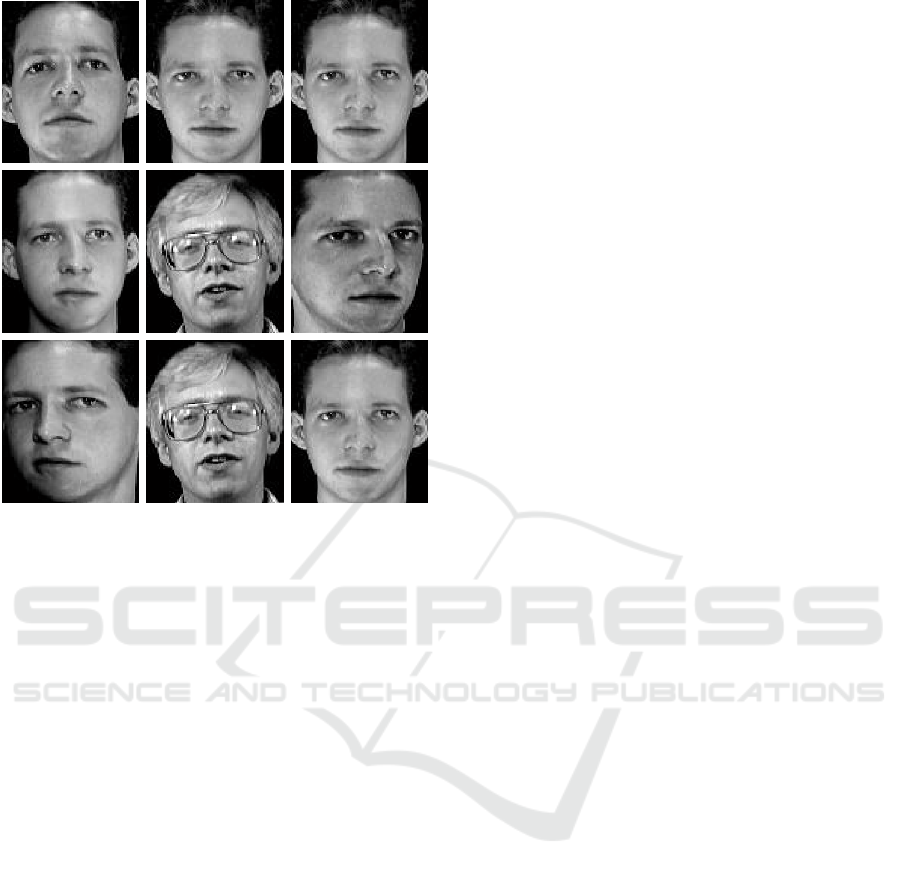
(a) (b) (c)
Figure 6: Examples of face recognition results for (a) given
test images; (b) using state-of-art method; (c) our method.
pose correction, and face recognition. Hence, the face
detection phase uses the Viola & Jones method, while
the pose correction phase relies on our new, pose-
correction algorithm fed by interactively selected fa-
cial features, such nose tip and nose top, in the de-
tected face. The face recognition phase applies the
eigenface approach on the pose-corrected face. Our
iFR system combines the advantages of both template
and feature approaches for face recognition. It outper-
forms the state-of-the-art methods, and is well suited
system for online applications involving face recogni-
tion.
REFERENCES
Ahonen, T., Hadid, A., and Pietikainen, M. (2006). Face
description with local binary patterns: Application to
face recognition. IEEE Transactions on Pattern Anal-
ysis and Machine Intelligence, 28(12):2037–2041.
Bordoloi, H. and Sarma, K. K. (2009). Face recognition
with image rotation detection, correction and rein-
forced decision using ANN. International Journal
of Electrical and Electronics Engineering, 3(7):1205–
1212.
Brunelli, R. and Poggio, T. (1993). Face recognition: fea-
tures versus templates. IEEE Transactions on Pat-
tern Analysis and Machine Intelligence, 15(10):1042–
1052.
Choi, J. Y., Neve, W. D., Plataniotis, K. N., and Ro, Y. M.
(2011). Collaborative face recognition for improved
face annotation in personal photo collections shared
on online social networks. IEEE Transactions on Mul-
timedia, 13(1):14–18.
Cootes, T. F., Edwards, G. J., and Taylor, C. J. (2001). Ac-
tive appearance model. IEEE Transactions on Pattern
Analysis and Machine Intelligence, 23(6):681–685.
Du, S. and Ward, R. (2006). Face recognition under
pose variations. Journal of the Franklin Institute,
343(6):596–613.
Gallego-Jutgla, E., de Ipina, K. L., Marti-Puig, P., and Sole-
Casals, J. (2013). Empirical mode decomposition-
based face recognition system. In Proceedings of the
International Conference on Bio-Inspired Systems and
Signal Processing, pages 445–450.
Gross, R. (2005). Face Databases, chapter Handbook of
Face Recognition, pages 301–327. Springer-Verlag.
Han, M.-J., Lin, C.-H., and Song, K.-T. (2013). Robotic
emotional expression generation based on mood tran-
sistion and personality model. IEEE Transactions on
Cybernetics, 43(4):1290–1303.
Huang, G. B., Mattat, M. A., Lee, H., and Learned-Miller,
E. (2012). Learning to align from scratch. In Proceed-
ings of the Conference on Advances in Neural Infor-
mation Processing Systems, pages 764–772.
Jain, A. K., Klare, B., and Park, U. (2012). Face matching
and retrieval in Forensics applications. IEEE Multi-
Media, 19(1):20.
Lindner, C., Bromiley, P. A., Ionita, M. C., and Cootes, T. F.
(2015). Robust and accurate shape model matching
using random forest regression voting. IEEE Trans-
actions on Pattern Analysis and Machine Intelligence,
37(9):1862–1874.
Montagne, C., Kodewitz, A., Vigneron, V., Giraud, V., and
Lelandais, S. (2013). 3D local binary pattern for PET
image classification by SVM. In Proceedings of the
International Conference on Bio-Inspired Systems and
Signal Processing, pages 145–4150.
Murphy-Chutorian, E. and Trivedi, M. M. (2009). Head
pose estimation in computer vision: A survey. IEEE
Transactions on Pattern Analysis and Machine Intel-
ligence, 31(4):607–626.
Nagi, J., Ahmed, S. K., and Nagi, F. (2008). A Matlab-
based face recognition system using image processing
and neural networks. In Proceedings of the Interna-
tional Colloquium on Signal Processing and its Ap-
plications, pages 83–88.
Nakamura, K. and Takano, H. (2011). New Approaces
to Characterization and Recognition of Faces, chap-
ter Face discimination using the orientation and size
recognition characteristics of the spreading associa-
tive neural network, pages 197–212. InTech.
Olszewska, J. I. (2013). Multi-scale, multi-feature vector
flow active contours for automatic multiple-face de-
tection. In Proceedings of the International Confer-
ence on Bio-Inspired Systems and Signal Processing,
pages 429–435.
Rambach, J., Huber, M. F., Balthasar, M. R., and Zoubir,
A. M. (2015). Collaborative multi-camera face recog-
iFR: Interactively Pose Corrected Face Recognition
111