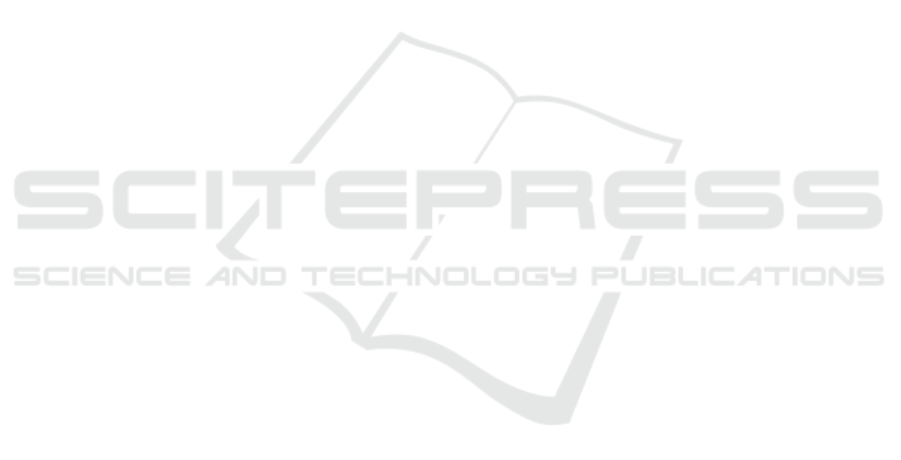
ment captured from the lips and tongue using perma-
nent magnet articulography. Preliminary evaluation
of the system via objective metrics show that the pro-
posed system is able to generate speech of sufficient
quality for some vocabularies. However, problems
still remain to scale up the system to work consis-
tently for phonetically rich tasks. It has also been re-
ported that one of the current limitations of PMA, that
is, the differences between the articulatory data cap-
tured in different sessions, can be greatly reduced by
applying a pre-processing technique to the sensor data
before the conversion. This result brings us closer to
being able to apply the direct synthesis method in a
realistic treatment scenario. These results encourage
us in pursuing our goal of developing a SSI that will
ultimately allow laryngectomised patients to recover
their voice. In order to reach this point, a number
of questions will need to addressed in future research
such as making better use of temporal context, im-
proving the conversion accuracy for a large vocab-
ulary, ways of recovering the prosodic information
(i.e. voicing information and stress), and extending
the technique to speech impaired speakers.
ACKNOWLEDGEMENTS
This is a summary of independent research funded by
the National Institute for Health Research (NIHR)’s
Invention for Innovation Programme. The views ex-
pressed are those of the authors and not necessarily
those of the NHS, the NIHR or the Department of
Health.
REFERENCES
Braz, D. S. A., Ribas, M. M., Dedivitis, R. A., Nishimoto,
I. N., and Barros, A. P. B. (2005). Quality of life and
depression in patients undergoing total and partial la-
ryngectomy. Clinics, 60(2):135–142.
Byrne, A., Walsh, M., Farrelly, M., and O’Driscoll, K.
(1993). Depression following laryngectomy. A pilot
study. The British Journal of Psychiatry, 163(2):173–
176.
Cheah, L. A., Bai, J., Gonzalez, J. A., Ell, S. R., Gilbert,
J. M., Moore, R. K., and Green, P. D. (2015). A user-
centric design of permanent magnetic articulography
based assistive speech technology. In Proc. BioSig-
nals, pages 109–116.
Chen, L.-H., Ling, Z.-H., Liu, L.-J., and Dai, L.-R. (2014).
Voice conversion using deep neural networks with
layer-wise generative training. IEEE/ACM Trans. Au-
dio Speech Lang. Process., 22(12):1859–1872.
Danker, H., Wollbr
¨
uck, D., Singer, S., Fuchs, M., Br
¨
ahler,
E., and Meyer, A. (2010). Social withdrawal af-
ter laryngectomy. European Archives of Oto-Rhino-
Laryngology, 267(4):593–600.
De Jong, S. (1993). SIMPLS: an alternative approach to
partial least squares regression. Chemometrics Intell.
Lab. Syst., 18(3):251–263.
Denby, B., Schultz, T., Honda, K., Hueber, T., Gilbert, J.,
and Brumberg, J. (2010). Silent speech interfaces.
Speech Commun., 52(4):270–287.
Fagan, M. J., Ell, S. R., Gilbert, J. M., Sarrazin, E.,
and Chapman, P. M. (2008). Development of a
(silent) speech recognition system for patients follow-
ing laryngectomy. Medical engineering & physics,
30(4):419–425.
Freitas, J., Teixeira, A., Bastos, C., and Dias, M. (2011).
Speech Technologies, volume 10, chapter Towards a
multimodal silent speech interface for European Por-
tuguese, pages 125–150. InTech.
Fukada, T., Tokuda, K., Kobayashi, T., and Imai, S. (1992).
An adaptive algorithm for Mel-cepstral analysis of
speech. In Proc. ICASSP, pages 137–140.
Ghahramani, Z. and Hinton, G. E. (1996). The EM algo-
rithm for mixtures of factor analyzers. Technical Re-
port CRG-TR-96-1, University of Toronto.
Gilbert, J. M., Rybchenko, S. I., Hofe, R., Ell, S. R., Fagan,
M. J., Moore, R. K., and Green, P. (2010). Isolated
word recognition of silent speech using magnetic im-
plants and sensors. Medical engineering & physics,
32(10):1189–1197.
Gonzalez, J. A., Cheah, L. A., Bai, J., Ell, S. R., Gilbert,
J. M., 1, R. K. M., and Green, P. D. (2014). Anal-
ysis of phonetic similarity in a silent speech interface
based on permanent magnetic articulography. In Proc.
Interspeech, pages 1018–1022.
Herff, C., Heger, D., de Pesters, A., Telaar, D., Brunner,
P., Schalk, G., and Schultz, T. (2015). Brain-to-text:
decoding spoken phrases from phone representations
in the brain. Frontiers in Neuroscience, 9(217).
Hofe, R., Ell, S. R., Fagan, M. J., Gilbert, J. M., Green,
P. D., Moore, R. K., and Rybchenko, S. I. (2013).
Small-vocabulary speech recognition using a silent
speech interface based on magnetic sensing. Speech
Commun., 55(1):22–32.
Hueber, T., Benaroya, E.-L., Chollet, G., Denby, B., Drey-
fus, G., and Stone, M. (2010). Development of a
silent speech interface driven by ultrasound and op-
tical images of the tongue and lips. Speech Commun.,
52(4):288–300.
Hueber, T., Benaroya, E.-L., Denby, B., and Chollet, G.
(2011). Statistical mapping between articulatory and
acoustic data for an ultrasound-based silent speech in-
terface. In Proc. Interspeech, pages 593–596.
Jou, S.-C., Schultz, T., Walliczek, M., Kraft, F., and Waibel,
A. (2006). Towards continuous speech recognition us-
ing surface electromyography. In Proc. Interspeech,
pages 573–576.
Kominek, J. and Black, A. W. (2004). The CMU Arctic
speech databases. In Fifth ISCA Workshop on Speech
Synthesis, pages 223–224.
Kubichek, R. (1993). Mel-cepstral distance measure for ob-
jective speech quality assessment. In Proc. IEEE Pa-
BIOSIGNALS 2016 - 9th International Conference on Bio-inspired Systems and Signal Processing
104