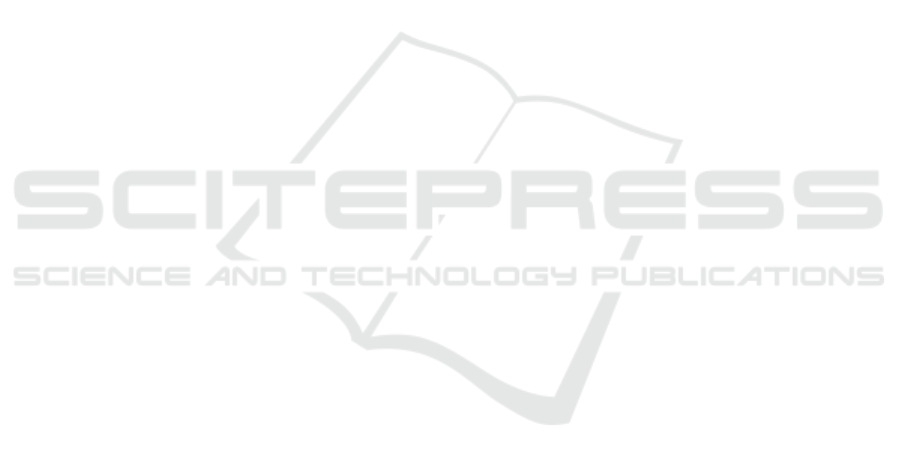
8.2 Impact of Flexibility
To understand the impact of flexibility, a similar situa-
tion with non flexible operator in one case and flexible
operators on the other must be compared. Here study
has been mainly focused with respect to two parame-
ters namely energy consumption and production and
utility variation. A randomly sampled result has been
put to display.
The plots in Figure 2 show the need of flexibility
in the hours of high demand. It is justified since it is
not wise to trouble the customers all the time as that
might lead to undesirable circumstances. For exam-
ple, in the 11
th
hour, there was an imminent need for
flexibility after the local gas turbine reached its maxi-
mum operating point. The plots in Figure 3 show the
variation of utility/profit in both cases. It can be ob-
served that at the time of flexibility, the non flexible
operator incurred a loss while the flexible one made a
profit, no matter how meager it is. From an econom-
ical standpoint, flexibility resulted in a better utility
than being non flexible keeping the other parameters
constant.
9 CONCLUSION
In this paper, though we have kept the mathematical
modeling simple, the impact of flexibility at aggrega-
tor level have been quite prominent. The naive way of
finding an optimal tariff, seemed effective in showing
flexibility. More importantly, the system showed flex-
ibility only in times of high demand, automatically
modeling the comfort level of the consumers. Find-
ing out the optimal tariff for the system is worth re-
searching as it is one of the critical parameter for the
system to show flexibility. Instead of keeping it con-
stant throughout the day, it can made to vary along
different hours of the day. There is lack of strategies
in the paper, by virtue of which a scheduling operator
can model others. Possible scopes of experimenting
lies in formulation of the flexibility function and com-
pensation function for the aggregator. The interaction
between the scheduling operators via the market can
be thought of as an auction mechanism. Herein lies
the future scope of game theory into modeling the ex-
pectation function for the scheduling operators along
with bidding strategies. With more than two schedul-
ing operators in the market, the grid dynamics will be
interesting to observe.
REFERENCES
Albadi, M. H. and El-Saadany, E. (2007). Demand response
in electricity markets: An overview. In IEEE power
engineering society general meeting, volume 2007,
pages 1–5.
Chen, C., Kishore, S., and Snyder, L. V. (2011). An innova-
tive RTP-based residential power scheduling scheme
for smart grids. In IEEE International Conference on
Acoustics, Speech and Signal Processing (ICASSP),
pages 5956–5959.
Fadlullah, Z. M., Nozaki, Y., Takeuchi, A., and Kato, N.
(2011). A survey of game theoretic approaches in
smart grid. In IEEE International Conference on Wire-
less Communications and Signal Processing (WCSP),
pages 1–4.
Gkatzikis, L., Koutsopoulos, I., and Salonidis, T. (2013).
The role of aggregators in smart grid demand response
markets. IEEE Journal on Selected Areas in Commu-
nications, 31(7):1247–1257.
Maity, I. and Rao, S. (2010). Simulation and pricing mecha-
nism analysis of a solar-powered electrical microgrid.
Systems Journal, 4(3):275–284.
Mohsenian-Rad, A.-H., Wong, V. W., Jatskevich, J., and
Schober, R. (2010a). Optimal and autonomous
incentive-based energy consumption scheduling algo-
rithm for smart grid. In Innovative Smart Grid Tech-
nologies (ISGT), 2010, pages 1–6. IEEE.
Mohsenian-Rad, A.-H., Wong, V. W., Jatskevich, J.,
Schober, R., and Leon-Garcia, A. (2010b). Au-
tonomous demand-side management based on game-
theoretic energy consumption scheduling for the fu-
ture smart grid. IEEE Transactions on Smart Grid,
1(3):320–331.
Momoh, J. et al. (2009). Smart grid design for efficient
and flexible power networks operation and control. In
IEEE/PES Power Systems Conference and Exposition
(PSCE), pages 1–8.
Nguyen, P. H., Kling, W. L., and Ribeiro, P. F. (2013). A
game theory strategy to integrate distributed agent-
based functions in smart grids. IEEE Transactions on
Smart Grid, 4(1):568–576.
Roche, R. (2012). Algorithmes et architectures multi-
agents pour la gestion de l’
´
energie dans les r
´
eseaux
´
electriques intelligents. Application aux centrales
`
a
turbines
`
a gaz et
`
a l’effacement diffus r
´
esidentiel.
PhD thesis, Universit
´
e de Technologie de Belfort-
Montb
´
eliard, Institut de Recherche sur les Trans-
ports, l’Energie et la Soci
´
et
´
e / Laboratoire Syst
`
emes
et Transports.
Saad, W., Han, Z., Poor, H. V., and Bas¸ar, T. (2012). Game-
theoretic methods for the smart grid: An overview
of microgrid systems, demand-side management, and
smart grid communications. Signal Processing Mag-
azine, IEEE, 29(5):86–105.
SMARTGREENS 2016 - 5th International Conference on Smart Cities and Green ICT Systems
356