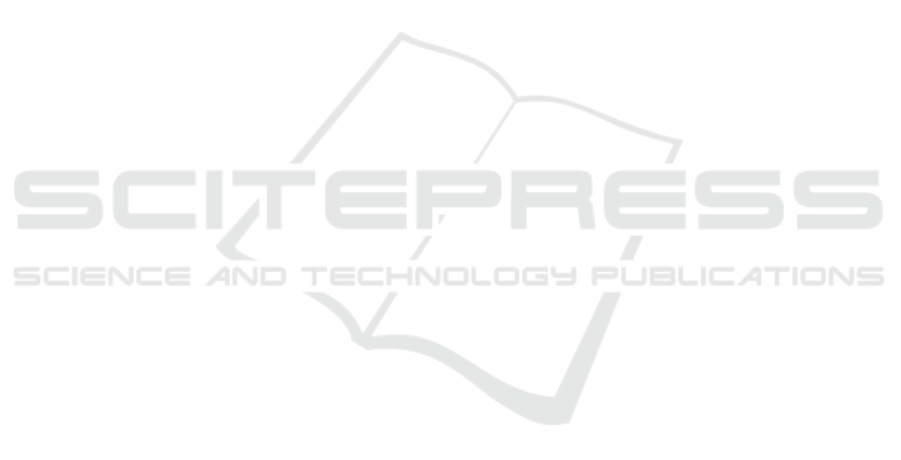
(Zhu et al., 2011) considered the age reduction coef-
ficient approach of (Malik, 1979) to model imperfect
preventive maintenance. (Panday et al., 2013) also
studied the selective maintenance problem for binary
systems under imperfect PM. They considered, the
hybrid hazard rate model introduced by (Lin et al.,
2000) to model imperfect maintenance actions. The
authors in (Djelloul et al., 2015) considered selective
maintenance problem in the case the system operates
missions of random duration.
The selective maintenance studied in the above
mentioned works merely rely on binary state systems
having only two operating states, namely function-
ing and failure states. However, many industrial sys-
tems are designed to operate their tasks according to
a range of performance levels varying from perfect
functioning to complete failure. Such systems are
known as multi-state systems (MSS). Dealing with
selective maintenance of MSS, only few works ap-
peared in the literature. The first work is reported
in (Chen et al., 1999). Each system’s component as
well as the system itself may be in one of the (K + 1)
possible states. Replacement of failed components
is the only available maintenance option. A mainte-
nance optimization problem is then derived to min-
imize the total maintenance cost while providing a
given required system reliability level for the next
mission. To solve this problem, a procedure based on
the short path method is proposed. In (Liu and Huang,
2010), the authors studied the selective maintenance
problem for a MSS where components are character-
ized by two operating states (functioning and failure
states) while the system performs several states with
different output performance levels. Several mainte-
nance options are also considered from minimal re-
pair to replacement through imperfect maintenance.
A genetic algorithm is used to solve the resulting se-
lective maintenance optimization problem. To over-
come the restrictive hypothesis of binary components
in (Liu and Huang, 2010), (Pandey et al., 2013) stud-
ied selective maintenance for a series-parallel MSS
where components are characterized by more than
one performance level (i.e. multi-state components).
The transition rates between states of a component
are assumed constants, i.e. components are mod-
eled as continuous-time Markov chain. In (Khatab
and Ait-Kadi, 2008), the authors generalized the se-
lective maintenance optimization problem to consider
more than one mission. To improve the reliability of
the system, preventive maintenance actions are per-
formed during breaks. The selective maintenance
problem consists on finding an optimal sequence of
maintenance actions the cost of which minimizes the
total maintenance cost while providing the desired
system reliability level for each mission. The result-
ing optimization problem is solved using the extended
great deluge algorithm.
Dealing with the MSS selective maintenance
problem, all the above mentioned papers assumed
that the duration of the break as well as that of the
next mission are both known and constant. However,
this assumption may no longer be valid in many real-
world situations where it is usually difficult to eval-
uate the exact duration of a mission. Indeed, such
duration may unfortunately be impacted by the occur-
rence of random events which lead the system either
to abort the mission or at most to continue operating
the mission but with more additional time. Similarly,
the occurrence of random events may conduct the de-
cision maker to shorten or even to extend the break
duration. As a consequence, it is more realistic and
practical to consider that mission as well as break du-
rations are not precisely known but rather random and
should therefore be governed by appropriate probabil-
ity distributions.
The present paper addresses the selective main-
tenance problem for a MSS systems when the du-
ration of the next mission and that of the break are
stochastic and modeled as random variables. Right
after each mission, the system becomes available for
maintenance during a limited duration of the break,
to meet the required reliability level for the execution
of the next mission. Due to the limited maintenance
resources, not all components are likely to be main-
tained. The selective maintenance decision problem
to be solved consists first in selecting a subset of com-
ponents and then choosing the level of maintenance
to be performed on each of the selected components.
The objective function may consist of maximizing the
successful completion of the next mission while tak-
ing into account maintenance budget and time allotted
to the break, or of minimizing the total maintenance
cost subject to the required reliability level and the
time allotted to the break, or of minimizing the to-
tal maintenance time subject to the required reliability
level and the maintenance budget. The present paper
considers the second objective function. The stochas-
tic selective maintenance problem is then formulated
and solved.
The remainder of this paper is organized as fol-
lows. Section 2 describes the investigated system
and defines its reliability. Section 2 shows how the
z−transform is used to estimate MSS reliability. Sec-
tion 3 presents the imperfect maintenance model and
defines time and cost for each maintenance action.
Section 4 discusses the reliability computation to op-
erate missions of random durations. The stochastic
selective maintenance optimization model is devel-
Selective Maintenance for Failure-prone Multi-state Systems When the Durations of Missions and Scheduled Breaks Are Stochastic
211