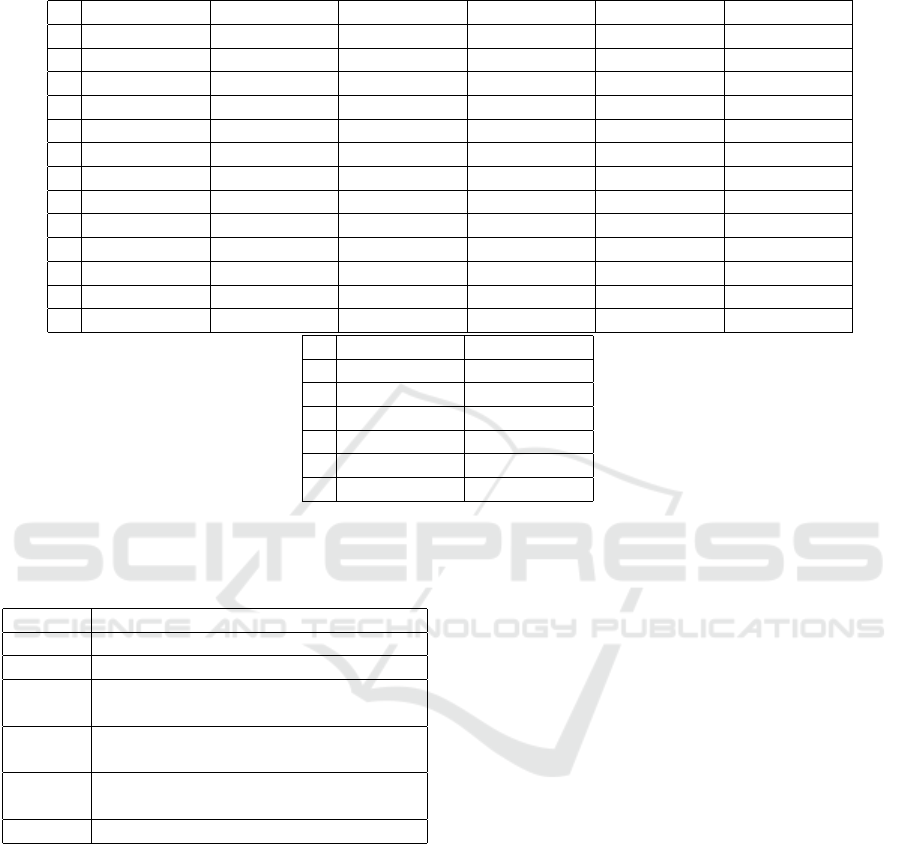
Table 2: Table 2 of MSE values of Sunsal classifier, where A=Argriculture, B=Water,C=Eucalyptus,D=Open Savannah,
E=Closed Savannah, F=Forest, G=Seasonal Forest, H=Ciliar Vegetation I= Open Savanah+ciliar vegetation, J=Open Sa-
vanah+Forest type 1, K= Open Savanah+Forest type 2, L= Savannah+ Seasonal Forest, M=Pasture, N=Urban.
A B C D E F
1 0,013702123 0,014358648 0,00394132 0,014724775 0,045046558 0,072690414
2 0,043921584 0,022896723 0,031124566 0,002306937 0,007234252 0,019553144
3 0,020523329 0,016096906 0,010706222 0,007059779 0,026975737 0,049272183
4 0,01338676 0,017668488 0,002655275 0,029399763 0,072473686 0,105373549
5 0,076233369 0,042593683 0,060901952 0,009707884 0,001062428 0,005719512
6 0,110176038 0,065631921 0,09301972 0,023370714 0,004278307 0,001799481
G H I J K L
1 0,056415746 0,011683206 0,006053524 0,052763398 0,005628253 0,003442471
2 0,01302728 0,001776301 0,006218013 0,010195047 0,012103119 0,008181732
3 0,037179256 0,00419623 0,002892844 0,033783286 0,003984626 0,00177814
4 0,084818225 0,027074711 0,015296688 0,081290543 0,011946412 0,011910625
5 0,005061559 0,010941475 0,021002377 0,001024141 0,031136352 0,025381872
6 0,005089482 0,026979652 0,041094464 0,001690714 0,05498827 0,047385143
M N
1 0,008173389 0,017284448
2 0,004114109 0,066694088
3 0,002740651 0,032219417
4 0,020860182 0,005923803
5 0,015761101 0,108046735
6 0,033708819 0,149705802
Table 3: New classes elaborated after conducting our ap-
proach.
Clusters Ground truth classes
1 Water (B)
2 OpenSav and CiliarVeg (D+H)
3 OpenSav+CiliarVeg, Forest+OpenSav
Type 2 and Sav+SeasonForest (I+K+L)
4 Agriculture, Eucalyptus and Urban
(A,C,N)
5 OpenSav+Forest Type 1 and ClosedSav
and SeasonForest (J+E+G)
6 Forest (F)
such as Agriculture. This deduction comes from the
fact that its MSE is relatively high (0.013) and this can
be explained by the heterogeneity of its class: it rep-
resents three behavior’s types as illutrated in Figure 7
and (Jonathan et al., 2005).
6 CONCLUSION
Thanks to coarse data, we conducted a clustering pro-
cess and generated ”labeled” dictionary. We explored
the sparse representation and its capabilities to fit the
data, to reduce dimensions in order to improve clus-
tering results. We compared two classifiers : OMP
and SunSAL and found that SunSAL outperforms
OMP. But here some critics must be mentioned : al-
though MODIS images provide coverage at continen-
tal and global scales, the ability to reveal specific de-
tails of the region study remains difficult. Many of
pixels generated by coarse resolution sensors are not
characteristic of only one vegetation but represent a
mixture as we mentioned in the experimentation sec-
tion. Therefore, for this study, we consider that the
pixel refers to one type of vegetation. So, in perspec-
tives, we aim to accurate our results by finding a way
to tradeoff multi-sensor data in order to enhance class
discrimination to monitor land cover change. This
would be done by the mean of merging multi-source
images with different spatial, spectral and temporal
resolutions.
RGB-SpectralImaging 2016 - Special Session on RBG and Spectral Imaging for Civil/Survey Engineering, Cultural, Environmental,
Industrial Applications
702