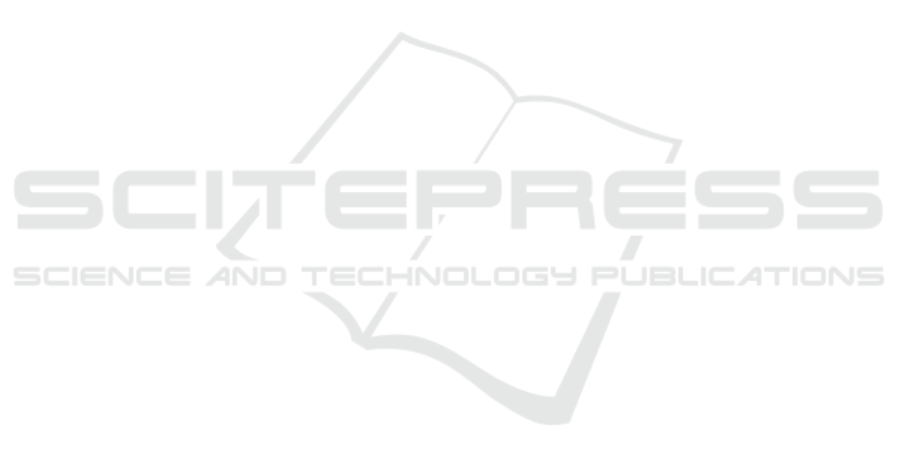
economic model. The simulation investigation fo-
cuses on technological and operational impacts, while
the economic model examines social and economic
aspects in the long-term. The proposed process en-
ables researchers to investigate hybrid networks in de-
tail, with which one can provide concrete recommen-
dations for stakeholders in both technological (opera-
tional) and economic (strategic) aspects.
2 STATE OF THE ART
With the unbundling of the energy supply chain,
technological advancements of renewables, support
mechanisms to promote their installation, increasing
environmental awareness and more active participa-
tion of customers, the amount of distributed (small-
scale) generation plants and de-centralized feed-in of
electricity has considerably increased in recent years.
Thus, energy distribution system operators (DSO)
have to cope with bidirectional load flows in their net-
works and both, DSOs and energy supply companies,
have to deal with decreasing turnover. Furthermore,
fluctuating energy production from renewable energy
sources (RES) - from large-scale to household level -
is de-coupled from energy demand, which is causing
a lack of storage in the electricity network (Trebolle
et al., 2010).
In general, this issue can be partially tackled by
shedding renewables during hours of high produc-
tion, increasing transmission capacity of the electric-
ity network, installing additional capacities of energy
storages and/or increasing the flexibility of demand.
However, the challenge will probably not be solved
by one of these options alone and the storage and flex-
ibility potentials on the electricity domain are limited.
Considering other energy domains and networks
(gas, district heating) as well can significantly in-
crease the storage capacity and demand flexibility po-
tentials. Conversely, these domains can benefit from
a closer interaction with the electricity domain too.
Converting electric energy when production from re-
newables is high and electricity demand is low, e.g.,
can reduce the usage of fossil fuels for heat pro-
duction. Though these synergies are becoming in-
creasingly apparent, the full potential of cooperation
among different energy domains is not yet fully ex-
ploited. There are several reasons for this: For one
thing, different energy domains are, in fact, compet-
ing for the customers energy demand. Space heat-
ing, e.g., can be provided by district heating, or by a
gas or electricity network using e.g. heat pumps or
boilers. Thus, different market participants (DSOs,
supply companies) operating on different energy do-
mains are rather interested in maximizing their own
turnover and profit than in finding cooperative strate-
gies to increase total efficiency. Furthermore, there
are some structural and regulatory issues complicat-
ing a connection and cooperation between different
energy networks. It is possible, e.g., that using cheap
excess electricity from RES production for heating is
not economical compared to using other fuels due to
electricity network charges, even though an increased
electricity demand could support network operation.
The topic of hybrid energy grid is getting more
interests recently. Behavior of individual compo-
nents (Keirstead et al., 2012), optimizing local con-
trols (Bakken et al., 2006)(Arnold et al., 2009), or
infrastructural planning (Hinterberger and Kleimaier,
2013) have been investigated before. Compared to
previous work, the proposed process of this paper is
more holistic and aims to deliver the whole picture,
and focuses on the evolutionary path of the existing
energy networks. One special focus here is exploiting
the synergies among different energy domains and,
hence, increasing the flexibility of energy networks
and facilitating the integration of RES. The approach
emphasizes a multi-agent perspective by taking into
account the individual objectives of different market
participants and aiming to develop cooperative strate-
gies among competitors resulting in win-win situa-
tions. Moreover, this process aims to identify barriers
in todays regulations and go beyond current market
rules to examine possible future hybrid control strate-
gies and business models.
3 HYBRID-GRID
INVESTIGATION: SETUPS AND
REQUIREMENTS
3.1 Identifying Hybrid Setups from the
Two European Cities
The investigation process is first initialized by spot-
ting possible hybrid chances from two actual Euro-
pean sites. Our target sites are the city of Skellete
˚
a,
Sweden, and two districts of Ulm, Germany.
Skellefte
˚
a is a city in mid-northern Sweden, in a
subarctic climate region. Population of the city is over
32,000, and served by district heating (DH) grid with
around 4200 heating substations. For a typical year,
the DH grid provides about 343,000 MWh of heat to
the city. Base heat load is being served by a CHP,
which uses bio-mass fuel to generate heat and power.
Districts of Einsingen and Hittistetten are located in
the suburb of Ulm, Germany. They are small residen-
SMARTGREENS 2016 - 5th International Conference on Smart Cities and Green ICT Systems
264