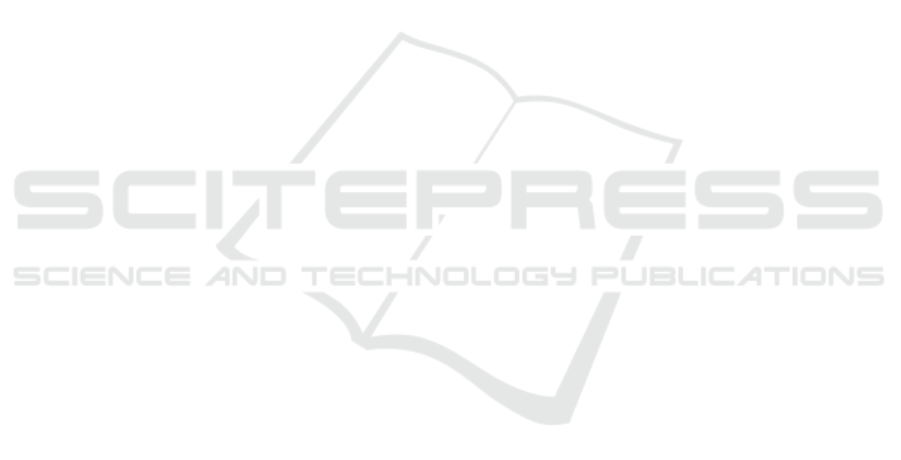
but naive Bayes performs best in our experiment in
both the classification accuracy and efficiency. The
overall accuracy lies between 84.6% and 89.4%, at
which the differences are negligible. Thus, this plat-
form is able to recognize various human activities.
However, all of the tested classifiers confused walk-
ing and using the stairs activities.
The second conclusion is that adding the smart-
watch’s sensor data to the recognition system im-
proves it’s accuracy with at least six percentage point.
Finally, it is computations that the best sampling
frequency is in the field of 10 Hz.
Some questions still require to be answered. Most
important is the conducting of larger experiments
with more people in order to perform more robust
evaluation to clearify if indeed one method is better
than the other, or whether, any off-the-shelf method
can do well in this classification task. This work
could be furhter extended by incorporating more sen-
sors (e.g. heart rate sensor), recognizing high-level
activities (e.g. shopping or eating dinner) or extrapo-
lating these trained classifiers to other people.
ACKNOWLEDGEMENTS
This work was sponsored by Federal Ministry of Ed-
ucation and Research (grant 01IS12050).
REFERENCES
Banos, O., Galvez, J.-M., Damas, M., Pomares, H., and Ro-
jas, I. (2014). Window size impact in human activity
recognition. Sensors, 14(4):6474–6499.
Bao, L. and Intille, S. S. (2004). Activity recognition from
user-annotated acceleration data. In Pervasive com-
puting, pages 1–17. Springer.
Bauman, A., Phongsavan, P., Schoeppe, S., and Owen, N.
(2006). Physical activity measurement-a primer for
health promotion. Promotion & Education, 13(2):92–
103.
Bayat, A., Pomplun, M., and Tran, D. A. (2014). A study
on human activity recognition using accelerometer
data from smartphones. Procedia Computer Science,
34:450–457.
Brezmes, T., Gorricho, J.-L., and Cotrina, J. (2009). Ac-
tivity recognition from accelerometer data on a mo-
bile phone. In Distributed computing, artificial intel-
ligence, bioinformatics, soft computing, and ambient
assisted living, pages 796–799. Springer.
da Costa Cachucho, R. E. G., Moreira, J. M., and Gama, J.
(2011). Activity recognition using, smartphone based,
accelerometer sensors.
Gyllensten, I. C. (2010). Physical activity recognition in
daily life using a triaxial accelerometer. PhD thesis,
KTH Royal Institute of Technology.
Krishnan, N. C., Colbry, D., Juillard, C., and Panchanathan,
S. (2008). Real time human activity recognition us-
ing tri-axial accelerometers. In Sensors, signals and
information processing workshop.
Lee, M. (2009). Physical activity recognition using a single
tri-axis accelerometer. In Proceedings of the world
congress on engineering and computer science, vol-
ume 1.
Lester, J., Choudhury, T., and Borriello, G. (2006). A prac-
tical approach to recognizing physical activities. In
Pervasive Computing, pages 1–16. Springer.
Long, X., Yin, B., and Aarts, R. M. (2009). Single-
accelerometer-based daily physical activity classifica-
tion. In Engineering in Medicine and Biology Society,
2009. EMBC 2009. Annual International Conference
of the IEEE, pages 6107–6110. IEEE.
Parkka, J., Ermes, M., Korpipaa, P., Mantyjarvi, J., Peltola,
J., and Korhonen, I. (2006). Activity classification
using realistic data from wearable sensors. Informa-
tion Technology in Biomedicine, IEEE Transactions
on, 10(1):119–128.
Preece, S. J., Goulermas, J. Y., Kenney, L. P., Howard, D.,
Meijer, K., and Crompton, R. (2009). Activity iden-
tification using body-mounted sensors - a review of
classification techniques. Physiological measurement.
Ravi, N., Dandekar, N., Mysore, P., and Littman, M. L.
(2005). Activity recognition from accelerometer data.
In AAAI, volume 5, pages 1541–1546.
Sharma, A., Purwar, A., Lee, Y.-D., Lee, Y.-S., and Chung,
W.-Y. (2008). Frequency based classification of activ-
ities using accelerometer data. In Multisensor Fusion
and Integration for Intelligent Systems, 2008. MFI
2008. IEEE International Conference on, pages 150–
153. IEEE.
Shoaib, M., Scholten, H., and Havinga, P. J. (2013). To-
wards physical activity recognition using smartphone
sensors. In Ubiquitous Intelligence and Computing,
2013 IEEE 10th International Conference on and 10th
International Conference on Autonomic and Trusted
Computing (UIC/ATC), pages 80–87. IEEE.
Subramanya, A., Raj, A., Bilmes, J. A., and Fox, D. (2012).
Recognizing activities and spatial context using wear-
able sensors. arXiv preprint arXiv:1206.6869.
Telgarsky, R. (2013). Dominant frequency extraction. arXiv
preprint arXiv:1306.0103.
Thiemjarus, S. (2010). A device-orientation independent
method for activity recognition. In Body Sensor
Networks (BSN), 2010 International Conference on,
pages 19–23. IEEE.
Yang, J. (2009). Toward physical activity diary: motion
recognition using simple acceleration features with
mobile phones. In Proceedings of the 1st interna-
tional workshop on Interactive multimedia for con-
sumer electronics, pages 1–10. ACM.
Human Activity Recognition - Using Sensor Data of Smartphones and Smartwatches
493