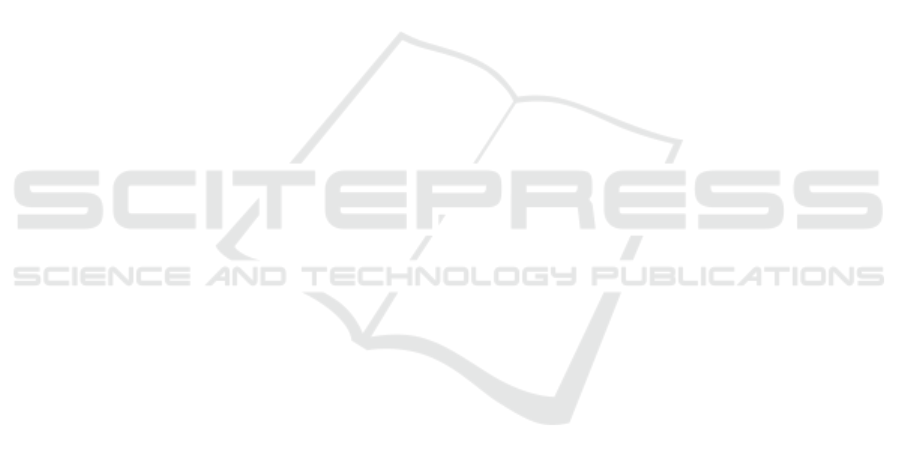
International Conference on Innovations in Informa-
tion Technology (IIT), pages 114–117. IEEE.
Bouchrika, T., Zaied, M., Jemai, O., and Amar, C. B.
(2014). Neural solutions to interact with computers
by hand gesture recognition. Multimedia Tools and
Applications, 72(3):2949–2975.
Dalal, N. and Triggs, B. (2005). Histograms of oriented gra-
dients for human detection. In IEEE Computer Society
Conference on Computer Vision and Pattern Recogni-
tion (CVPR), volume 1, pages 886–893. IEEE.
Dalal, N., Triggs, B., and Schmid, C. (2006). Human de-
tection using oriented histograms of flow and appear-
ance. In Computer Vision–ECCV 2006, pages 428–
441. Springer.
Hou, R., Zamir, A. R., Sukthankar, R., and Shah, M.
(2014). Damn–discriminative and mutually nearest:
Exploiting pairwise category proximity for video ac-
tion recognition. In Computer Vision–ECCV 2014,
pages 721–736. Springer.
Jain, M., J
´
egou, H., and Bouthemy, P. (2013). Better ex-
ploiting motion for better action recognition. In 2013
IEEE Conference on Computer Vision and Pattern
Recognition (CVPR), pages 2555–2562. IEEE.
Kuehne, H., Jhuang, H., Garrote, E., Poggio, T., and Serre,
T. (2011). Hmdb: a large video database for human
motion recognition. In IEEE International Conference
on Computer Vision (ICCV), pages 2556–2563. IEEE.
Laptev, I., Marszałek, M., Schmid, C., and Rozenfeld,
B. (2008). Learning realistic human actions from
movies. In IEEE Conference on Computer Vision and
Pattern Recognition (CVPR), pages 1–8. IEEE.
Peng, X., Wang, L., Wang, X., and Qiao, Y. (2014). Bag of
visual words and fusion methods for action recogni-
tion: Comprehensive study and good practice. arXiv
preprint arXiv:1405.4506.
Perronnin, F., S
´
anchez, J., and Mensink, T. (2010). Improv-
ing the fisher kernel for large-scale image classifica-
tion. In Computer Vision–ECCV 2010, pages 143–
156. Springer.
Raptis, M. and Soatto, S. (2010). Tracklet descriptors for
action modeling and video analysis. In Computer
Vision–ECCV 2010, pages 577–590. Springer.
S
´
anchez, J., Perronnin, F., Mensink, T., and Verbeek, J.
(2013). Image classification with the fisher vector:
Theory and practice. International journal of com-
puter vision, 105(3):222–245.
Sekma, M., Mejdoub, M., and Amar, C. B. (2013). Hu-
man action recognition using temporal segmentation
and accordion representation. In Computer Analysis
of Images and Patterns, pages 563–570. Springer.
Simonyan, K. and Zisserman, A. (2014). Two-stream con-
volutional networks for action recognition in videos.
In Advances in Neural Information Processing Sys-
tems, pages 568–576.
Wang, H., Kl
¨
aser, A., Schmid, C., and Liu, C.-L. (2011).
Action recognition by dense trajectories. In IEEE
Conference on Computer Vision and Pattern Recog-
nition (CVPR), pages 3169–3176. IEEE.
Wang, H. and Schmid, C. (2013). Action recognition with
improved trajectories. In IEEE International Confer-
ence on Computer Vision (ICCV), pages 3551–3558.
IEEE.
Wang, H., Ullah, M. M., Klaser, A., Laptev, I., and Schmid,
C. (2009). Evaluation of local spatio-temporal fea-
tures for action recognition. In British Machine Vision
Conference (BMVC), pages 124–1. BMVA Press.
Wang, L., Qiao, Y., and Tang, X. (2013). Motionlets: Mid-
level 3d parts for human motion recognition. In IEEE
Conference on Computer Vision and Pattern Recogni-
tion (CVPR), pages 2674–2681. IEEE.
Wang, W.-C., Chung, P.-C., Cheng, H.-W., and Huang, C.-
R. (2015). Trajectory kinematics descriptor for trajec-
tory clustering in surveillance videos. In IEEE Inter-
national Symposium on Circuits and Systems (ISCAS),
pages 1198–1201. IEEE.
Yang, X. and Tian, Y. (2014). Action recognition using su-
per sparse coding vector with spatio-temporal aware-
ness. In Computer Vision–ECCV 2014, pages 727–
741. Springer.
Exploiting the Kinematic of the Trajectories of the Local Descriptors to Improve Human Action Recognition
187