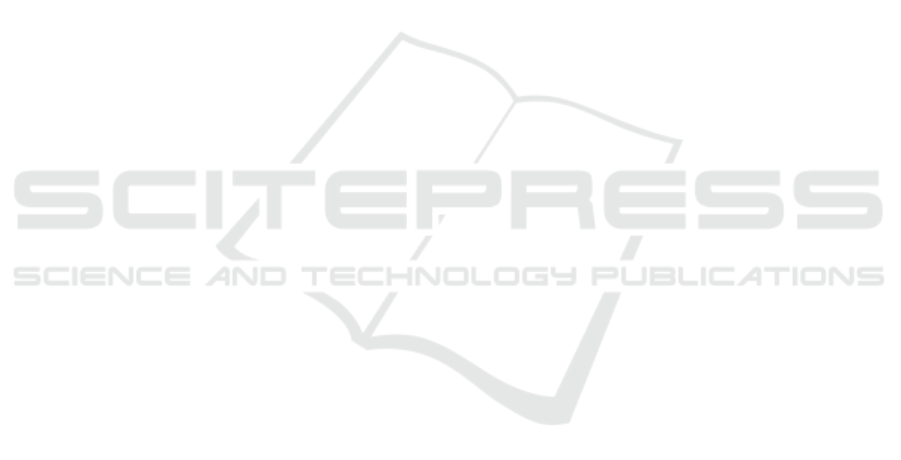
ACKNOWLEDGEMENT
The work on this article has been supported by
the project ‘Representation of dynamic 3D scenes
using the Atomic Shapes Network model’ fi-
nanced by National Science Centre, decision DEC-
2011/03/D/ST6/03753.
REFERENCES
Bhaskaran, S., Datt, B., Forster, T., T., N., and Brown, M.
(2004). Integrating imaging spectroscopy (445-2543
nm) and geographic information systems for post-
disaster management: A case of hailstorm damage
in sydney. International Journal of Remote Sensing,
25(13):2625–2639.
Bioucas-Dias, J. M., Plaza, A., Dobigeon, N., Parente, M.,
Du, Q., Gader, P., and Chanussot, J. (2012). Hy-
perspectral unmixing overview: Geometrical, statis-
tical, and sparse regression-based approaches. Se-
lected Topics in Applied Earth Observations and Re-
mote Sensing, IEEE Journal of, 5(2):354–379.
Chapelle, O., Haffner, P., and Vapnik, V. (1999). Sup-
port vector machines for histogram-based image clas-
sification. Neural Networks, IEEE Transactions on,
10(5):1055–1064.
Chen, Y., Nasrabadi, N. M., and Tran, T. D. (2013). Hy-
perspectral image classification via kernel sparse rep-
resentation. Geoscience and Remote Sensing, IEEE
Transactions on, 51(1):217–231.
Denoyer, L. and Gallinari, P. (2004). Bayesian network
model for semi-structured document classification. In-
formation Processing & Management, 40(5):807 –
827.
Ellis, J. (2003). Hyperspectral imaging technologies key for
oil seep/oil-impacted soil detection and environmental
baselines. Environmental Science and Engineering.
Retrieved on February, 23:2004.
Fang, L., Li, S., Kang, X., and Benediktsson, J. A. (2015).
Spectral–spatial classification of hyperspectral images
with a superpixel-based discriminative sparse model.
Geoscience and Remote Sensing, IEEE Transactions
on, 53(8):4186–4201.
Friedl, M. and Brodley, C. (1997). Decision tree classifica-
tion of land cover from remotely sensed data. Remote
Sensing of Environment, 61(3):399 – 409.
Kuncheva, L. (2002). A theoretical study on six classifier
fusion strategies. Pattern Analysis and Machine Intel-
ligence, IEEE Transactions on, 24(2):281–286.
Kuncheva, L., Bezdek, J., and Duin, R. (2001). Decision
templates for multiple classifier fusion: an experimen-
tal comparison. Pattern Recognition, 34:299–314.
Manian, V. and Jimenez, L. O. (2007). Land cover and ben-
thic habitat classification using texture features from
hyperspectral and multispectral images. Journal of
Electronic Imaging, 16(2):023011–023011–12.
Melgani, F. and Bruzzone, L. (2004). Classification of hy-
perspectral remote sensing images with support vec-
tor machines. Geoscience and Remote Sensing, IEEE
Transactions on, 42(8):1778–1790.
Perrone, M. P. and Cooper, L. (1993). When networks dis-
agree: Ensemble methods for hybrid neural networks.
pages 126–142. Chapman and Hall.
Pu, Y.-Y., Feng, Y.-Z., and Sun, D.-W. (2015). Re-
cent progress of hyperspectral imaging on quality and
safety inspection of fruits and vegetables: A review.
Comprehensive Reviews in Food Science and Food
Safety, 14(2):176–188.
Rojas, M., D
´
opido, I., Plaza, A., and Gamba, P. (2010).
Comparison of support vector machine-based pro-
cessing chains for hyperspectral image classification.
In SPIE Optical Engineering+ Applications, pages
78100B–78100B. International Society for Optics and
Photonics.
Ruta, D. and Gabrys, B. (2000). An overview of classifier
fusion methods.
Wolpert, D. (1992). Stacked generalization. Neural Net-
works, 5:241–259.
Xu, L. and Li, J. (2014). Bayesian classification of hyper-
spectral imagery based on probabilistic sparse repre-
sentation and markov random field. Geoscience and
Remote Sensing Letters, IEEE, 11(4):823–827.
Two Stage SVM Classification for Hyperspectral Data
391