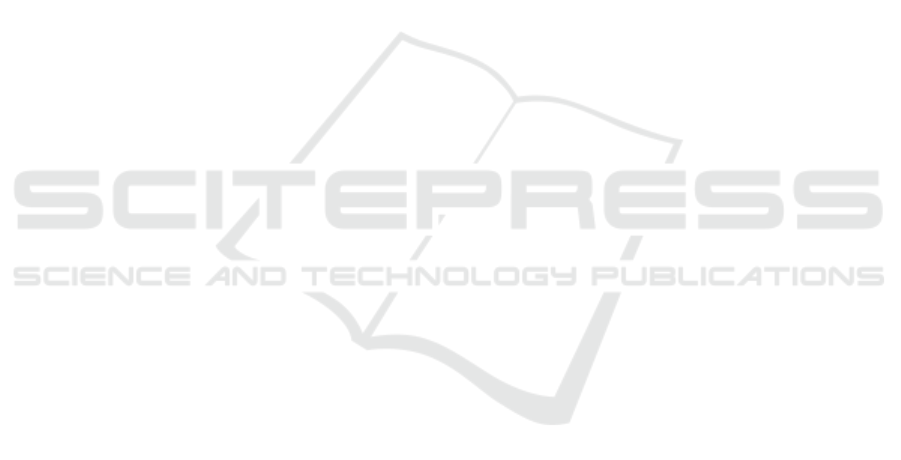
6 CONCLUSIONS
This paper presents our view of the ideals of
information-based medicine, far extending the cur-
rent ideals of evidence-based medicine. We believe
that the concept of information-based medicine has
a potential to become a popular buzzword in the fu-
ture. In general, information-based approaches have
spread already beyond medicine, e.g. to the concept
of information-based policy in political and human
sciences (Prakash and Potoski, 2012), where they re-
place the concept evidence-based policy.
In psychiatry, just like as in other clinical fields,
the concept of information-based medicine expresses
the potential to use new sources of data and knowl-
edge to improve the everyday health care. The past
decades teach us that new knowledge becomes in-
troduced to standard psychiatric care slower com-
pared to other clinical fields. Still, new results of ba-
sic research together with e-health technologies have
an enormous potential to improve the health care
quality, patient safety as well as reduction of ex-
penses. These new results together with new tools
lead us to describing the vision of psychiatric care by
the concept of the information-based psychiatry. This
paper describes the role of medical informatics in the
process of development of information-based psychi-
atry.
However, we must be aware of specific features
of psychiatry which represent serious limitations, bar-
riers or drawbacks for the smooth development of
information-based psychiatry. This paper discusses
such specific features of psychiatry. We also discuss
the current state of the art of basic research in fields
important for the transform of psychiatry towards the
information-based psychiatry. Important tasks in the
process of development of information-based psychi-
atry include utilizing big data for an individual patient
and their combination with new knowledge acquired
by basic research. Other irreplaceable tasks include
computer applications on various levels from the low-
est ones (data storage and integration) up to using ad-
vanced tools of multivariate statistics and data mining
for analyzing big data.
All these aspects together with desirable organi-
zational changes of the psychiatric care are necessary
(but not sufficient) tools endorsing the realization of
the ideals of the paradigm of information-based psy-
chiatry. Its potential is to combine data available from
various sources, to analyze them and to bring the re-
sults as a new psychiatric knowledge closer to the pa-
tient allowing an improved availability, destigmatiza-
tion and humanization of psychiatric care for an indi-
vidual patient.
ACKNOWLEDGEMENTS
The work was financially supported by the Neuron
Fund for Support of Science.
REFERENCES
Bergman, L. G. and Fors, U. G. H. (2008). Decision support
in psychiatry–A comparison between the diagnostic
outcomes using a computerized decision support sys-
tem versus manual diagnosis. BMC Medical Informat-
ics and Decision Making, 8:1–10.
Borang
´
ıu, T. and Purcarea, V. (2008). The future of
healthcare–Information based medicine. Journal of
Medicine and Life, 1:233–237.
Chen, H., Fuller, S. S., Friedman, C., and Hersh, W. (2005).
Medical informatics: Knowledge management and
data mining in biomedicine. Springer, New York.
Daber, R., Sukhadia, S., and Morrissette, J. J. (2013).
Understanding the limitations of next generation se-
quencing informatics, an approach to clinical pipeline
validation using artificial data sets. Cancer Genetics,
206:441–448.
Dentico, D., Cheung, B. L., Chang, J.-Y., Guokas, J.,
Boly, M., Tononi, G., and van Veen, B. (2014). Re-
versal of cortical information flow during visual im-
agery as compared to visual perception. NeuroImage,
100:237–243.
Deslich, S., Stec, B., Tomblin, S., and Coustasse, A.
(2013). Telepsychiatry in the 21st century: Trans-
forming healthcare with technology. Perspectives in
Health Information Management, 10.
Duffau, H., editor (2011). Brain mapping: From neu-
ral basis of cognition to surgical applications, Wien.
Springer.
Eddy, D. M. (1990). Practice policies: Where do they come
from? Journal of the American Medical Association,
263:1265–1275.
Ellegood et al., J. (2015). Clustering autism: Using neu-
roanatomical differences in 26 mouse models to gain
insight into the heterogeneity. Molecular Psychiatry,
20:188–215.
Evans, C. H. and Ildstad, S. T., editors (2001). Small
clinical trials: Issues and challenges, 2001. National
Academy Press.
Guyatt, G., Cairns, J., and Churchill et al., D. (1992).
Evidence-based medicine: A new approach to teach-
ing the practice of medicine. Journal of the American
Medical Association, 268:2420–2425.
Hanson, A. and Levin, B. L. (2013). Mental health infor-
matics. Oxford University Press, Oxford.
Hasman, A., Ammenwerth, E., Dickhaus, H., Knaup, P.,
Lovis, C., Mantas, J., Maojo, V., Martin-Sanchez,
F. J., Musen, M., Patel, V. L., Surjan, G., Talmon, J. L.,
and Sarkar, I. N. (2011). Biomedical informatics–a
confluence of disciplines? Methods of Information in
Medicine, 50:508–524.
Perspectives of Information-based Methods in Medicine: An Outlook for Mental Health Care
369