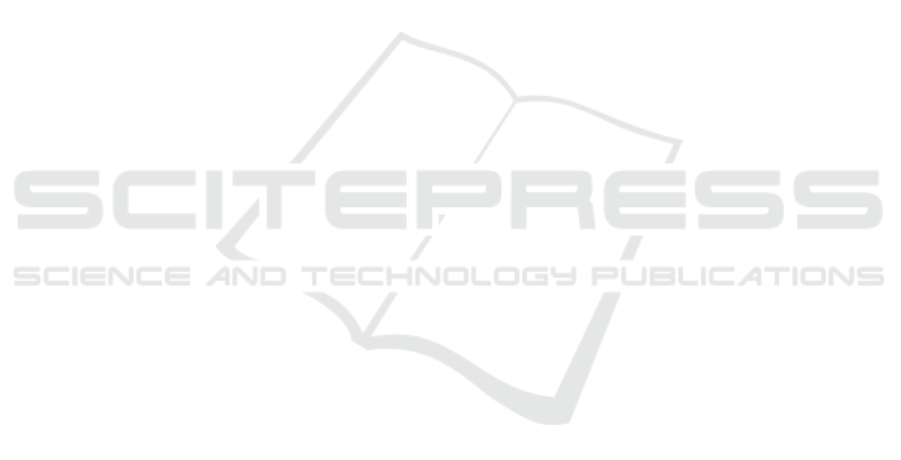
ru
´
ıdo urbano. In SIMGEO ’10, pages 001–0010.
Barczyszyn, G. L. (2015). Integrac¸
˜
ao de dados geogr
´
aficos
para planejamento urbano da cidade de Curitiba (In-
tegration of geographic data for the urban planning of
the city of Curitiba). Universidade Tecnol
´
ogica Fed-
eral do Paran
´
a.
Bijsterveld, K. (2008). Mechanical Sound: Technology,
Culture, and Public Problems of Noise in the Twen-
tieth Century. The MIT Press.
Brin, S. and Page, L. (1998). The anatomy of a large-scale
hypertextual web search engine. In Proceedings of the
Seventh International Conference on World Wide Web
7, WWW7, pages 107–117, Amsterdam, The Nether-
lands. Elsevier Science Publishers B. V.
Brown, A. and Lam, K. (1987). Urban noise surveys. Ap-
plied Acoustics, 20(1):23–39.
Calixto, A., Diniz, F. B., and Zannin, P. (2003). The statis-
tical modeling of road traffic noise in an urban setting.
Cities, 20:1–74.
Chen, R., Chu, T., Liu, J., Chen, Y., Chen, L., Xu, W., Li,
X., Hyyppa, J., and Tang, J. (2014). Development
of a contextual thinking engine in mobile devices. In
UPINLBS ’14, pages 90–96.
Duclaux, O., Frejafon, E., Schmidt, H., Thomasson, A.,
Mondelain, D., Yu, J., Guillaumond, C., Puel, C.,
Savoie, F., Ritter, P., Boch, J., and Wolf, J. (2002). 3d-
air quality model evaluation using the lidar technique.
Atmospheric Environment, 36(32):5081 – 5095.
Erwig, M., Guting, R., Schneider, M., and Vazirgiannis, M.
(1999). Spatio-temporal data types: An approach to
modeling and querying moving objects in databases.
GeoInformatica, 3(3):269–296.
Greenwald, A., Hampel, G., Phadke, C., and Poosala, V.
(2011). An economically viable solution to geofenc-
ing for mass-market applications. Bell Lab. Tech. J.,
16(2):21–38.
Hartwig, F. and Dearing, B. (1979). Exploratory Data Anal-
ysis. 07. SAGE Publications.
LNZ Soluc¸
˜
oes em Vibrac¸
˜
oes e Ac
´
ustica (2011). Di-
agn
´
ostico de Ru
´
ıdos Urbanos - Relat
´
orio Final. Tech-
nical report, Prefeitura Municipal de Curitiba - Sec-
retaria Municipal do Meio Ambiente .
Louen, C., Wehrens, A., and Vall
´
ee, D. (2014). Analysis of
the effectiveness of different noise reducing measures
based on individual perception in germany. Trans-
portation Research Procedia, 4:472–481.
Madireddy, M., De Coensel, B., Can, A., Degraeuwe,
B., Beusen, B., De Vlieger, I., and Botteldooren, D.
(2011). Assessment of the impact of speed limit
reduction and traffic signal coordination on vehi-
cle emissions using an integrated approach. Trans-
portation research part D: transport and environment,
16(7):504–508.
Martinez, W., Martinez, A., and Solka, J. (2010). Ex-
ploratory Data Analysis with MATLAB, Second Edi-
tion. Chapman & Hall/CRC Computer Science &
Data Analysis. Taylor & Francis.
Mcdougall, K. (2011). The potential of citizen volunteered
spatial information for building sdi. In GSDI-11 Con-
ference.
Mostashari, A., Arnold, F., Maurer, M., and Wade, J.
(2011). Citizens as sensors: The cognitive city
paradigm. In CEWIT ’11, pages 1–5.
Moura, T. H. V. M. and Davis Jr., C. A. (2013). Linked
geospatial data: desafios e oportunidades de pesquisa.
In XIV Brazilian Symposium on GeoInformatics.,
pages 13–18. SBC.
NIST/SEMATECH (2012). E-Handbook of Statistical
Methods, available at http://www.itl.nist.gov/div898/
handbook/.
Oliveira, M. P. G., Medeiros, E. B., and Davis, Jr., C. A.
(1999). Planning the Acoustic Urban Environment: A
GIS-centered Approach. In GIS ’99, pages 128–133,
New York, NY, USA. ACM.
Ravada, S., Ali, M., Bao, J., and Sarwat, M. (2013). Acm
sigspatial gis cup 2013: Geo-fencing. In SIGSPA-
TIAL’13, pages 584–587, New York, NY, USA. ACM.
Rodrigues, F. (2010). Metodologia para investigac¸
˜
ao de
relac¸
˜
ao entre ru
´
ıdo de tr
´
afego e condic¸
˜
oes opera-
cionais do fluxo em centros . PhD thesis, Instituto
Alberto Luiz Coimbra de P
´
os-Graduac¸
˜
ao e Pesquisa
de Engenharia - UFRJ.
Rodriguez Garzon, S. and Deva, B. (2014). Geofencing
2.0: Taking location-based notifications to the next
level. In UbiComp ’14, pages 921–932, New York,
NY, USA. ACM.
Ryoo, J., Kim, H., and Das, S. (2012). Geo-fencing:
Geographical-fencing based energy-aware proactive
framework for mobile devices. In Quality of Service
(IWQoS), 2012 IEEE 20th International Workshop on,
pages 1–9.
Schweizer, I., Darmstadt, T., Probst, F., B
¨
artl, R., Darm-
stadt, T., M
¨
uhlh
¨
auser, M., Darmstadt, T., Schulz, A.,
and Darmstadt, T. (2011). Noisemap - real-time par-
ticipatory noise maps. In PhoneSense’2011.
Sheth, A., Seshan, S., and Wetherall, D. (2009). Geo-
fencing: Confining wi-fi coverage to physical bound-
aries. In Pervasive ’09, pages 274–290. Springer-
Verlag.
Verheijen, E. and Jabben, J. (2010). Effect of electric cars
on traffic noise and safety. Bilthoven: RIVM.
Zannin, P. H. T., Calixto, A., Diniz, F. B., and C., J. A.
(2003). A survey of urban noise annoyance in a large
brazilian city: the importance of a subjective analysis
in conjunction with an objective Environmental Im-
pact Assessment Review, 23:245–255.
Zannin, P. H. T., Diniz, F. B., and Barbosa, W. A. (2002).
Environmental noise pollution in the city of Curitiba,
Brazil . Applied Acoustics, 63(4):351 – 358.
Analyzing the Acoustic Urban Environment - A Geofencing-centered Approach in the Curitiba Metropolitan Region, Brazil
85