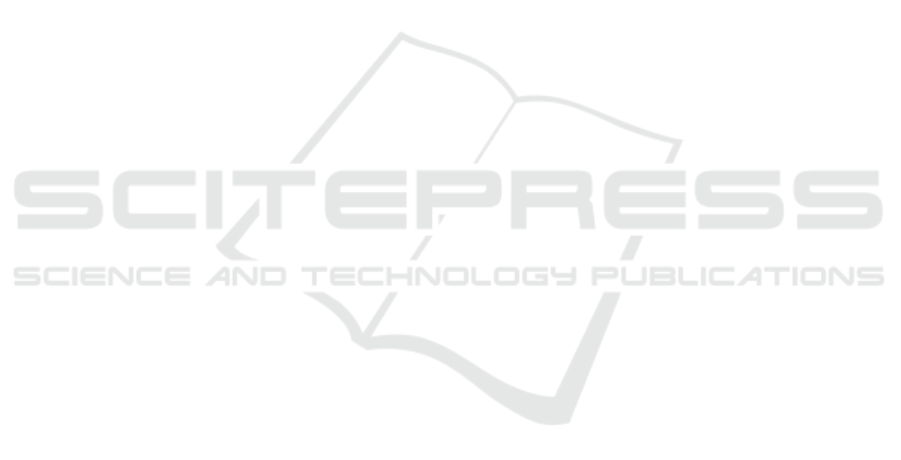
on Very Large Data Bases (VLDB), pages 1083–1086.
VLDB Endowment.
Adomavicius, G. and Tuzhilin, A. (2005). Toward the
next generation of recommender systems: A survey
of the state-of-the-art and possible extensions. IEEE
Transactions on Knowledge and Data Engineering,
17(6):734–749.
Adomavicius, G. and Tuzhilin, A. (2011). Context-aware
recommender systems. In Ricci, F., Rokach, L.,
Shapira, B., and Kantor, P. B., editors, Recommender
Systems Handbook, pages 217–253. Springer.
Agrawal, S., Chaudhuri, S., and Das, G. (2002). DBX-
plorer: A system for keyword-based search over re-
lational databases. In 18th International Conference
on Data Engineering (ICDE), pages 5–16. IEEE.
Baltrunas, L., Church, K., Karatzoglou, A., and Oliver, N.
(2015). Frappe: Understanding the usage and per-
ception of mobile app recommendations in-the-wild.
CoRR, abs/1505.03014.
Baltrunas, L., Kaminskas, M., Ludwig, B., Moling, O.,
Ricci, F., Aydin, A., L
¨
uke, K.-H., and Schwaiger, R.
(2011). InCarMusic: Context-aware music recom-
mendations in a car. In EC-Web, volume 11, pages
89–100. Springer.
Bergamaschi, S., Domnori, E., Guerra, F., Orsini, M., Lado,
R. T., and Velegrakis, Y. (2010). Keymantic: Semantic
keyword-based searching in data integration systems.
Proceedings of the VLDB Endowment, 3(1–2):1637–
1640.
Bergamaschi, S., Guerra, F., Interlandi, M., Trillo-Lado, R.,
and Velegrakis, Y. (2013). QUEST: A keyword search
system for relational data based on semantic and ma-
chine learning techniques. Proceedings of the VLDB
Endowment, 6(12):1222–1225.
Bergamaschi, S., Guerra, F., Rota, S., and Velegrakis,
Y. (2011). A Hidden Markov Model approach to
keyword-based search over relational databases. In
Jeusfeld, M., Delcambre, L., and Ling, T.-W., editors,
Conceptual Modeling–ER 2011, volume 6998, pages
411–420. Springer.
Chakrabarti, S., Sarawagi, S., and Sudarshan, S. (2010). En-
hancing search with structure. IEEE Data Engineer-
ing Bulletin, 33(1):3–24.
del Carmen Rodr
´
ıguez-Hern
´
andez, M. and Ilarri, S. (2015).
Pull-based recommendations in mobile environments.
Computer Standards & Interfaces.
Forney, J. G. D. (1973). The Viterbi algorithm. Proceedings
of the IEEE, 61(3):268–278.
Hristidis, V. and Papakonstantinou, Y. (2002). DISCOVER:
Keyword search in relational databases. In 28th In-
ternational Conference on Very Large Data Bases
(VLDB), pages 670–681. VLDB Endowment.
Jannach, D., Zanker, M., Felfernig, A., and Friedrich, G.
(2010). Recommender Systems: An Introduction.
Cambridge University Press, first edition.
Kantor, P. B., Rokach, L., Ricci, F., and Shapira, B. (2011).
Recommender Systems Handbook. Springer, New
York, USA.
Ko
ˇ
sir, A., Odic, A., Kunaver, M., Tkalcic, M., and Tasic,
J. F. (2011). Database for contextual personalization.
Elektrotehni
ˇ
ski vestnik, 78(5):270–274.
Levandoski, J. J., Sarwat, M., Eldawy, A., and Mokbel,
M. F. (2012). LARS: A location-aware recommender
system. In 28th International Conference on Data En-
gineering (ICDE), pages 450–461. IEEE.
Li, G., Ooi, B. C., Feng, J., Wang, J., and Zhou, L.
(2008). EASE: An effective 3-in-1 keyword search
method for unstructured, semi-structured and struc-
tured data. In 2008 ACM SIGMOD International Con-
ference on Management of Data (SIGMOD), pages
903–914. ACM.
Lou, H.-L. (1995). Implementing the Viterbi algorithm.
IEEE Signal Processing Magazine, 12(5):42–52.
Rabiner, L. R. (1989). A tutorial on Hidden Markov Models
and selected applications in speech recognition. Pro-
ceedings of the IEEE, 77(2):257–286.
Salton, G. and McGill, M. J. (1986). Introduction to Mod-
ern Information Retrieval. McGraw-Hill, Inc., New
York, NY, USA.
Shapira, B. and Zabar, B. (2011). Personalized search: In-
tegrating collaboration and social networks. Journal
of the American Society for Information Science and
Technology, 62(1):146–160.
Singam, J. A. and Srinivasan, S. (2015). Optimal keyword
search for recommender system in Big Data applica-
tion. ARPN Journal of Engineering and Applied Sci-
ences (ARPN-JEAS), 10(7):3243–3247.
Stanescu, A., Nagar, S., and Caragea, D. (2013). A hybrid
recommender system: User profiling from keywords
and ratings. In 2013 IEEE/WIC/ACM International
Joint Conferences on Web Intelligence (WI) and In-
telligent Agent Technologies (IAT), volume 1, pages
73–80. IEEE.
Teorey, T. J., Yang, D., and Fry, J. P. (1986). A logical
design methodology for relational databases using the
extended entity-relationship model. ACM Computing
Surveys, 18(2):197–222.
Vargas-Govea, B., Gonz
´
alez-Serna, G., and Ponce-
Medellın, R. (2011). Effects of relevant contextual
features in the performance of a restaurant recom-
mender system. In Fifth ACM Conference on Rec-
ommender Systems (RecSys): Third Workshop on
Context-Aware Recommender Systems (CARS), vol-
ume 791. CEUR.
Ziegler, C.-N., McNee, S. M., Konstan, J. A., and Lausen,
G. (2005). Improving recommendation lists through
topic diversification. In 14th International Conference
on World Wide Web (WWW), pages 22–32. ACM.
Zobel, J. and Moffat, A. (2006). Inverted files for text search
engines. ACM Computing Surveys (CSUR), 38(2):6.
ICEIS 2016 - 18th International Conference on Enterprise Information Systems
214