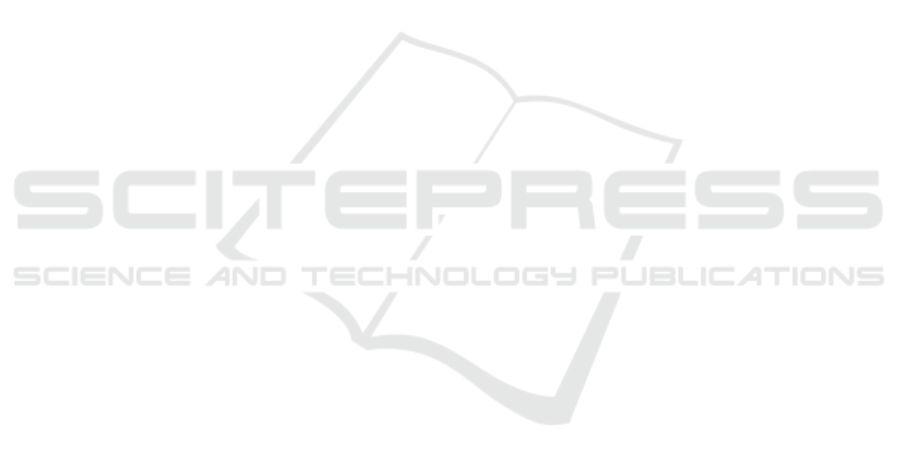
computationally efficient. We provide examples that
show that our approach can fruitfully solve few ev-
ident drawbacks of the general model, as applied to
information flow forecast.
There are at least three different ways in which
this investigation can be extended. First of all we aim
at formalising a problem of dissemination of informa-
tion pieces throughout a network. The problem can be
formulated as follows: given a social network, a num-
ber k and a probability value p, select k members in
such a way that the set of members reached by an in-
formation piece sent to the members in the selection
and dissemintated by them and the chains of mem-
bers generated therefore, has a probability of being
total (namely to cover the entire network) of at least
p.
A second study investigates ways of providing
reacher models of topics. In particular, we aim at in-
vestigating topics with sub-topics.
A third investigation will introduce the notion of
orientation. Foir instance two persons can be both
interested in football, but one may support Juventus
F.C., whilst the other one may support A.C. Chievo
Verona. These studies are taken into a track of re-
search for viral marketing purposes, including meth-
ods to evaluate networks for advertisment, alerts, and
emergencies.
REFERENCES
A. Ramachandra Rao, S. B. (1987). Measures of reciprocity
in a social network. Sankhy: The Indian Journal of
Statistics, Series A (1961-2002), 49(2):141–188.
Alguliev, R., Aliguliyev, R., and Ganjaliyev, F. (2011). In-
vestigation of the role of similarity measure and rank-
ing algorithm in mining social networks. Journal of
Information Science, 37(3):229–234.
Cilibrasi, R. and Vit
´
anyi, P. M. B. (2007). The google
similarity distance. IEEE Trans. Knowl. Data Eng.,
19(3):370–383.
Cruz, J. D., Bothorel, C., and Poulet, F. (2014). Commu-
nity detection and visualization in social networks: In-
tegrating structural and semantic information. ACM
Trans. Intell. Syst. Technol., 5(1):11:1–11:26.
Davoodi, E., Kianmehr, K., and Afsharchi, M. (2013). A se-
mantic social network-based expert recommender sys-
tem. Applied Intelligence, 39(1):1–13.
Downes, S. (2005). Semantic networks and social networks.
The Learning Organization, 12(5):411–417.
Eppstein, D. (1999). Finding the k shortest paths. SIAM J.
Comput., 28(2):652–673.
Er
´
et
´
eo, G., Gandon, F. L., Corby, O., and Buffa, M.
(2009). Semantic social network analysis. CoRR,
abs/0904.3701.
Kang, S. M. (2007). A note on measures of similarity based
on centrality. Social Networks, 29(1):137 – 142.
Landherr, A., Friedl, B., and Heidemann, J. (2010). A
critical review of centrality measures in social net-
works. Business and Information Systems Engineer-
ing, 2(6):371–385.
Leydesdorff, L. (2012). Advances in science visualiza-
tion: Social networks, semantic maps, and discursive
knowledge. CoRR, abs/1206.3746.
Mika, P. (2004). Social networks and the semantic web.
In Web Intelligence, 2004. WI 2004. Proceedings.
IEEE/WIC/ACM International Conference on, pages
285–291.
Mika, P. (2005). Flink: Semantic web technology for the
extraction and analysis of social networks. Web Se-
mantics: Science, Services and Agents on the World
Wide Web, 3(2):211 – 223. Selcted Papers from
the International Semantic Web Conference, 2004
ISWC, 20043rd. International Semantic Web Confer-
ence, 2004.
Tonta, Y. and Darvish, H. R. (2010). Diffusion of latent
semantic analysis as a research tool: A social network
analysis approach. Journal of Informetrics, 4(2):166
– 174.
Yuan, S.-T. and Fei, Y.-L. (2010). A synthesis of seman-
tic social network and attraction theory for innovating
community-based e-service. Expert Systems with Ap-
plications, 37(5):3588 – 3597.
Zhang, Y., Chen, H., and Wu, Z. (2006). A social network-
based trust model for the semantic web. In Yang,
L., Jin, H., Ma, J., and Ungerer, T., editors, Au-
tonomic and Trusted Computing, volume 4158 of
Lecture Notes in Computer Science, pages 183–192.
Springer Berlin Heidelberg.
Zhou, L., Ding, L., and Finin, T. (2011). How is the se-
mantic web evolving? a dynamic social network per-
spective. Computers in Human Behavior, 27(4):1294
– 1302. Social and Humanistic Computing for the
Knowledge Society.
Zywica, J. and Danowski, J. (2008). The faces of facebook-
ers: Investigating social enhancement and social com-
pensation hypotheses; predicting facebook and offline
popularity from sociability and self-esteem, and map-
ping the meanings of popularity with semantic net-
works. Journal of Computer-Mediated Communica-
tion, 14(1):1–34.
Semantic Social Network Analysis Foresees Message Flows
303