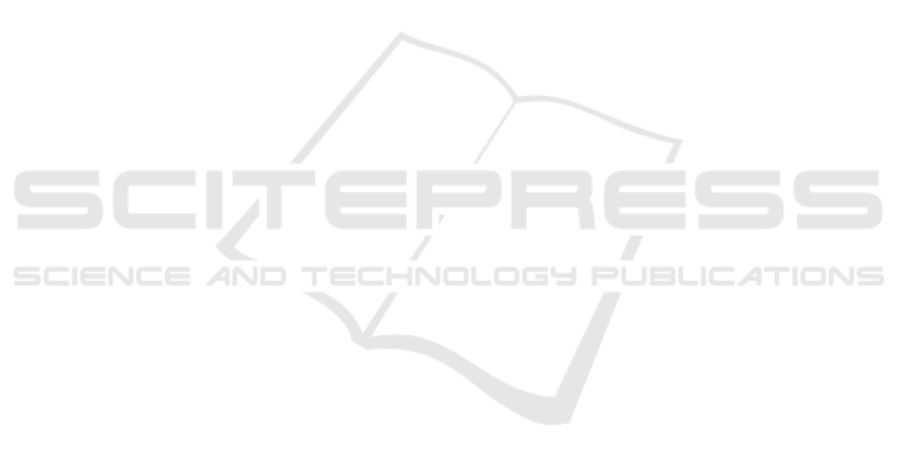
of finding suitable recommendations in different situ-
ations, e.g., for tourists who spend only a very short
time visiting a city. Improvements are also possible
in regards to other solutions of context incorporation.
Currently, we use pre-filtering only, but post-filtering
and contextual modeling should be considered as well
in future work. The contextual pre-filtering immedi-
ately excludes inappropriate events but we should ex-
amine if users are willing to accept little violations of
the thresholds if an event really satisfies their needs.
Furthermore, social recommendations promise an im-
provement of the recommendations. Events of par-
ticular importance for the user, e.g., events the user
liked on social networks like Facebook, could receive
a bonus on the calculated rating to increase the prob-
ability of recommendation. Another aspect is implicit
user feedback which was not collected by this first
prototype but could be used to improve the recom-
mendations. Our first prototype or pure CB or pure
CF approaches could be used as a baseline application
in order to evaluate the suggested extensions and their
influence on the quality of the recommendations. For
this purpose, we want to conduct another study with a
larger number of users which allows us to obtain more
in-depth user feedback and statistics.
ACKNOWLEDGEMENTS
This work is part of the TUM Living Lab Connected
Mobility (TUM LLCM) project and has been funded
by the Bayerisches Staatsministerium f
¨
ur Wirtschaft
und Medien, Energie und Technologie (StMWi).
REFERENCES
Adomavicius, G. and Tuzhilin, A. (2005). Toward the next
generation of recommender systems: A survey of the
state-of-the-art and possible extensions. IEEE Trans.
on Knowl. and Data Eng., 17(6):734–749.
Adomavicius, G. and Tuzhilin, A. (2011). Context-aware
recommender systems. In Ricci, F., Rokach, L.,
Shapira, B., and Kantor, P. B., editors, Recommender
Systems Handbook, pages 217–253. Springer US.
Balabanovi
´
c, M. and Shoham, Y. (1997). Fab: Content-
based, collaborative recommendation. Commun.
ACM, 40(3):66–72.
Burke, R. (2002). Hybrid recommender systems: Survey
and experiments. User Modeling and User-Adapted
Interaction, 12(4):331–370.
Cornelis, C., Guo, X., Lu, J., and Zhang, G. (2005). A
fuzzy relational approach to event recommendation.
In Prasad, B., editor, IICAI, pages 2231–2242. IICAI.
Daly, E. M. and Geyer, W. (2011). Effective event discov-
ery: Using location and social information for scoping
event recommendations. In Proceedings of the Fifth
ACM Conference on Recommender Systems, RecSys
’11, pages 277–280, New York, NY, USA. ACM.
De Pessemier, T., Minnaert, J., Vanhecke, K., Dooms, S.,
and Martens, L.(2013). Social recommendations for
events. In 5th ACM RecSys Workshop on Recom-
mender Systems and the Social Web.
Dey, A. K., Abowd, G. D., and Salber, D. (2001). A
conceptual framework and a toolkit for supporting
the rapid prototyping of context-aware applications.
Hum.-Comput. Interact., 16(2):97–166.
Dooms, S., De Pessemier, T., and Martens, L. (2011).
A user-centric evaluation of recommender algorithms
for an event recommendation system. In Proceed-
ings of the RecSys 2011 : Workshop on Human
Decision Making in Recommender Systems (Deci-
sions@RecSys’11) and User-Centric Evaluation of
Recommender Systems and Their Interfaces - 2
(UCERSTI 2) affiliated with the 5th ACM Conference
on Recommender Systems (RecSys 2011), pages 67–
73.
Herzog, D. and Woerndl, W. (2015). Spontaneous event
recommendations on the go. In Proceedings of the
2nd International Workshop on Decision Making and
Recommender Systems (DMRS2015), Bolzano, Italy,
October 22-23, 2015.
Khrouf, H. and Troncy, R. (2013). Hybrid event recommen-
dation using linked data and user diversity. In Pro-
ceedings of the 7th ACM Conference on Recommender
Systems, RecSys ’13, pages 185–192, New York, NY,
USA. ACM.
Melville, P., Mooney, R. J., and Nagarajan, R. (2002).
Content-boosted collaborative filtering for improved
recommendations. In Eighteenth National Conference
on Artificial Intelligence, pages 187–192, Menlo Park,
CA, USA. American Association for Artificial Intelli-
gence.
Minkov, E., Charrow, B., Ledlie, J., Teller, S., and Jaakkola,
T. (2010). Collaborative future event recommenda-
tion. In Proceedings of the 19th ACM International
Conference on Information and Knowledge Manage-
ment, CIKM ’10, pages 819–828, New York, NY,
USA. ACM.
Oku, K., Nakajima, S., Miyazaki, J., and Uemura, S.
(2006). Context-aware svm for context-dependent in-
formation recommendation. In Proceedings of the 7th
International Conference on Mobile Data Manage-
ment, MDM ’06, pages 109–, Washington, DC, USA.
IEEE Computer Society.
Pazzani, M. J. and Billsus, D. (2007). Content-based rec-
ommendation systems. In Brusilovsky, P., Kobsa, A.,
and Nejdl, W., editors, The Adaptive Web, pages 325–
341. Springer-Verlag, Berlin, Heidelberg.
Quercia, D., Lathia, N., Calabrese, F., Di Lorenzo, G., and
Crowcroft, J. (2010). Recommending social events
from mobile phone location data. In Proceedings
of the 2010 IEEE International Conference on Data
Mining, ICDM ’10, pages 971–976, Washington, DC,
USA. IEEE Computer Society.
Ricci, F. (2011). Mobile recommender systems. Informa-
tion Technology & Tourism, 12(3):205–231.
WEBIST 2016 - 12th International Conference on Web Information Systems and Technologies
302