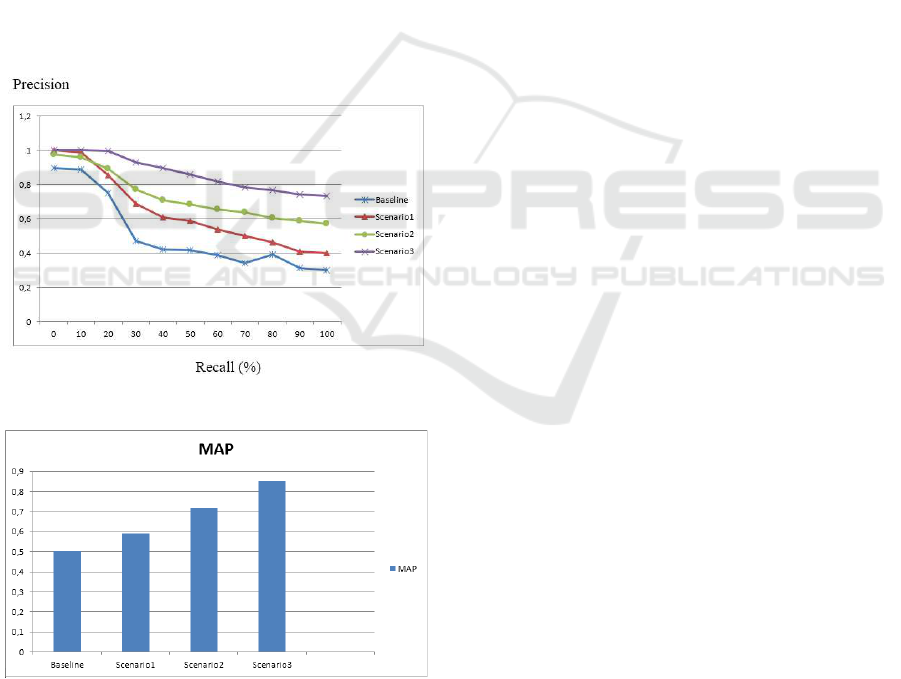
precision of the 10, 20 and 30 first documents re-
turned, the NDCG (Normalized Cumulative Gain)
and MAP (Mean Average Precision) of all 40 selected
queries. For each query, the first 100 records are re-
turned by the experimental SRI Lemur and average
precisions are calculated to measure the relevance of
the system. The presented results compare precision,
MAP and NDCG values between the personalized ap-
proach and the baseline. We notice that a precision at
5 of a personalized search is better than the baseline.
The sheet at (cf. Figure 3). presents the search re-
sults quality measurement with virtual communities.
We calculate P@5, P@10, and MAP(cf. Figure 4)
for a set of 40 queries. We can see in this sheet that
P@10 presents more accurate results. Moreover, we
see that performance improvement is better for P@10
or P@15 than P@30 and P@100. This improvement
can be explained by the fact that there is less irrele-
vance when we consider the first 10 or 15 results. We
can also conclude that the personalized search includ-
ing virtual communities improves system precision.
Figure 3: Recall/Precision Curve.
Figure 4: MAP histogram.
4 CONCLUSIONS
We began this paper with an overview of personaliza-
tion. Then we presented our proposal of using vir-
tual communities to model users for information re-
trieval. The proposed approach includes the creation
of user profiles, the construction of virtual commu-
nities and influence networks. We conducted an ex-
perimentation and evaluation phase on INEX Book
Social Search Dataset (Koolen et al., 2012). Evalu-
ation shows that the system improves search results
when integrating virtual communities and influence
networks. However, these results show some limita-
tions. In fact, the process of user profile construction
and that of virtual communities construction are not
synchronized. The two processes cannot take place
simultaneously. Profile construction is always done
in batch mode in the case of a cold start.
REFERENCES
Cheung, D. W., Kao, B., and Lee, J. (1998). Discov-
ering user access patterns on the world wide web.
Knowledge-Based Systems, 10(7):463–470.
Esparza, S. G., OMahony, M. P., and Smyth, B. (2012).
Mining the real-time web: a novel approach to product
recommendation. Knowledge-Based Systems, 29:3–
11.
Koolen, M., Kazai, G., Kamps, J., Doucet, A., and Lan-
doni, M. (2012). Overview of the INEX 2011 Books
and Social Search Track. In Geva, S., Kamps, J., and
Schenkel, R., editors, Focused Retrieval of Content
and Structure : 10th International Workshop of the
Initiative for the Evaluation of XML Retrieval, INEX
2011, volume 7424 of Lecture Notes in Computer Sci-
ence, pages 1–29. Springer.
Maleszka, B. (2015). An adaptation method for hierarchi-
cal user profile in personalized document retrieval sys-
tems. In Intelligent Information and Database Sys-
tems, pages 107–116. Springer.
Micarelli, A., Gasparetti, F., Sciarrone, F., and Gauch, S.
(2007). Personalized search on the world wide web.
In The adaptive web, pages 195–230. Springer.
Min, J. and Jones, G. J. (2011). Building user interest pro-
files from wikipedia clusters.
Newman, M. (2004). Detecting community structure in net-
works. European Physical Journal, 38:321–330.
Tanudjaja, F. and Mui, L. (2002). Persona: A contextu-
alized and personalized web search. In System Sci-
ences, 2002. HICSS. Proceedings of the 35th Annual
Hawaii International Conference on, pages 1232–
1240. IEEE.
Treur, J. and Umair, M. (2011). An agent model integrat-
ing an adaptive model for environmental dynamics.
International Journal of Intelligent Information and
Database Systems, 5(3):201–228.
van Rijsbergen, K. (2013). The roots of the theoretical ba-
sis for information retrieval. In International Confer-
ence on the Theory of Information Retrieval, ICTIR
Utilizing Virtual Communities for Information Retrieval and User Modeling
33