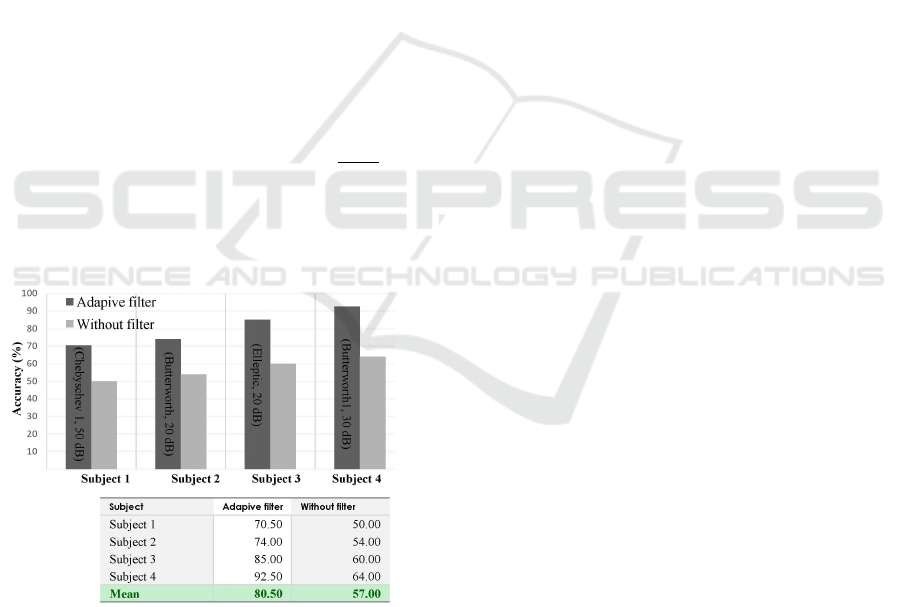
and the other one for testing. As depicted in Figure
6, the accuracy of the two class motor imagery ac-
tions increases when we integrate the adaptive filter-
ing techniques for all subjects. In fact, the proposed
BCI chain is able to discriminate RH and LH EEG
signals with a high accuracy reaching 92% for subject
4 for example. The mean performance of all subjects
is close to 80% using adaptive filtering techniques.
Figure 6 shows clearly a significant improvement in
the system accuracy by just tuning the filters parame-
ters. The adaptive filter is done automatically during
the training phase for each subject and the best param-
eters were fixed during the test phase. Furthermore,
the enhancement of classification accuracy over tra-
ditional classifiers based on one fixed filter is signifi-
cant and can reach u;p 25%. The filters parameters are
heterogeneous among subject. For example for sub-
ject one the best filters is Chebyschev1 with an SNR
of 50 dB, that implies motor imagery frequencies of
this subject are inside µ and β bands. In others cases
for example, for subject two the LH and RH frequen-
cies are outside these theoretical bands. Information
Transfer Rate (ITR) is used to evaluate the proposed
system according to the following equation:
IT R = L[plog
2
(p) + log
2
(N) + (1 − p)log
2
(
1 − p
N −1
)] (8)
Let consider L is the number of decisions in one
minute, and p is the accuracy of the subject in making
decisions among N targets.
Figure 6: Classification accuracy for four subjects.
The ITR for all subjects is 8.64 bits/min which
is very interesting result compared to a similar work.
For example, in (Sannelli et al., 2010), the ITR com-
puted for three subjects is 3.56 bits/min. The system
proposed in (McFarland et al., 2003) dedicated for
controlling the mouse using two motor imagery ac-
tions reaches 7.4 bits/min as ITR for eight subjects.
For comparison purpose with the offline approach,
the same algorithms are used in the off-line context
and a small increase of only 1% of accuracy. Our re-
sults show that adapting filtering is very interesting to
be integrated into the on-line validation of the EEG-
based motor imagery application to reach a high ac-
curacy.
5 CONCLUSION
The proposed EEG chain shows a clear improvement
of the performance of the system leading to good dis-
crimination between LH and RH motor imagery tasks
for online validation. In order to improve the per-
formance of the proposed system, adaptive filter was
used to remove the maximum of the unwanted sig-
nals, and to tune the pass band which contain µ and β
band. The proposed method shows that the combina-
tion of the adaptive filter with CSP as features extrac-
tion and LDA as classifier improves the classification
accuracy using the online approach. Furthermore, the
ITR of the system is very interesting comparing with
those obtained from equivalent existing systems.
Our future work target is to extend the proposed
procedure to a multiclass paradigm in order to classify
three tasks that will be used with a state machine to
control home devices equipment. In addition, it will
be interesting to implement the proposed procedure in
real time embedded system.
ACKNOWLEDGEMENTS
This project was funded by the National Plan
for Science, Technology and Innovation (MAARI-
FAH), King Abdulaziz City for Science and Tech-
nology, Kingdom of Saudi Arabia, Award Number
(ELE1730).
REFERENCES
Belwafi, K., Djemal, R., Ghaffari, F., and Romain, O.
(2014). An adaptive EEG filtering approach to max-
imize the classification accuracy in motor imagery.
In Computational Intelligence, Cognitive Algorithms,
Mind, and Brain (CCMB), 2014 IEEE Symposium on,
pages 121–126.
Cai, B., Xiao, S., Jiang, L., Wang, Y., and Zheng, X. (2013).
A rapid face recognition bci system using single-trial
erp. In Neural Engineering (NER), 2013 6th Interna-
tional IEEE/EMBS Conference on, pages 89–92.
Duan, S., Xu, T., Zhuang, W., and Mao, D. (2014). The fea-
ture extraction of erd/ers signals based on the wavelet
Smart-BIODEV 2016 - Special Session on Smart Embedded Biomedical Devices for In Situ Physiological Signal Processing
338