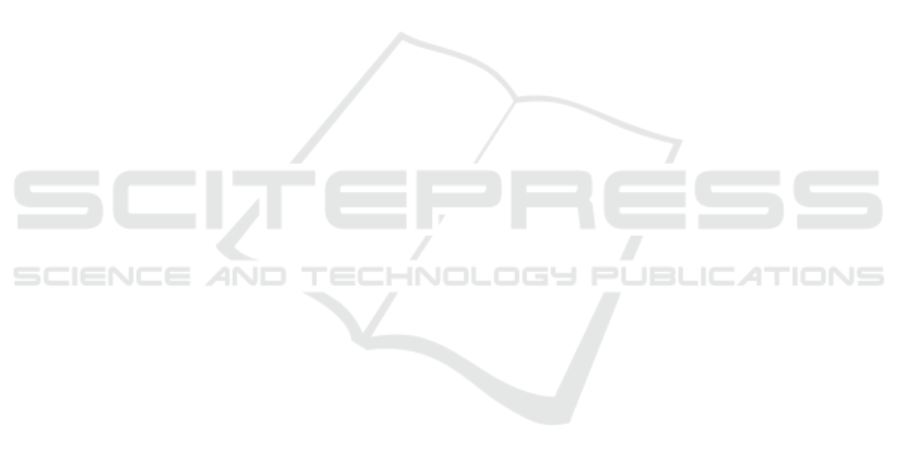
estimation. In order to evaluate the model we used a
public dataset and verified that the approach used is
capable of classifying activities according to the SRK
model, which is useful for the risk analysis.
In future works, we intend to create an adaptive al-
gorithm that learns with the user’s behavior and adjust
the values for the thresholds used for the classification
of a behavior as skill, rule or knowledge. We also in-
tend to improve the approach for actions prediction,
considering the evaluation of the performance of the
algorithms. Besides that, we intend to perform tests in
public datasets with a higher number of user’s physio-
logical information in order to allow a more complete
evaluation of the calculated risks.
REFERENCES
Cook, D. (2011). Learning setting-generalized activity
models for smart spaces. IEEE Intelligent Systems.
Craven, P. L., Belov, N., Tremoulet, P., Thomas, M., Berka,
C., Levendowski, D., and Davis, G. (2007). Cog-
nitive workload gauge development: comparison of
real-time classification methods. Foundations of Aug-
mented Cognition, pages 75–84.
Curone, D., Tognetti, A., Secco, E. L., Anania, G., Car-
bonaro, N., De Rossi, D., and Magenes, G. (2010).
Heart rate and accelerometer data fusion for activ-
ity assessment of rescuers during emergency interven-
tions. Information Technology in Biomedicine, IEEE
Transactions on, 14(3):702–710.
da Rocha, C. C., Lima, J. C. D., Viera, M., Capretz, M. A.,
Bauer, M. A., Augustin, I., and Dantas, M. A. (2010).
A context-aware authentication approach based on be-
havioral definitions. In IKE, pages 178–184.
Gil-Quijano, J. and Sabouret, N. (2010). Prediction of hu-
mans’ activity for learning the behaviors of electri-
cal appliances in an intelligent ambient environment.
In Web Intelligence and Intelligent Agent Technology
(WI-IAT), 2010 IEEE/WIC/ACM International Con-
ference on, volume 2, pages 283–286. IEEE.
Katz, S., Ford, A. B., Moskowitz, R. W., Jackson, B. A., and
Jaffe, M. W. (1963). Studies of illness in the aged: the
index of adl: a standardized measure of biological and
psychosocial function. Jama, 185(12):914–919.
Kofod-Petersen, A. and Cassens, J. (2006). Using activity
theory to model context awareness. In Modeling and
Retrieval of Context, pages 1–17. Springer.
Kriegel, H.-P., Kr
¨
oger, P., Schubert, E., and Zimek, A.
(2009). Loop: local outlier probabilities. pages 1649–
1652.
Kuutti, K. (1996). Activity theory as a potential framework
for human-computer interaction research. pages 17–
44.
Mikalsen, M. and Kofod-Petersen, A. (2004). Representing
and reasoning about context in a mobile environment.
pages 25–35.
Naeem, U., Bigham, J., and Wang, J. (2007). Recognis-
ing activities of daily life using hierarchical plans. In
Smart Sensing and Context, pages 175–189. Springer.
Neto, A. D. F., Boufleuer, R., Romero de Azevedo, B.,
Augustin, I., Carlos D. Lima, J., and C. Rocha, C.
(2013). Towards a middleware to infer the risk level
of an activity in context-aware environments using the
srk model. In UBICOMM 2013, The Seventh Interna-
tional Conference on Mobile Ubiquitous Computing,
Systems, Services and Technologies, pages 38–42.
Neto, A. D. F., de Azevedo, B. R., Boufleuer, R., Lima,
J. C. D., Augustin, I., and Pasin, M. (2014). An ap-
proach based on activity theory and the srk model for
risk and performance evaluation of human activities
in a context-aware middleware. In Proceedings of the
13th International Conference on Mobile and Ubiqui-
tous Multimedia, pages 40–47. ACM.
ODonnell, R.D. & Eggemeier, F. (1986). Workload assess-
ment methodology. In Boff, K., Kaufman, L., and
Thomas, J., editors, Handbook of perception and hu-
man performance., volume 2, pages 42/1–42/49. John
Wiley & Sons.
Paas, F., Tuovinen, J. E., Tabbers, H., and Van Gerven, P. W.
(2003). Cognitive load measurement as a means to
advance cognitive load theory. Educational psycholo-
gist, 38(1):63–71.
Rasch, K. (2013). Smart assistants for smart homes.
Rasmussen, J. (1983). Skills, rules, and knowledge; sig-
nals, signs, and symbols, and other distinctions in hu-
man performance models. Number 3, pages 257–266.
IEEE.
Wang, C., Zheng, Q., Peng, Y., De, D., and Song, W.-
Z. (2014). Distributed abnormal activity detection
in smart environments. International Journal of Dis-
tributed Sensor Networks, 2014.
Recognition of Human Activities using the User’s Context and the Activity Theory for Risk Prediction
289