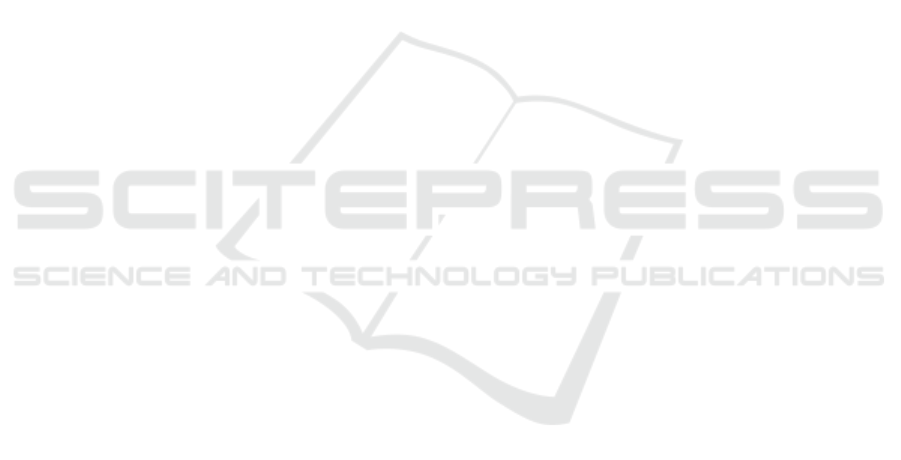
applied to other segmentation algorithms to improve
the tracking phase in the same way.
In particular, the proposed global patch reliability
measure, considering a diverse range of features, has
shown one of the many possible ways of integrating
segmentation phase data to object modelling. In the
present work, no a priori knowledge has been consid-
ered about the objects to be tracked. The integration
of the data from the segmentation phase with more
complex object models can also improve the tracking
phase, by better determining the objects of interest for
a context or application. At the same time, these relia-
bility measures can help these object models to better
determine their parameters, subject to noisy measure-
ments.
The preliminary evaluation obtained promising re-
sults both in robust tracking and quick processing.
Nevertheless, extensive testing is required for fully
validating the approach.
This work can be extended in several ways: the
approach can be tested for different types of detectors
of interest points and local feature detectors. Also,
the algorithm can be tested for different background
subtraction approaches. Also, an extensive parameter
sensitivity evaluation is still needed. As local features
are utilised, this approach could be naturally extended
to deal with dynamic occlusion situations.
ACKNOWLEDGEMENTS
This research has been supported, in part, by Fonde-
cyt Project 11121383, Chile.
REFERENCES
Adam, A., Rivlin, E., and Shimshoni, I. (2006). Ro-
bust fragments-based tracking using the integral his-
togram. In Computer Vision and Pattern Recogni-
tion, 2006 IEEE Computer Society Conference on,
volume 1, pages 798–805.
Alahi, A., Ortiz, R., and Vandergheynst, P. (2012). Freak:
Fast retina keypoint. In Procedings of the IEEE Con-
ference on Computer Vision and Pattern Recognition
(CVPR 2012), pages 510–517.
Bhattacharyya, A. (1943). On a measure of divergence be-
tween two statistical populations defined by probabil-
ity distributions. Bulletin of the Calcutta Mathemati-
cal Society, 35:99–110.
Breitenstein, M., Reichlin, F., Leibe, B., Koller-Meier,
E., and Van Gool, L. (2009). Robust tracking-by-
detection using a detector confidence particle filter. In
Computer Vision, 2009 IEEE 12th International Con-
ference on, pages 1515–1522.
Correia, P. L. and Pereira, F. (2003). Objective evaluation of
video segmentation quality. Image Processing, IEEE
Transactions on, 12(2):186–200.
Erdem, C¸ . E., Sankur, B., et al. (2004). Performance mea-
sures for video object segmentation and tracking. Im-
age Processing, IEEE Transactions on, 13(7):937–
951.
Kalal, Z., Matas, J., and Mikolajczyk, K. (2011). Track-
ing learning detection. IEEE Transactions on Pattern
Analysis and Machine Intelligence, 34(7):1409–1422.
Lee, S. and Horio, K. (2013). Human tracking using parti-
cle filter with reliable appearance model. In SICE An-
nual Conference (SICE), 2013 Proceedings of, pages
1418–1424.
McIvor, A. (2000). Background subtraction techniques. In
Proceedings of the Conference on Image and Vision
Computing (IVCNZ 2000), pages 147–153, Hamilton,
New Zealand.
Rosten, E. and Drummond, T. (2006). Machine learning
for high-speed corner detection. In Proceedings of
the IEEE European Conference on Computer Vision
(ECCV’06), volume 1, pages 430–443.
Sun, L. and Liu, G. (2011). Visual object tracking based
on combination of local description and global repre-
sentation. Circuits and Systems for Video Technology,
IEEE Transactions on, 21(4):408–420.
Toyama, K., Krumm, J., Brumitt, B., and Meyers, B.
(1999). Wallflower: principles and practice of back-
ground maintenance. In Proceedings of the Interna-
tional Conference on Computer Vision (ICCV 1999),
pages 255–261. doi:10.1109/ICCV.1999.791228.
Troya-Galvis, A., Gancarski, P., Passat, N., and Berti-
Equille, L. (2015). Unsupervised quantification of
under- and over-segmentation for object-based remote
sensing image analysis. Selected Topics in Applied
Earth Observations and Remote Sensing, IEEE Jour-
nal of, 8(5):1936–1945.
Wang, L., Yan, H., yu Wu, H., and Pan, C. (2013).
Forward-backward mean-shift for visual tracking
with local-background-weighted histogram. Intelli-
gent Transportation Systems, IEEE Transactions on,
14(3):1480–1489.
Yang, F., Lu, H., and Yang, M. (2014). Robust superpixel
tracking. IEEE Transactions on Image Processing,
23(4):1639–1651.
Zuniga, M. D., Bremond, F., and Thonnat, M. (2011).
Real-time reliability measure driven multi-hypothesis
tracking using 2d and 3d features. EURASIP Jour-
nal on Advances in Signal Processing, 2011(1):142.
doi:10.1186/1687-6180-2011-142.
Robust Real-time Tracking Guided by Reliable Local Features
69