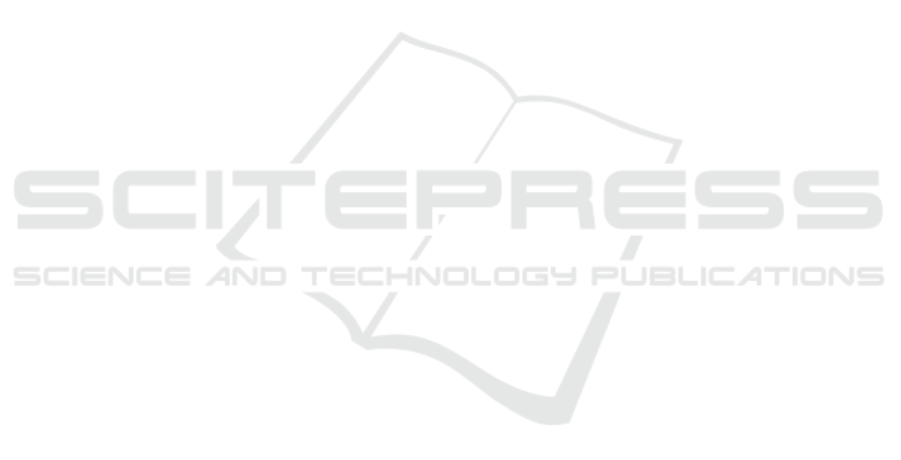
extended to multiple actions by computing several po-
lar regions of interest and then characterizing each of
them individually.
ACKNOWLEDGEMENTS
This research is funded by the RTRA Digiteo project
MAPOCA.
REFERENCES
Cao, T., Wu, X., Guo, J., Yu, S., and Xu, Y. (2009). Abnor-
mal crowd motion analysis. In Int. Conf. on Robotics
and Biomimetics, pages 1709–1714.
Cao, X., Zhang, H., Deng, C., Liu, Q., and Liu, H. (2014).
Action recognition using 3d daisy descriptor. Mach.
Vision Appl., 25(1):159–171.
Chang, C.-C. and Lin, C.-J. (2011). Libsvm: A library
for support vector machines. ACM Trans. Intell. Syst.
Technol., 2(3):27:1–27:27.
Chaudhry, R., Ravichandran, A., Hager, G., and Vidal, R.
(2009). Histograms of oriented optical flow and binet-
cauchy kernels on nonlinear dynamical systems for
the recognition of human actions. In Computer Vision
and Pattern Recognition, 2009. CVPR 2009. IEEE
Conference on, pages 1932–1939.
Efros, A., Berg, A. C., Mori, G., and Malik, J. (2003). Rec-
ognizing Action at a Distance. In Int. Conf. on Com-
puter Vision, Washington, DC, USA.
Garrigues, M. and Manzanera, A. (2012). Real time semi-
dense point tracking. In Campilho, A. and Kamel,
M., editors, Int. Conf. on Image Analysis and Recog-
nition (ICIAR 2012), volume 7324 of Lecture Notes in
Computer Science, pages 245–252, Aveiro, Portugal.
Springer.
Ikizler, N., Cinbis, R., and Duygulu, P. (2008). Human
action recognition with line and flow histograms. In
Pattern Recognition, 2008. ICPR 2008. 19th Interna-
tional Conference on, pages 1–4.
Jain, M., Jegou, H., and Bouthemy, P. (2013). Better ex-
ploiting motion for better action recognition. In Pro-
ceedings of the 2013 IEEE Conference on Computer
Vision and Pattern Recognition, CVPR ’13, pages
2555–2562, Washington, DC, USA. IEEE Computer
Society.
Kantorov, V. and Laptev, I. (2014). Efficient feature ex-
traction, encoding and classification for action recog-
nition.
Ke, Y., Sukthankar, R., and Hebert, M. (2005). Efficient
visual event detection using volumetric features. In
Computer Vision, 2005. ICCV 2005. Tenth IEEE In-
ternational Conference on, volume 1, pages 166–173
Vol. 1.
Laptev, I., Marszałek, M., Schmid, C., and Rozenfeld,
B. (2008). Learning realistic human actions from
movies. In Conference on Computer Vision & Pattern
Recognition.
Liu, J., Luo, J., and Shah, M. (2009). Recognizing realistic
actions from videos ”in the wild”. IEEE International
Conference on Computer Vision and Pattern Recogni-
tion.
Ryoo, M. S. and Aggarwal, J. K. (2010). UT-Interaction
Dataset, ICPR contest on Semantic Description of Hu-
man Activities (SDHA).
Schuldt, C., Laptev, I., and Caputo, B. (2004). Recognizing
human actions: A local svm approach. In Proceedings
of the Pattern Recognition, 17th International Confer-
ence on (ICPR’04) Volume 3 - Volume 03, ICPR ’04,
pages 32–36, Washington, DC, USA. IEEE Computer
Society.
Scovanner, P., Ali, S., and Shah, M. (2007). A 3-
dimensional sift descriptor and its application to ac-
tion recognition. pages 357–360.
Tabia, H., Gouiffes, M., and Lacassagne, L. (2012). Mo-
tion histogram quantification for human action recog-
nition. In Pattern Recognition (ICPR), 2012 21st In-
ternational Conference on, pages 2404–2407. IEEE.
Wang, H., Klaser, A., Schmid, C., and Liu, C.-L. (2011).
Action recognition by dense trajectories. In Proceed-
ings of the 2011 IEEE Conference on Computer Vi-
sion and Pattern Recognition, CVPR ’11, pages 3169–
3176, Washington, DC, USA. IEEE Computer Soci-
ety.
Wang, H. and Schmid, C. (2013). Action Recognition with
Improved Trajectories. In ICCV 2013 - IEEE Interna-
tional Conference on Computer Vision, pages 3551–
3558, Sydney, Australia. IEEE.
Wang, H., Ullah, M. M., Kliser, A., Laptev, I., and Schmid,
C. (2009). Evaluation of local spatio-temporal fea-
tures for action recognition. In BMVC. British Ma-
chine Vision Association.
Weinland, D., Ronfard, R., and Boyer, E. (2011). A sur-
vey of vision-based methods for action representation,
segmentation and recognition. Computer Vision and
Image Understanding, 115(2):224–241.
Willems, G., Tuytelaars, T., and Gool, L. (2008). An effi-
cient dense and scale-invariant spatio-temporal inter-
est point detector. In Proceedings of the 10th Euro-
pean Conference on Computer Vision: Part II, ECCV
’08, pages 650–663, Berlin, Heidelberg. Springer-
Verlag.
Yu, T.-H., Kim, T.-K., and Cipolla, R. (2010). Real-time ac-
tion recognition by spatiotemporal semantic and struc-
tural forest. In Proceedings of the British Machine
Vision Conference, pages 52.1–52.12. BMVA Press.
doi:10.5244/C.24.52.
Zhang, Z., Hu, Y., Chan, S., and Chia, L.-T. (2008). Mo-
tion context: A new representation for human ac-
tion recognition. Computer Vision–ECCV 2008, pages
817–829.
VISAPP 2016 - International Conference on Computer Vision Theory and Applications
448