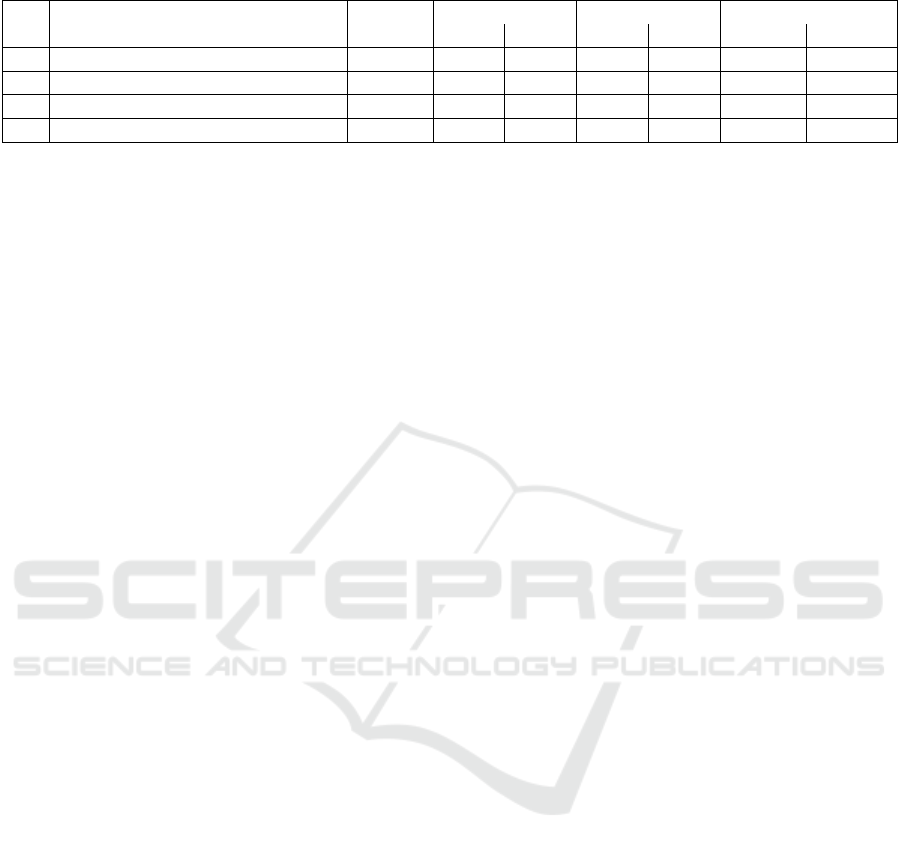
Table 3: Comparison of precision, convergence and convergence point for both observation models.
ID Scenario Mean Mean Prec. % Conv. Mean Conv. Point
Length OM 1 OM 2 OM 1 OM 2 OM 1 OM 2
S1 Fetch and read mail 420 0.383 0.047 1.00 0.00 0.824 -0.002
S2 Go to grocery shopping 2064 0.340 0.728 1.00 0.75 0.910 0.022
S3 Go to work 33602 0.974 0.718 1.00 0.75 0.029 0.032
S4 Morning routine 2869 0.048 0.922 0.00 1.00 -0.0004 0.082
pendent on those. Many of the annotated observation
data sets could be successfully identified, but not all
scenarios could be recognised equally well.
We have shown that it is not sufficient to evalu-
ate the performance of human behaviour reconstruc-
tion solely based on action sequences. Furthermore,
Computational Causal Behaviour Models can easily
be used together with smart home environments.
In the future our approach will be further eval-
uated using other environments, e.g. with multiple
residents and in other flats. Additionally the set of
actions and scenarios will be extended to cover addi-
tional scenarios in the flat such as cooking or sleeping.
The observation model can also be extended by addi-
tional context information, e.g. the personal calendar,
which might influence the reconstruction especially
if the resident is outside of the flat. Another investi-
gation will be the use of other time and observation
models, e.g. a minute based time model and an obser-
vation model that considers delay times. Finally, we
will combine our setting with the ideas presented in
(Yordanova and Kirste, 2016) to learn the necessary
models directly from a natural language text.
REFERENCES
Baker, C. L., Saxe, R., and Tenenbaum, J. B. (2009). Ac-
tion understanding as inverse planning. Cognition,
113(3):329–349.
Bao, L. and Intille, S. (2004). Activity recognition from
user-annotated acceleration data. In Ferscha, A. and
Mattern, F., editors, Pervasive Computing, volume
3001 of LNCS, pages 1–17. Springer.
Blaylock, N. and Allen, J. (2014). Hierarchical goal recog-
nition. In Sukthankar, G., Goldman, R. P., Geib, C.,
Pynadath, D. V., and Bui, H. H., editors, Plan, activity,
and intent recognition, pages 3–32. Elsevier, A’dam.
Bui, H. H., Venkatesh, S., and West, G. A. W. (2002). Policy
Recognition in the Abstract Hidden Markov Model. J.
of Artificial Intelligence Research, 17:451–499.
Fikes, R. E. and Nilsson, N. J. (1971). Strips: A new ap-
proach to the application of theorem proving to prob-
lem solving. In Proc. of the second Int. Joint Conf.
on Artificial Intelligence (IJCAI), pages 608–620, San
Francisco. Morgan Kaufmann.
Hiatt, L., Harrison, A., and Trafton, G. (2011). Accom-
modating human variability in human-robot teams
through theory of mind. In Proc. of the 22nd Int. Joint
Conf. on Artificial Intelligence (IJCAI), pages 2077–
2071, Barcelona, Spain.
Kr
¨
uger, F., Nyolt, M., Yordanova, K., Hein, A., and Kirste,
T. (2014). Computational State Space Models for Ac-
tivity and Intention Recognition. A Feasibility Study.
PLOS ONE, 9(11):e109381.
Lee, S.-W. and Mase, K. (2002). Activity and location
recognition using wearable sensors. Pervasive Com-
puting, IEEE, 1(3):24–32.
Liao, L., Patterson, D. J., Fox, D., and Kautz, H. (2007).
Learning and inferring transportation routines. AI,
171(5-6):311–331.
Mcdermott, D., Ghallab, M., Howe, A., Knoblock, C.,
Ram, A., Veloso, M., Weld, D., and Wilkins, D.
(1998). PDDL - the planning domain definition lan-
guage. Technical Report TR-98-003, Yale Center for
Computational Vision and Control,.
McEwen, A. and Cassimally, H. (2014). Designing the In-
ternet of Things. John Wiley and Sons, Ltd.
Nyolt, M., Kr
¨
uger, F., Yordanova, K., Hein, A., and Kirste,
T. (2015). Marginal filtering in large state spaces. Int.
J. of Approximate Reasoning.
Ram
´
ırez, M. and Geffner, H. (2011). Goal recognition over
POMDPs: inferring the intention of a POMDP agent.
In Proc. of the 22nd IJCAI, pages 2009–2014. AAAI.
Sukthankar, G., Goldman, R. P., Geib, C., Pynadath, D. V.,
and Bui, H. H. (2014). Plan, activity, and intent recog-
nition. Elsevier, A’dam.
van Kasteren, T. L. M. (2011). Activity Recognition for
Health Monitoring Elderly using Temporal Proba-
bilistic Models. PhD thesis, Universiteit van A’dam.
Wilson, D. H. and Atkeson, C. (2005). Simultaneous Track-
ing and Activity Recognition (STAR) Using Many
Anonymous, Binary Sensors. In Pervasive Comput-
ing, volume 3468, pages 62–79. Springer.
Yordanova, K. and Kirste, T. (2016). Learning Models of
Human Behaviour from Textual Instructions. In Proc.
of ICAART 2016, Rome, Italy.
Reconstruction of Everyday Life Behaviour based on Noisy Sensor Data
437