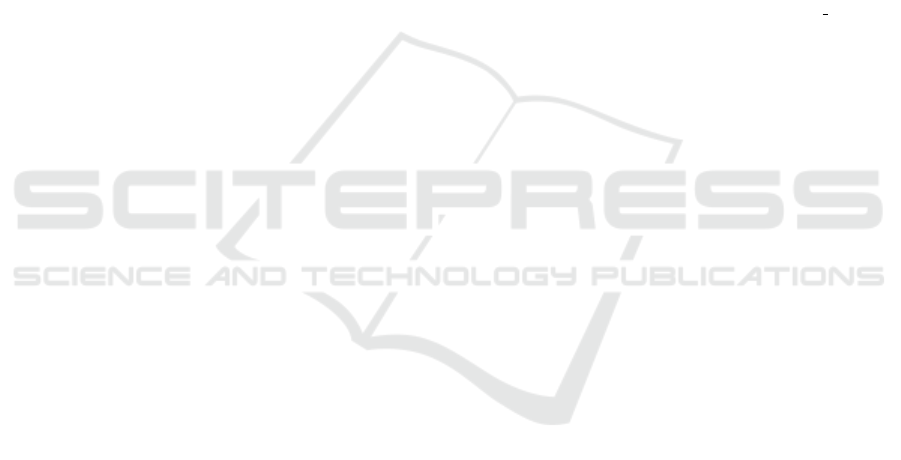
fication procedure gives similar performance results.
A slightly lower recognition accuracy was obtained
on the real-scene dataset due to a number of reasons,
such as the low performance of Tesseract OCR on
texts with complex fonts or designs. On the other
hand, many texts in this dataset present only rota-
tion or slight perspective deformations, compared to
the synthetic dataset, which contains more challeng-
ing texts that are subject to multiple transformations at
the same time. The difficulty of the synthetic dataset
is also proven by the high rectification performance
score. We have demonstrated that the proposed rec-
tification method can successfully correct oriented,
sheared or perspective distorted texts. We have also
shown that we could rectify unreadable texts and ob-
tain satisfactory OCR accuracy scores. Future per-
spectives focus on a deeper analysis on the shape of
some characters such as “A”, “L” or “T” namely a
study of their symmetry to prevent inaccurate quad-
rangle approximations. The evaluation of the recti-
fication procedure is influenced by the used OCR.
Tesseract expects a very accurate text rectification and
often fails when the characters are slightly inclined.
For example, the letter “t” is often interpreted as “f”,
“l” as the symbol “\”, “L” as “Z”. Hence, more per-
formant OCRs are being considered for further tests
such as CuneiForm, ABBYY or OmniPage.
ACKNOWLEDGEMENTS
This work was supported by FUI 14 (LINX project).
REFERENCES
Almazan, J., Fornes, A., and Valveny, E. (2013). De-
formable hog-based shape descriptor. In ICDAR,
pages 1022–1026.
Busta, M., Drtina, T., Helekal, D., Neumann, L., and Matas,
J. (2015). Efficient character skew rectification in
scene text images. In ACCV, pages 134–146.
Calarasanu, S., Fabrizio, J., and Dubuisson, S. (2015). Us-
ing histogram representation and earth mover’s dis-
tance as an evaluation tool for text detection. In IC-
DAR.
Cambra, A. and Murillo, A. (2011). Towards robust and
efficient text sign reading from a mobile phone. In
ICCV, pages 64–71.
Chen, X., Yang, J., Zhang, J., and Waibel, A. (2004). Auto-
matic detection and recognition of signs from natural
scenes. TIP, 13(1):87–99.
Clark, P., Mirmehdi, D., and Doermann, D. (2001). Recog-
nizing text in real scenes. IJDAR, 4:243–257.
Deng, H., Zhu, Q., Tao, J., and Feng, H. (2014). Rec-
tification of license plate images based on hough
transformation and projection. TELKOMNIKA IJEE,
12(1):584–591.
Fan, K. C. and Huang, C. H. (2005). Italic detection and
rectification. JISE, 23:403–419.
Ferreira, S., Garin, V., and Gosselini, B. (2005). A text de-
tection technique applied in the framework of a mobile
camera-based application. In CBDAR, pages 133–139.
Hase, H., Yoneda, M., Shinokawa, T., and Suen, C. (2001).
Alignment of free layout color texts for character
recognition. In ICDAR, pages 932–936.
Kiran, A. G. and Murali, S. (2013). Automatic rectifica-
tion of perspective distortion from a single image us-
ing plane homography. IJCSA, 3(5):47–58.
Li, L. and Tan, C. (2008). Character recognition under se-
vere perspective distortion. In ICPR.
Liang, J., DeMenthon, D., and Doermann, D. (2008). Geo-
metric rectification of camera-captured document im-
ages. PAMI, 30(4):591–605.
Liu, C. and Wang, B. (2015). Icdar 2015
competition on scene text rectification.
http://ocrserv.ee.tsinghua.edu.cn/icdar2015 str/.
Lu, S. and Tan, C. (2006). Camera text recognition based
on perspective invariants. In ICPR, volume 2, pages
1042–1045.
Merino-Gracia, C., Mirmehdi, M., Sigut, J., and Gonz
´
alez-
Mora, J. L. (2013). Fast perspective recovery of text
in natural scenes. IVC, 31(10):714–724.
Myers, G., Bolles, R., Luong, Q.-T., Herson, J., and Arad-
hye, H. (2005). Rectification and recognition of text
in 3-d scenes. IJDAR, 7(2-3):147–158.
Phan, T. Q., Shivakumara, P., Tian, S., and Tan, C. L.
(2013). Recognizing text with perspective distortion
in natural scenes. In ICCV, pages 569–576.
Santosh, K. and Wendling, L. (2015). Character recognition
based on non-linear multi-projection profiles measure.
FCS, 9(5):678–690.
Smith, R. (2007). An overview of the tesseract ocr engine.
In ICDAR, pages 629–633.
Stamatopoulos, N., Gatos, B., Pratikakis, I., and Perantonis,
S. (2011). Goal-oriented rectification of camera-based
document images. TIP, 20(4):910–920.
Yao, C. (2012). Detecting texts of arbitrary orientations in
natural images. In CVPR, pages 1083–1090.
Ye, Q., Jiao, J., Huang, J., and Yu, H. (2007). Text detec-
tion and restoration in natural scene images. VCIR,
18(6):504–513.
Yonemoto, S. (2014). A method for text detection and recti-
fication in real-world images. In ICIV, pages 374–377.
Zhang, L., Lu, Y., and Tan, C. (2004). Italic font recognition
using stroke pattern analysis on wavelet decomposed
word images. In ICPR, volume 4, pages 835–838.
Zhang, X., Lin, Z., Sun, F., and Ma, Y. (2013). Rectification
of optical characters as transform invariant low-rank
textures. In ICDAR, pages 393–397.
Zhou, P., Li, L., and Tan, C. (2009). Character recognition
under severe perspective distortion. In ICDAR, pages
676–680.
VISAPP 2016 - International Conference on Computer Vision Theory and Applications
248