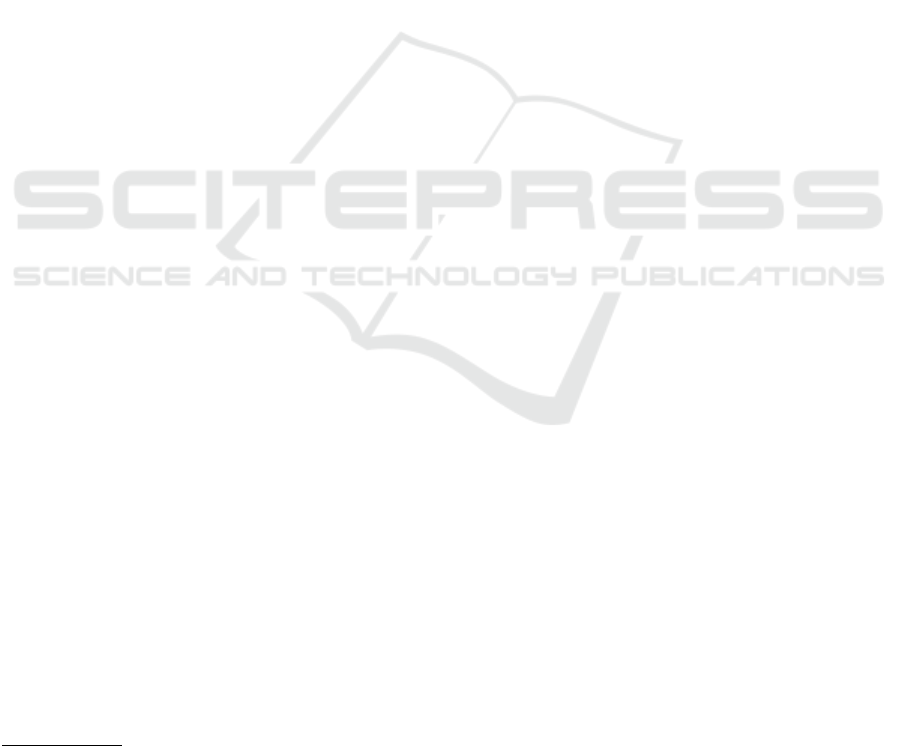
reviews decreases as time passes; this happens both
for reviews with positive arguments and for reviews
with negative arguments. However, such a decrease is
much more steep for negative ones than for positive
ones.
In Fig. 3 we can observe the distribution of posi-
tive and negative arguments.
11
Regarding positive ar-
guments, we cannot exclude a power-law model for
the distribution tail with x-min = 18 and α = 2.56
(pvalue = 0.54)
12
. We also tested a log-normal model
with x-min = 9, µ = 3.01 and σ = 0.81 (pvalue =
0.68). We then searched a common x-min value to
compare the two fitted distributions: for x − min =
4, both the log-normal (µ = 3.03 and σ = 0.78) and
the power-law (α = 1.55) models still cannot be ruled
out, with p − value = 0.57 and pvalue = 0.54 re-
spectively. However, a comparison between the two
leads to a two-sided pvalue = 0.001, which implies
that one model is closer to the true distribution - in
this case, the log-normal model performs better. For
negative arguments, we replicated the distribution fit-
ting: for xmin = 2, a power law model cannot be
ruled out (α = 1.78 and p-value = 0.22) as well as
a log-normal model (µ = 1.48 and σ = 0.96, pvalue =
0.32). Again, after comparing the fitted distributions,
we cannot drop the hypotheses that both the distribu-
tions are equally far from the true distribution (two-
sided pvalue = 0.49). In this case, too few data are
present to make a wise choice.
Among the positive arguments (plot on the left),
there are four arguments that represent, taken to-
gether, almost 44% of customers’ opinions. These ar-
guments are: i) good because the kid loved it, ii) good
because it fits well, iii) good because it has a good
quality/price ratio, iv) good because it has a good
quality. Negative arguments represent, all together,
less than 20% of opinions.
We have a clear view where the pros and cons of
this product are stated as arguments: not surprisingly,
the overall quality is the main reason why customers
consider the product as a good or bad deal. Even
among detractors, this product is not considered ex-
pensive, but quality still is an issue for most of them.
The plots in Fig. 1 show the cumulative frequen-
cies and the rate at which new arguments are added
as a function of time. In the left plot, it is interesting
to note that, despite the difference in volume (positive
arguments are more cited than negative ones), the cu-
mulative frequencies at which positive and negative
arguments are added are almost identical. Positive ar-
11
We used the R poweRlaw package for heavy tailed distri-
butions (developed by Colin Gillespie (Gillespie, 2015)).
12
We used the relatively conservative choice that the power
law is ruled out if pvalue 0.1 (Clauset et al., 2009).
guments start being posted earlier than negative ones,
consistently with the fact that enthusiast customers
are the first that review the product. Moreover, it is
interesting to note that no new positive argument is
added in the 2011-2013 interval, while some negative
ones arise in the reviews. Since 2013, positive and
negative arguments follow a similar trajectory. How-
ever, as can be noted in the second plot on the right,
new arguments are not added at the same pace. If we
consider the total amount of added arguments, posi-
tive ones are repeated more often than negatives, and
the rate at which a new positive argument is added is
considerably lower than its counterpart. This infor-
mation sheds a light on customers’ behaviour: dissat-
isfied customers tend to post new reasons why they
dislike the product, more than just repeating what
other dissatisfied customers have already said.
5 SIMULATION
In this section we propose an agent-based simulation
to replicate empirical data about customers, reviews,
and arguments as described in Section 4. The aim of
this step is to translate the theoretical mechanism used
to write reviews into its computational counterpart.
Following J. Moody (Moody, 2008), our aim is to
specify a substance-specific model that can shed light
on how customers behave when they have to review
a product, thus to identify properties that make real-
world data and simulated data differ, without quanti-
fying these differences with a statistical significance.
We opt for the Agent-Based Modelling (ABM)
computational approach (Macy and Willer, 2002) to
simulate arguments networks of online reviews from
user behaviour. There is a growing literature that uses
ABM in network studies (Macy and Skvoretz, 1998;
Flache and Macy, 2011). ABMs are a straightfor-
ward way to detail and implement substance-specific
mechanisms in the form of computational models,
i.e., software that generates entities with attributes and
decision-making rules, and that is goal-oriented.
Despite the specific solution implemented, the
main logic would be to test different specifications
of the mechanism against empirical data and to re-
fine such implementations until a satisfactory match is
found or, alternatively, to get back to the blackboard
and think again about the hypotheses - agent-based
modelling is, ultimately, a tool to aid theory building.
An interesting analytical strategy to understand
the robustness of an ABM is to compare its results
against empirical data (Manzo, 2007) in order to as-
sess how realistic the model behaves - thus how sound
is the theory behind it. We will also compare the re-
ICAART 2016 - 8th International Conference on Agents and Artificial Intelligence
78