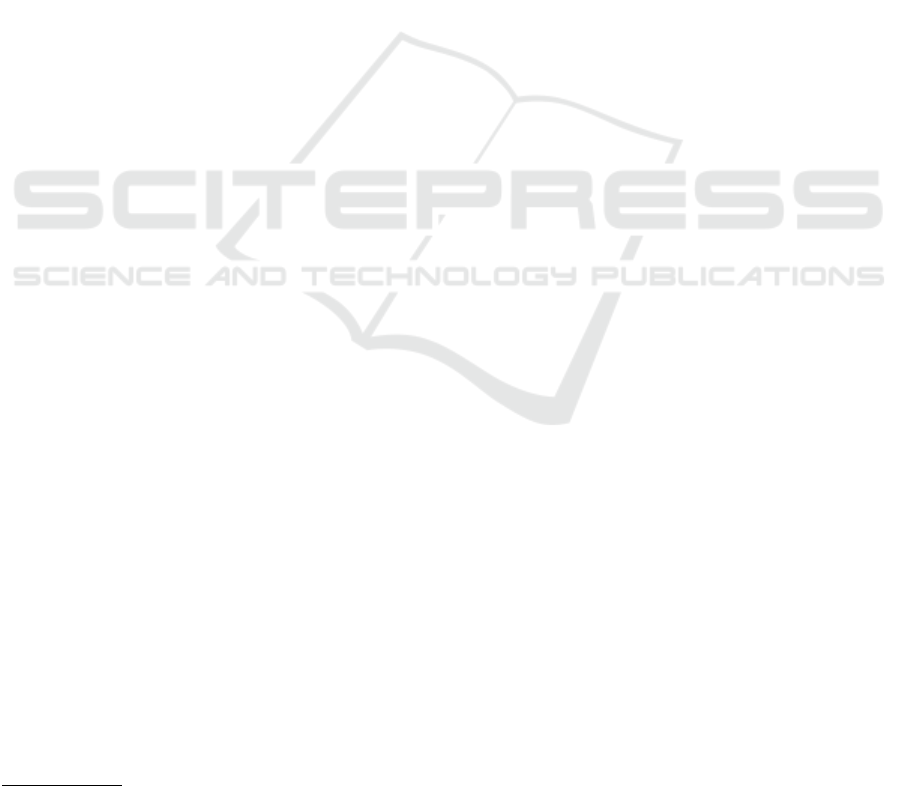
data. However, these systems have no notion of user
context. The same can’t be said for Coveo
10
, a tool
specifically oriented to exploit contextual knowledge
for dealing with information related to customers and
agents. No semantic information, however, is ex-
ploited.
On the other hand, there also a small number of
systems which exploit, even if in a sometimes lim-
ited way, semantic and context information. The On-
togator system (Hyvonen et al., 2003), which is part
of an image management and retrieval system, pro-
vides an interactive recommendation system which
allows the user to browse images based on ontolog-
ical properties. To exploit user contexts, it introduces
views to the ontology that rely on different concept
hierarchies, called “facets”. Each view represents a
specific information-need. IBM’s Content Analytics
with Enterprise Search
11
exploits a framework called
Unstructured Information Management Architecture
(UIMA), in order to build analytic applications and to
find meanings, relationships and relevant facts hidden
in unstructured text. Context information is provided
by means of manual annotations. These approaches
require manual intervention on the documents and/or
adopt a still limited notion of context, i.e. they do not
exploit all of the data potentially available on the user,
such as the contents of any web page visited, attach-
ment downloaded, etc.
7 CONCLUSIONS
In this paper we have presented AMBIT-SE, a se-
mantic enterprise search engine that takes advan-
tage of user-awareness. To this purpose, the en-
gine exploits textual information (coming from sev-
eral sources) about the user, and builds a User se-
mantic glossary, which is exploited to enable effec-
tive user-aware searches on the retrievable informa-
tion, stored in the Website semantic glossary. We have
tested it with different real websites; the results show
that our combined exploitation of synonyms, related
terms and user information leads to very good per-
formance, much better than standard syntactic (enter-
prise) search engines.
With regard to future work, we aim at further op-
timizing the employed similarity metrics and testing
our approach with a wider range of websites. Indeed,
the reported experiments consider a good number of
cases with different features, but more tests can be
useful to further confirm the validity of our approach.
10
http://www.coveo.com/
11
https://www.ibm.com/
ACKNOWLEDGEMENTS
This work was supported by the project “Algo-
rithms and Models for Building context-dependent
Information delivery Tools” (AMBIT) co-funded by
Fondazione Cassa di Risparmio di Modena (SIME
2013.0660).
REFERENCES
Abdou, S. and Savoy, J. (2008). Searching in medline:
Query expansion and manual indexing evaluation. Inf.
Process. Manage., 44(2):781–789.
Baeza-Yates, R. A. and Ribeiro-Neto, B. (1999). Mod-
ern Information Retrieval. Addison-Wesley Longman
Publishing Co., Inc., Boston, MA, USA.
Beneventano, D., Bergamaschi, S., and Martoglia, R.
(2015). Exploiting semantics for searching agricul-
tural bibliographic data. Journal of Information Sci-
ence.
Bergamaschi, S., Martoglia, R., and Sorrentino, S. (2015).
Exploiting semantics for filtering and searching
knowledge in a software development context. Knowl-
edge and Information Systems, 45(2):295–318.
Bernardi, R. (2011). Digital libraries: Ranked evaluation.
Bolchini, C., Orsi, G., Quintarelli, E., Schreiber, F. A.,
and Tanca, L. (2011). Context modeling and con-
text awareness: steps forward in the context-addict
project. Bulletin of the Technical Committee on Data
Engineering, 34:47–54.
Cabri, G., Leonardi, L., Mamei, M., and Zambonelli,
F. (2003). Location-dependent Services for Mo-
bile Users. IEEE Transactions on Systems, Man,
and Cybernetics-Part A: Systems And Humans,
33(6):667–681.
Carpineto, C. and Romano, G. (2012). A survey of auto-
matic query expansion in information retrieval. ACM
Comput. Surv., 44(1):1:1–1:50.
Haslhofer, B., Martins, F., and Magalh
˜
aes, J. a. (2013).
Using skos vocabularies for improving web search.
In Proceedings of the 22Nd International Conference
on World Wide Web, WWW ’13 Companion, pages
1253–1258.
Heflin, J. and Hendler, J. (2000). Searching the web with
shoe. In Artificial Intelligence for Web Search. Papers
from the AAAI Workshop.
Hyvonen, E., Saarela, S., and Viljanen, K. (2003). Ontoga-
tor: combining view- and ontology-based search with
semantic browsing. In Proceedings of XML Finland.
Leacock, C. and Chodorow, M. (1998). Combining local
context and wordnet similarity for word sense identi-
fication. In WordNet: An electronic lexical database.
Liu, F., Yu, C., and Meng, W. (2004). Personalized web
search for improving retrieval effectiveness. IEEE
Trans. on Knowl. and Data Eng., 16(1):28–40.
Mangold, C. (2007). A survey and classification of semantic
search approaches. In Semantics and Ontology.
AMBIT-SE: Towards a User-aware Semantic Enterprise Search Engine
107