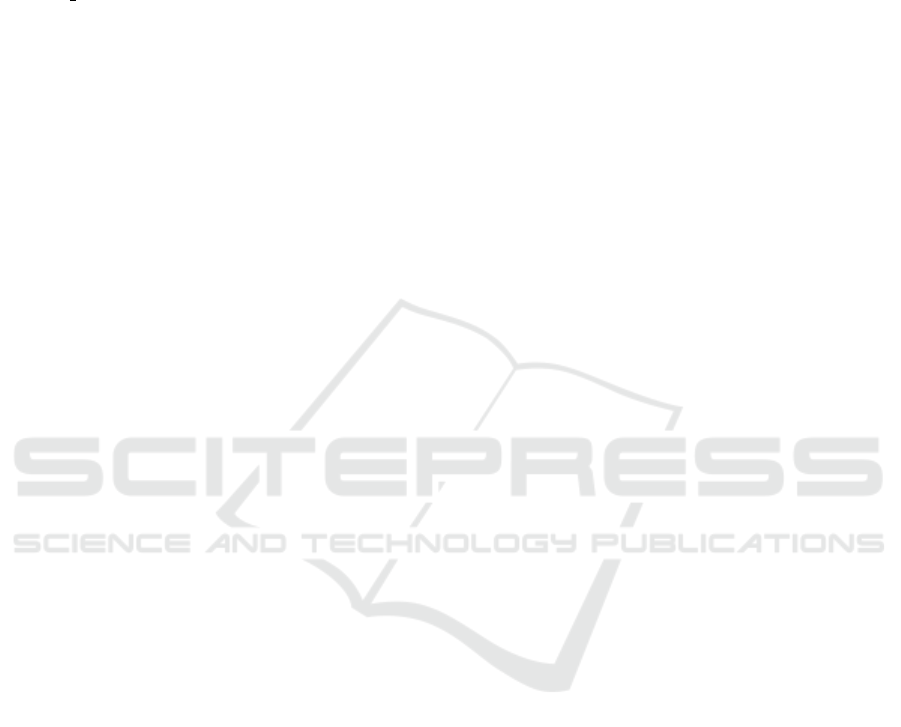
dent progress modeler. First, the computational re-
quirements are reduced because the entire student’s
history is not necessary to compute the updated la-
tent features. Then, the algorithm remains domain in-
dependent because the tagged skills of the tasks are
not necessary to deliver a score prediction. Finally,
KSEMF SD reduced the prediction error and is less
sensitive to the lack of that. In future work we believe
to further be able to reduce the error by developing a
better initialization of the students’ latent features.
ACKNOWLEDGEMENT
This research has been co-funded by the Sev-
enth Framework Programme of the European Com-
mission, through project iTalk2Learn (#318051).
www.iTalk2Learn.eu.
REFERENCES
Cichocki, A., Zdunek, R., Phan, A. H., and Amari, S.-i.
(2009). Nonnegative matrix and tensor factorizations:
applications to exploratory multi-way data analysis
and blind source separation. Wiley. com.
Corbett, A. and Anderson, J. (1994). Knowledge tracing:
Modeling the acquisition of procedural knowledge.
UMAI.
D Baker, R. S., Corbett, A. T., and Aleven, V. (2008). More
accurate student modeling through contextual estima-
tion of slip and guess probabilities in bayesian knowl-
edge tracing. In ITS, pages 406–415. Springer.
Janning, R., Schatten, C., and Lars, S.-T. (2014a). Fea-
ture analysis for affect recognition supporting task se-
quencing. In ECTEL.
Janning, R., Schatten, C., and Schmidt-Thieme, L. (2014b).
Multimodal affect recognition for adaptive intelligent
tutoring systems. In FFMI EDM.
Kalman, R. E. (1960). A new approach to linear filtering
and prediction problems. Journal of Fluids Engineer-
ing, 82(1):35–45.
Koren, Y., Bell, R., and Volinsky, C. (2009). Matrix factor-
ization techniques for recommender systems. Com-
puter, 42(8):30–37.
Li, B., Zhu, X., Li, R., Zhang, C., Xue, X., and Wu,
X. (2011). Cross-domain collaborative filtering over
time. In Proceedings of the Twenty-Second inter-
national joint conference on Artificial Intelligence-
Volume Volume Three, pages 2293–2298. AAAI Press.
Manouselis, N., Drachsler, H., Vuorikari, R., Hummel, H.,
and Koper, R. (2011). Recommender systems in tech-
nology enhanced learning. In Recommender systems
handbook, pages 387–415. Springer.
Nielsen, J. (1994). Usability engineering. Elsevier.
Pardos, Z. A. and Heffernan, N. T. (2010). Modeling indi-
vidualization in a bayesian networks implementation
of knowledge tracing. In UMAP. Springer.
Pardos, Z. A. and Heffernan, N. T. (2011). Kt-idem: intro-
ducing item difficulty to the knowledge tracing model.
In UMAP, pages 243–254. Springer.
Pavlik, P., Cen, H., and Koedinger, K. (2009). Performance
factors analysis-a new alternative to knowledge trac-
ing. In AIED.
Pil
´
aszy, I. and Tikk, D. (2009). Recommending new
movies: Even a few ratings are more valuable than
metadata. In RecSys.
Rendle, S. and Schmidt-Thieme, L. (2008). Online-
updating regularized kernel matrix factorization mod-
els for large-scale recommender systems. In Proceed-
ings of the 2008 ACM conference on Recommender
systems, pages 251–258. ACM.
Schatten, C., Janning, R., and Schmidt-Thieme, L. (2014a).
Vygotsky based sequencing without domain informa-
tion: A matrix factorization approach. In Computer
Supported Education, pages 35–51. Springer.
Schatten, C., Janning, R., and Schmidt-Thieme, L. (2015).
Integration and evaluation of a machine learning se-
quencer in large commercial its. In AAAI2015.
Springer.
Schatten, C., Mavrikis, M., Janning, R., and Schmidt-
Thieme, L. (2014b). Matrix factorization feasibility
for sequencing and adaptive support in its. In EDM.
Schatten, C. and Schmidt-Thieme, L. (2014). Adaptive
content sequencing without domain information. In
CSEDU.
Schatten, C., Wistuba, M., Schmidt-Thieme, L., and
Gutirrez-Santos, S. (2014c). Minimal invasive inte-
gration of learning analytics services in its. In ICALT.
Schilling, N., Wistuba, M., Drumond, L., and Schmidt-
Thieme, L. (2015). Joint model choice and hyperpa-
rameter optimization with factorized multilayer per-
ceptrons. In Tools with Artificial Intelligence (ICTAI),
2015 IEEE 27th International Conference on, pages
72–79. IEEE.
Thai-Nghe, N., Drumond, L., Horvath, T., Krohn-
Grimberghe, A., Nanopoulos, A., and Schmidt-
Thieme, L. (2011). Factorization techniques for pre-
dicting student performance. Educational Recom-
mender Systems and Technologies: Practices and
Challenges. IGI Global.
Thai-Nghe, N., Drumond, L., Horvath, T., and Schmidt-
Thieme, L. (2012). Using factorization machines for
student modeling. In UMAP Workshops.
Thai-Nghe, N., Drumond, L., Krohn-Grimberghe, A., and
Schmidt-Thieme, L. (2010). Recommender system
for predicting student performance. Procedia Com-
puter Science, 1(2):2811–2819.
Voss, L., Schatten, C., and Schmidt-Thieme, L. (2015). A
transfer learning approach for applying matrix factor-
ization to small its datasets. In EDM2015.
Vygotsky, L. L. S. (1978). Mind in society: The develop-
ment of higher psychological processes. HUP.
Wang, Y. and Heffernan, N. T. (2012). The student skill
model. In ITS2012.
Student Progress Modeling with Skills Deficiency Aware Kalman Filters
41