Mrozowski, J., Awrejcewicz, J., and Bamberski, P. (2007).
Analysis of stability of the human gait. Journal of
theoretical and applied mechanics, 45:91–98.
Naksuk, N., Lee, C., and Rietdyk, S. (2005). Whole-body
human-to-humanoid motion transfer. In 5th IEEE-
RAS Int. Conf. on Humanoid Robots, pages 104–109.
Sinnet, R. W., Powell, M. J., Jiang, S., and Ames, A. D.
(2011). Compass gait revisited: A human data per-
spective with extensions to three dimensions. In Proc.
of 50th IEEE Conf. on Decision and Control, and Eu-
ropean Control Conf. (CDC-ECC), pages 682–689.
Vukobratovic, M. and Borovac, B. (2004). Zero-moment
point thirty five years of its life. International Journal
of Humanoid Robotics, 1(1):157–173.
Vukobratovic, M. and Juricic, D. (1969). Contribution to
the synthesis of biped gait. In IEEE Transactions on
Biomedical Engineering, volume 16(1), pages 1–6.
Wong, C., Zhang, Z.-Q., Lo, B., and Yang, G.-Z. (2015).
Wearable sensing for solid biomechanics: A review.
Sensors Journal, IEEE, 15(5):2747–2760.
VISAPP 2016 - International Conference on Computer Vision Theory and Applications
168