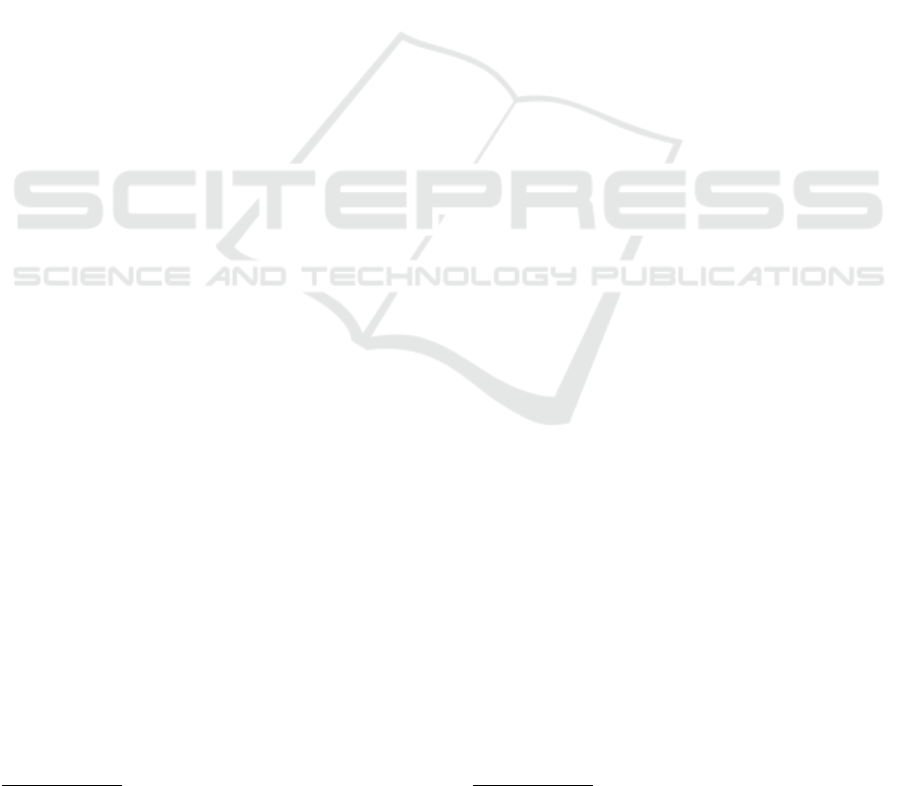
are four situations that enable cross-domain recom-
mendations: a) no overlap between items and users,
b) user sets of different domains overlap, c) item sets
overlap, and d) item and user sets overlap.
In this work, we investigate whether the source
domain improves the recommendation performance
in the target domain on system level in the situation
when only items overlap. The idea behind the paper
is as follows. Traditional cross-domain recommender
systems utilize overlapping users to discover addi-
tional interests of users, leading to the improvement
of the recommendation diversity. When the items
overlap, the source domain lets detect more accurate
similarities between items, which should positively
result in recommendation performance in the target
domain.
Due to the lack of publicly available datasets for
cross-domain recommender systems with overlapping
items (Berkovsky et al., 2008; Kille et al., 2013), we
collected data from Vkontakte
3
(VK) – Russian on-
line social network (OSN) and Last.fm
4
(FM) – mu-
sic recommender service. We then matched VK and
FM audio recordings and developed the cross-domain
recommender system that suggests VK recordings to
VK users based on data from both domains. Each au-
dio recording is represented by its metadata excluding
the actual audio file. VK recordings thus represent
the target domain, while the source domain consists
of FM recordings. VK and FM recordings share titles
and artists, but have different user ratings and other
attributes.
In order to address the research question and illus-
trate the potential of additional data, we chose sim-
ple but popular recommendation algorithms to con-
duct experiments for validation: collaborative filter-
ing based on users’ ratings and content-based filtering
based on the descriptions of the items.
Our results indicate that the source domain can
improve the recommendation performance in the tar-
get domain. Furthermore, with the growth of non-
overlapping items in different domains, the improve-
ment of recommendation performance decreases.
This paper thus has the following contributions:
• we initially investigate the cross-domain recom-
mendation problem in the situation when only
items overlap;
• we collect a novel dataset to conduct the experi-
ments for addressing the research question.
The paper might be useful in real life scenarios.
For example, according to our results, the perfor-
mance of a recommender system lacking user rat-
3
http://vk.com/
4
http://last.fm/
ings to achieve an acceptable performance can be im-
proved using ratings collected from another recom-
mender system that suggests items of the same type.
However, the performance might decrease if the rec-
ommender systems do not have enough overlapping
items.
The rest of the paper is organized as follows. Sec-
tion 2 overviews related works. Section 3 describes
the datasets used to conduct experiments. Section 4 is
dedicated to recommendation approaches, while sec-
tion 5 describes conducted experiments. Finally, sec-
tion 6 draws final concussions.
2 RELATED WORKS
Most existing approaches consider additional infor-
mation about users to boost the recommendation per-
formance. For example, one of the first studies dedi-
cated to cross-domain recommender systems investi-
gated the effectiveness of source domains with over-
lapping users (Winoto and Tang, 2008). In the exper-
iment, undergraduates from a local university were
asked to rate items from different domains, such as
movies, songs and books. The authors measured rec-
ommendation performance in different domain com-
binations and concluded that source domains decrease
the recommendation performance, but may improve
the diversity of recommendations.
In contrast, other studies demonstrated that source
domains can boost the recommendation performance
in the target domain in situations when users or both
users and items overlap. For example, Sang demon-
strated the feasibility of utilizing the source domain.
The study was conducted on a dataset collected from
Twitter
5
and YouTube
6
. The author established rela-
tionships between items from different domains using
topics (Sang, 2014). Similarly to Sang, Shapira et al.
also linked items from different domains, where 95
participants rated movies and allowed the researches
to collect data from their Facebook pages. The re-
sults suggested that additional domains improve the
recommendation performance (Shapira et al., 2013).
Another study with positive results was conducted by
Abel et al. The dataset contained information related
to the same users from 7 different OSNs (Abel et al.,
2013). Sahebi et al. demonstrated the usefulness
of recommendations based on additional domains to
overcome cold start problem (Sahebi and Brusilovsky,
2013).
Most works on cross-domain recommender sys-
tems focus on the situation when users or both users
5
https://twitter.com/
6
https://www.youtube.com/
WEBIST 2016 - 12th International Conference on Web Information Systems and Technologies
132