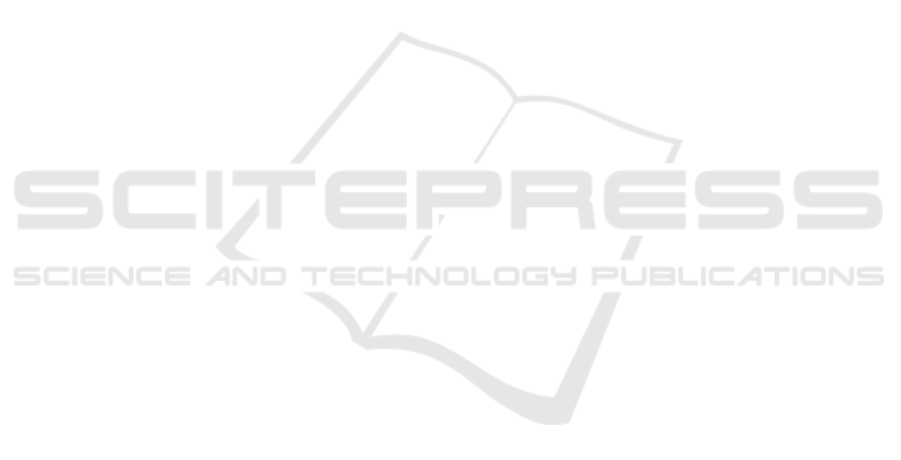
D. A., Unverzagt, F. W., and Petersen, R. C. (2011).
Outcomes of mild cognitive impairment depend on
definition: a population study. Archives of neurology,
68(6):761–767.
Gauthier, S., Reisberg, B., Zaudig, M., Petersen, R. C.,
Ritchie, K., Broich, K., Belleville, S., Brodaty, H.,
Bennett, D., Chertkow, H., Cummings, J. L., de Leon,
M., Feldman, H., Ganguli, M., Hampel, H., Schel-
tens, P., Tierney, M. C., Whitehouse, P., and Winblad,
B. (2006). Mild cognitive impairment. The Lancet,
367(9518):1262 – 1270.
Hall, M., Frank, E., Holmes, G., Pfahringer, B., Reutemann,
P., and Witten, I. H. (2009). The weka data min-
ing software: An update. SIGKDD Explor. Newsl.,
11(1):10–18.
Haller, S., Missonnier, P., Herrmann, F. R., Rodriguez, C.,
Deiber, M.-P., Nguyen, D., Gold, G., Lovblad, K.-O.,
and Giannakopoulos, P. (2013). Individual classifica-
tion of mild cognitive impairment subtypes by support
vector machine analysis of white matter DTI. AJNR.
American journal of neuroradiology, 34(2):283–91.
Hedden, T. and Gabrieli, J. D. E. (2004). Insights into the
ageing mind: a view from cognitive neuroscience. Nat
Rev Neurosci, 5(2):87–96.
Hindmarch, I., Lehfeld, H., de Jongh, P., and Erzigkeit, H.
(1998). The bayer activities of daily living scale (b-
adl). Dementia and Geriatric Cognitive Disorders,
9(suppl 2)(Suppl. 2):20–26.
Hinrichs, C., Singh, V., Xu, G., and Johnson, S. C. (2011).
Predictive markers for AD in a multi-modality frame-
work: An analysis of MCI progression in the ADNI
population. NeuroImage, 55(2):574–589.
Kochan, N. A., Slavin, M. J., Brodaty, H., Crawford, J. D.,
Trollor, J. N., Draper, B., and Sachdev, P. S. (2010).
Effect of Different Impairment Criteria on Prevalence
of “Objective” Mild Cognitive Im-
pairment in a Community Sample. The American
Journal of Geriatric Psychiatry, 18(8):711–722.
Kotsiantis, S. B. (2007). Supervised machine learning:
A review of classification techniques. informatica
31:249268.
Lemm, S., Blankertz, B., Dickhaus, T., and Muller, K.-R.
(2011). Introduction to machine learning for brain
imaging. NeuroImage, 56(2):387 – 399.
Lemos, L., Silva, D., Guerreiro, M., Santana, I., de Men-
dona, A., Toms, P., and Madeira, S. C. (2012). Dis-
criminating alzheimers disease from mild cognitive
impairment using neuropsychological data. KDD
2012.
Liaw, A. and Wiener, M. (2002). Classification and Regres-
sion by randomForest. R News, 2(3):18–22.
Maglogiannis, I. (2007). Emerging Artificial Intelligence
Applications in Computer Engineering: Real World
AI Systems with Applications in EHealth, HCI, Infor-
mation Retrieval and Pervasive Technologies. Fron-
tiers in artificial intelligence and applications. IOS
Press.
Mitchell, A. J. and Shiri-Feshki, M. (2009). Rate of pro-
gression of mild cognitive impairment to dementia
meta-analysis of 41 robust inception cohort studies.
Acta Psychiatrica Scandinavica, 119(4):252–265.
Murthy, S. (1998). Automatic construction of decision trees
from data: A multi-disciplinary survey. Data Mining
and Knowledge Discovery, 2(4):345–389.
Petersen, R. C., Knopman, D. S., Boeve, B. F., Geda, Y. E.,
Ivnik, R. J., Smith, G. E., Roberts, R. O., and Jack,
C. R. (2009). Mild Cognitive Impairment: Ten Years
Later. Archives of neurology, 66(12):1447–1455.
Raamana, P. R., Wen, W., Kochan, N. a., Brodaty, H.,
Sachdev, P. S., Wang, L., and Beg, M. F. (2014). The
sub-classification of amnestic mild cognitive impair-
ment using MRI-based cortical thickness measures.
Frontiers in Neurology, pages 1–10.
Reddy, P., Kochan, N., Brodaty, H., Sachdev, P., Wang, L.,
Beg, M. F., and Wen, W. (2013). Novel ThickNet fea-
tures for the discrimination of amnestic MCI subtypes.
NeuroImage Clinical, 6:284–295.
Reppermund, S., Zhuang, L., Wen, W., Slavin, M. J.,
Trollor, J. N., Brodaty, H., and Sachdev, P. S.
(2014). White matter integrity and late-life depression
in community-dwelling individuals: diffusion tensor
imaging study using tract-based spatial statistics. The
British Journal of Psychiatry, 205:315–320.
Sachdev, P. S., Brodaty, H., Reppermund, S., Kochan,
N. A., Trollor, J. N., Draper, B., Slavin, M. J., Craw-
ford, J., Kang, K., Broe, G. A., Mather, K. A., and
Lux, O. (2010). The sydney memory and ageing study
(mas): methodology and baseline medical and neu-
ropsychiatric characteristics of an elderly epidemio-
logical non-demented cohort of australians aged 7090
years. International Psychogeriatrics, 22:1248–1264.
Sachdev, P. S., Lipnicki, D. M., Crawford, J., Reppermund,
S., Kochan, N. a., Trollor, J. N., Wen, W., Draper,
B., Slavin, M. J., Kang, K., Lux, O., Mather, K. a.,
Brodaty, H., and Team, A. S. (2013a). Factors Pre-
dicting Reversion from Mild Cognitive Impairment to
Normal Cognitive Functioning: A Population-Based
Study. PLoS ONE, 8(3):1–10.
Sachdev, P. S., Zhuang, L., Braidy, N., and Wen, W.
(2013b). Is Alzheimer’s a disease of the white mat-
ter? Curr Opin Psychiatry, 26(3):244–251.
Thillainadesan, S., Wen, W., Zhuang, L., Crawford, J.,
Kochan, N., Reppermund, S., Slavin, M., Trollor, J.,
Brodaty, H., and Sachdev, P. (2012). Changes in
mild cognitive impairment and its subtypes as seen on
diffusion tensor imaging. International Psychogeri-
atrics, 24:1483–1493.
Winblad, B., Palmer, K., Kivipelto, M., Jelic, V.,
Fratiglioni, L., Wahlund, L.-O., Nordberg, A., Bck-
man, L., Albert, M., Almkvist, O., Arai, H., Basun,
H., Blennow, K., De Leon, M., DeCarli, C., Erkin-
juntti, T., Giacobini, E., Graff, C., Hardy, J., Jack, C.,
Jorm, A., Ritchie, K., Van Duijn, C., Visser, P., and
Petersen, R. (2004). Mild cognitive impairment be-
yond controversies, towards a consensus: report of the
international working group on mild cognitive impair-
ment. Journal of Internal Medicine, 256(3):240–246.
Classification of Mild Cognitive Impairment Subtypes using Neuropsychological Data
629