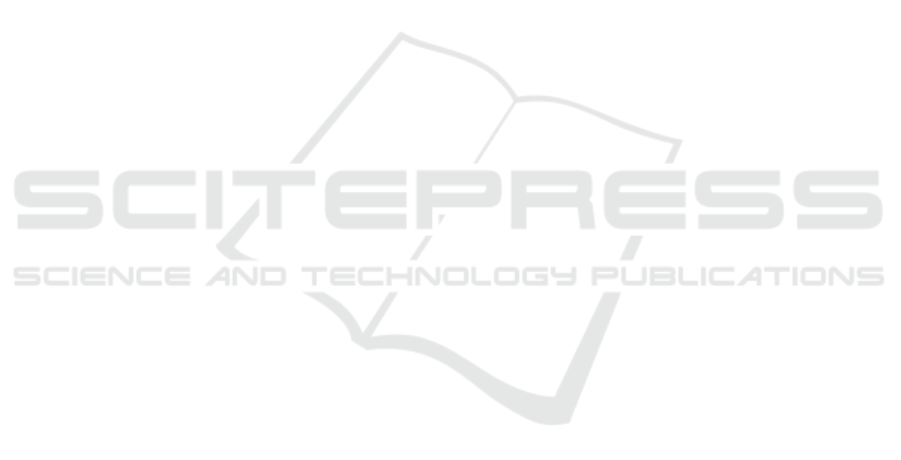
of the centre feature. When this is matched with the
inferred features on the fourth object in Fig. 11, this
seems reasonable.
Finally, the qualitative result show that a diverse
set of features are needed for predicting even slightly
different actions with high rates of success. Exempli-
fied by the fact that only a single prototype is good
for the NPG grasp whereas others are suitable for the
WPG grasp. This is an indication that our unsuper-
vised approach for prototype learning based on the
occurrence in the object set is a reasonable way to go.
6 CONCLUSION
In this paper, we have proposed, the Sliced Pineapple
Grid Feature (SPGF), a novel semi-local shape-based
descriptor, with the aim of utilising it for grasp affor-
dance learning. The descriptor has a number of key
properties such as its ability to encode double sided
structures, encoding of gaps as well as being rota-
tional invariant. As the extraction of a specific dis-
crete set of shape descriptors is based on an unsuper-
vised approach, the amount of features can be tuned
for different applications or object sets. When utilis-
ing the learned features for grasp affordance learning
and afterwards use the learned knowledge on novel
situations, our system is able to predict grasp success
with a rate of up to 96% for individual object classes
and up to 84% when applied to an object set consist-
ing of three classes of objects.
By utilising the learned features, the performance
is better or comparable to the best results achieved in
(Thomsen et al., 2015) performed on the same dataset
despite that the learning conditions in this work are
significantly more difficult. Regardless of the re-
spectable performance of the grasp affordance system
when applied to the full object set, the potential of the
system is not yet fully realized, primarily illustrated
by the fact that the CG performance for the container
objects is well below the highest achieved score for
the individual grasp types WPG and NPG. The pri-
mary reason for this is an unbalanced dataset, as the
chance of randomly selecting a successful WPG grasp
is significantly higher than the chance of randomly se-
lecting a NPG grasp. This means that the votes from
WPG tend to dominate the votes from NPG, resulting
in a worse combined score.
From a qualitative perspective, the learned fea-
tures exhibit similarity to structures that can be iden-
tified as building blocks of objects such as “walls”,
“rims”, “edges”, “surfaces” and others. Furthermore,
the results indicate that different features are suitable
for different affordances, in our work demonstrated
by the two grasp types. Given the intuitiveness of the
learned surface structure, a natural next step is to com-
bine these into more elaborated features.
ACKNOWLEDGEMENT
The research leading to these results has received
funding from the European Community’s Seventh
Framework Programme FP7/2007-2013 (Specific
Programme Cooperation, Theme 3, Information and
Communication Technologies) under grant agree-
ment no. 270273, Xperience.
REFERENCES
Archive3D. Archive3d free online cad model database.
http://www.archive3d.net.
Curtis, N., Xiao, J., and Member, S. (2008). Efficient
and effective grasping of novel objects through learn-
ing and adapting a knowledge base. In IEEE In-
ternational Conference on Robotics and Automation
(ICRA), pages 2252–2257.
Detry, R., Ek, C. H., Madry, M., and Kragic, D. (2013).
Learning a dictionary of prototypical grasp-predicting
parts from grasping experience. In IEEE International
Conference on Robotics and Automation.
Durrant-Whyte, H. and Bailey, T. (2006). Simultaneous lo-
calization and mapping: part i. Robotics Automation
Magazine, IEEE, 13(2):99–110.
Huebner, K., Ruthotto, S., and Kragic, D. (2008). Mini-
mum volume bounding box decomposition for shape
approximation in robot grasping. In Robotics and Au-
tomation, 2008. ICRA 2008. IEEE International Con-
ference on, pages 1628–1633. IEEE.
J. Bohg, A. Morales, T. A. and Kragic, D. (2014). Data-
driven grasp synthesis a survey. IEEE Transactions
on Robotics, 30(2):289–309.
Jiang, Y., Moseson, S., and Saxena, A. (2011). Efficient
grasping from rgbd images: Learning using a new
rectangle representation. In ICRA’11, pages 3304–
3311.
Jørgensen, J. A., Ellekilde, L.-P., and Petersen, H. G.
(2010). RobWorkSim - an Open Simulator for Sensor
based Grasping. Robotics (ISR), 2010 41st Interna-
tional Symposium on and 2010 6th German Confer-
ence on Robotics (ROBOTIK), pages 1 –8.
Kasper, A., Xue, Z., and Dillmann, R. (2012). The KIT
object models database: An object model database
for object recognition, localization and manipulation
in service robotics. The International Journal of
Robotics Research.
Kootstra, G., Popovi
´
c, M., Jørgensen, J. A., Kuklinski, K.,
Miatliuk, K., Kragic, D., and Kr
¨
uger, N. (2012). En-
abling grasping of unknown objects through a syner-
gistic use of edge and surface information. Has been
accepted for Internation Journal of Robotic Research.
A Semi-local Surface Feature for Learning Successful Grasping Affordances
573