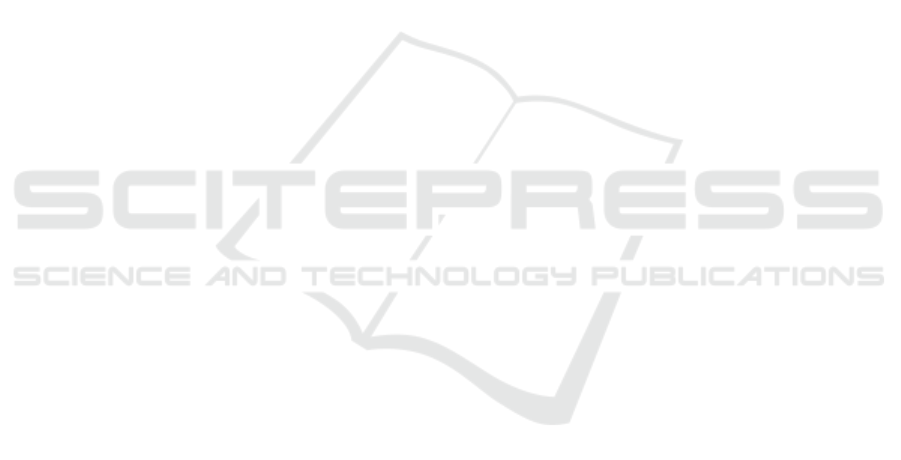
puter Vision and Image Understanding, 114(5):503–
519.
Kollar, T., Tellex, S., Roy, D., and Roy, N. (2014). Ground-
ing verbs of motion in natural language commands
to robots. In Khatib, O., Kumar, V., and Sukhatme,
G., editors, Experimental Robotics, volume 79 of
Springer Tracts in Advanced Robotics, pages 31–47.
Springer Berlin Heidelberg.
Kr
¨
uger, F., Hein, A., Yordanova, K., and Kirste, T. (2015).
Recognising the actions during cooking task (cooking
task dataset). University Library, University of Ros-
tock. http://purl.uni-rostock.de/rosdok/id00000116.
Kr
¨
uger, F., Nyolt, M., Yordanova, K., Hein, A., and Kirste,
T. (2014). Computational state space models for activ-
ity and intention recognition. a feasibility study. PLoS
ONE, 9(11):e109381.
Kr
¨
uger, F., Yordanova, K., Hein, A., and Kirste, T. (2013).
Plan synthesis for probabilistic activity recognition. In
Filipe, J. and Fred, A. L. N., editors, Proceedings of
the 5th International Conference on Agents and Ar-
tificial Intelligence (ICAART 2013), pages 283–288,
Barcelona, Spain. SciTePress.
Kr
¨
uger, F., Yordanova, K., K
¨
oppen, V., and Kirste, T.
(2012). Towards tool support for computational causal
behavior models for activity recognition. In Proceed-
ings of the 1st Workshop: ”Situation-Aware Assistant
Systems Engineering: Requirements, Methods, and
Challenges” (SeASE 2012) held at Informatik 2012,
pages 561–572, Braunschweig, Germany.
Li, X., Mao, W., Zeng, D., and Wang, F.-Y. (2010). Auto-
matic construction of domain theory for attack plan-
ning. In IEEE International Conference on Intelli-
gence and Security Informatics (ISI), 2010, pages 65–
70.
Miller, G. A. (1995). Wordnet: A lexical database for en-
glish. Commun. ACM, 38(11):39–41.
Nguyen, T. A., Kambhampati, S., and Do, M. (2013).
Synthesizing robust plans under incomplete domain
models. In Burges, C., Bottou, L., Welling, M.,
Ghahramani, Z., and Weinberger, K., editors, Ad-
vances in Neural Information Processing Systems 26,
pages 2472–2480. Curran Associates, Inc.
Okeyo, G., Chen, L., Wang, H., and Sterritt, R. (2011).
Ontology-based learning framework for activity assis-
tance in an adaptive smart home. In Chen, L., Nugent,
C. D., Biswas, J., and Hoey, J., editors, Activity Recog-
nition in Pervasive Intelligent Environments, volume 4
of Atlantis Ambient and Pervasive Intelligence, pages
237–263. Atlantis Press.
Perkowitz, M., Philipose, M., Fishkin, K., and Patterson,
D. J. (2004). Mining models of human activities from
the web. In Proceedings of the 13th International
Conference on World Wide Web, WWW ’04, pages
573–582, New York, NY, USA. ACM.
Philipose, M., Fishkin, K. P., Perkowitz, M., Patterson,
D. J., Fox, D., Kautz, H., and Hahnel, D. (2004). In-
ferring activities from interactions with objects. IEEE
Pervasive Computing, 3(4):50–57.
Ramirez, M. and Geffner, H. (2011). Goal recognition over
pomdps: Inferring the intention of a pomdp agent. In
Proceedings of the Twenty-Second International Joint
Conference on Artificial Intelligence, volume 3 of IJ-
CAI’11, pages 2009–2014, Barcelona, Spain. AAAI
Press.
Richter, S. and Westphal, M. (2010). The lama planner:
Guiding cost-based anytime planning with landmarks.
Journal of Artificial Intelligence Research, 39(1):127–
177.
Sil, A. and Yates, E. (2011). Extracting strips representa-
tions of actions and events. In Recent Advances in
Natural Language Processing, pages 1–8.
Tenorth, M., Nyga, D., and Beetz, M. (2010). Understand-
ing and executing instructions for everyday manipu-
lation tasks from the world wide web. In IEEE In-
ternational Conference on Robotics and Automation
(ICRA), pages 1486–1491.
Ye, J., Stevenson, G., and Dobson, S. (2014). Usmart:
An unsupervised semantic mining activity recogni-
tion technique. ACM Trans. Interact. Intell. Syst.,
4(4):16:1–16:27.
Yordanova, K. (2015a). Discovering causal relations in tex-
tual instructions. In Recent Advances in Natural Lan-
guage Processing, pages 714–720, Hissar, Bulgaria.
Yordanova, K. (2015b). Time series from textual instruc-
tions for causal relations discovery (causal relations
dataset). University Library, University of Rostock.
http://purl.uni-rostock.de/rosdok/id00000117.
Yordanova, K. and Kirste, T. (2015). A process for sys-
tematic development of symbolic models for activity
recognition. ACM Transactions on Interactive Intelli-
gent Systems, 5(4).
Yordanova, K., Kr
¨
uger, F., and Kirste, T. (2012). Tool sup-
port for activity recognition with computational causal
behaviour models. In Proceedings of the 35th German
Conference on Artificial Intelligence, pages 561–573,
Saarbr
¨
ucken, Germany.
Yordanova, K., Nyolt, M., and Kirste, T. (2014). Strategies
for reducing the complexity of symbolic models for
activity recognition. In Agre, G., Hitzler, P., Kris-
nadhi, A., and Kuznetsov, S., editors, Artificial In-
telligence: Methodology, Systems, and Applications,
volume 8722 of Lecture Notes in Computer Science,
pages 295–300. Springer International Publishing.
Zhang, Z., Webster, P., Uren, V., Varga, A., and Ciravegna,
F. (2012). Automatically extracting procedural knowl-
edge from instructional texts using natural language
processing. In Calzolari, N., Choukri, K., Declerck,
T., Do
˘
gan, M. U., Maegaard, B., Mariani, J., Moreno,
A., Odijk, J., and Piperidis, S., editors, Proceedings of
the International Conference on Language Resources
and Evaluation (LREC’12), Istanbul, Turkey. Euro-
pean Language Resources Association.
Zhuo, H. H. and Kambhampati, S. (2013). Action-model
acquisition from noisy plan traces. In Proceedings
of the 23rd International Joint Conference on Artifi-
cial Intelligence (IJCAI), pages 2444–2450, Beijing,
China. AAAI.
ICAART 2016 - 8th International Conference on Agents and Artificial Intelligence
422