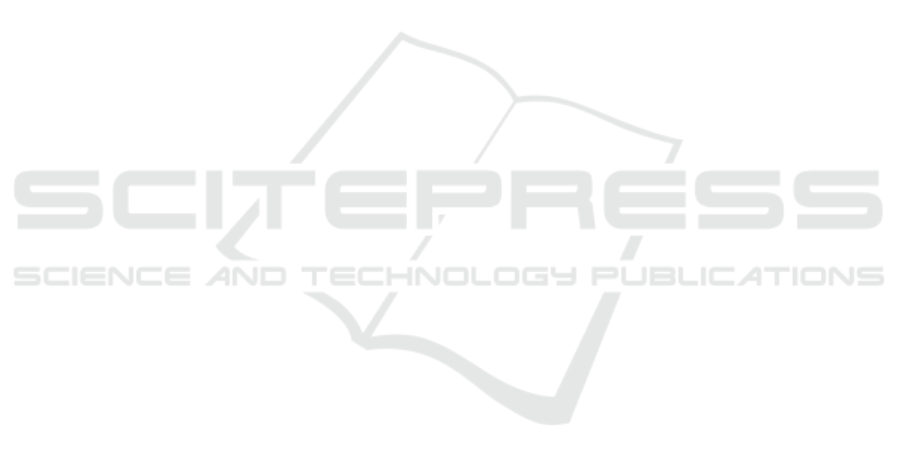
to correctly identify almost all malign workers when
they give at least 20% of misleading ratings.
We also computed the recall of malign workers’
identification as we vary the clustering threshold (τ
C
).
Since workers are marked as cheaters when they fail
to join clusters, a different value for this parameter
could increase or decrease the amount of misleading
ratings the workers should provide to be identified as
cheaters by our framework. Figure 5 shows that the
recall remains stable with varying τ
C
values. On av-
erage, 75% of malign workers that were not identi-
fied as cheaters had only 10% of misleading ratings.
This confirms the previous limit of 20% of mialsedi-
nag ratings as the minimum amount of biased ratings
that a malign worker has to provide to be identified as
a cheater by our system.
Finally, we confirmed the above results using our
real dataset. We added 10 malign workers with 20%
of misleading ratings and the rest of the ratings fol-
lowing the majority of the other workers, and the
framework correctly identified 93% of the malign
workers on average. These results are quite promis-
ing, but marking malign workers as cheaters is only
half of the work. To complete the work, malign work-
ers should not be able pass the verification test. How-
ever, since malign workers are aware of their mislead-
ing ratings, they will be able to reproduce their ratings
when the verification test is run. We leave the iden-
tification of a different verification test that is hard to
pass for malign workers but not for trustful workers
falsely flagged as cheaters to future work.
6 CONCLUSION
We presented a crowdsourcing platform to acquire re-
liable ratings of items. Our data acquisition platform
differs from existing crowdsourcing systems and rec-
ommendation systems because it targets the most ex-
pert users to provide ratings for items with the fewest
number of ratings. Our system relies on incremen-
tal clustering to identify cheaters and a carefully-
designed utility function to assign items to rate to
the most expert workers. Our experimental evaluation
on both synthetic and real restaurant datasets showed
that detecting cheaters, acquiring ratings from expert
workers only, and automating the rating acquisition
process all have a positive impact on both the cost
of acquiring reliable ratings and on improving recom-
mendation accuracy in popular recommendation sys-
tems. In the future, we plan to run more experiments
on other datasets including movie datasets and to de-
sign other utility functions that are most adapted to
such datasets.
REFERENCES
Dawid, A. P. and Skene, A. M. (1979). Maximum likeli-
hood estimation of observer error-rates using the em
algorithm. Applied Statistics, 28(1).
Felfernig, A., Ulz, T., Haas, S., Schwarz, M., Reiterer,
S., and Stettinger, M. (2015). Peopleviews: Human
computation for constraint-based recommendation. In
CrowdRec.
Hacker, S. and von Ahn, L. (2009). Matchin: Eliciting user
preferences with an online game. In CHI.
Hirth, M., Hoßfeld, T., and Tran-Gia, P. (2011). Cost-
Optimal Validation Mechanisms and Cheat-Detection
for Crowdsourcing Platforms. In FINGNet.
Ho, C., Jabbari, S., and Vaughan, J. W. (2013). Adaptive
task assignment for crowdsourced classification. In
ICML.
Ho, C.-J. and Vaughan, J. W. (2012). Online task assign-
ment in crowdsourcing markets. In AAAI.
Ipeirotis, P. G., Provost, F., and Wang, J. (2010). Quality
management on amazon mechanical turk. In KDD,
Workshop on Human Computation.
Joglekar, M., Garcia-Molina, H., and Parameswaran, A.
(2013). Evaluating the crowd with confidence. In
KDD.
Johnson, S. C. (1967). Hierarchical clustering schemes.
Psychometrika, 2.
Karger, D. R., Oh, S., and Shah, D. (2011). Budget-optimal
task allocation for reliable crowdsourcing systems.
CoRR, abs/1110.3564.
Lee, J., Sun, M., and Lebanon, G. (2012). A compara-
tive study of collaborative filtering algorithms. arXiv
preprint arXiv:1205.3193.
Li, H., Zhao, B., and Fuxman, A. (2014). The wisdom of
minority: Discovering and targeting the right group of
workers for crowdsourcing. In WWW.
Mui, L., Mohtashemi, M., and Halberstadt, A. (2002).
A computational model of trust and reputation. In
HICSS.
Roy, S. B., Lykourentzou, I., Thirumuruganathan, S., Amer-
Yahia, S., and Das, G. (2013). Crowds, not drones:
Modeling human factors in interactive crowdsourcing.
In DBCrowd.
Satzger, B., Psaier, H., Schall, D., and Dustdar, S. (2012).
Auction-based Crowdsourcing Supporting Skill Man-
agement. Information Systems, Elsevier.
Tian, Y. and Zhu, J. (2012). Learning from crowds in the
presence of schools of thought. In KDD.
Wolley, C. and Quafafou, M. (2013). Scalable expert selec-
tion when learning from noisy labelers. In ICMLA.
WEBIST 2016 - 12th International Conference on Web Information Systems and Technologies
86