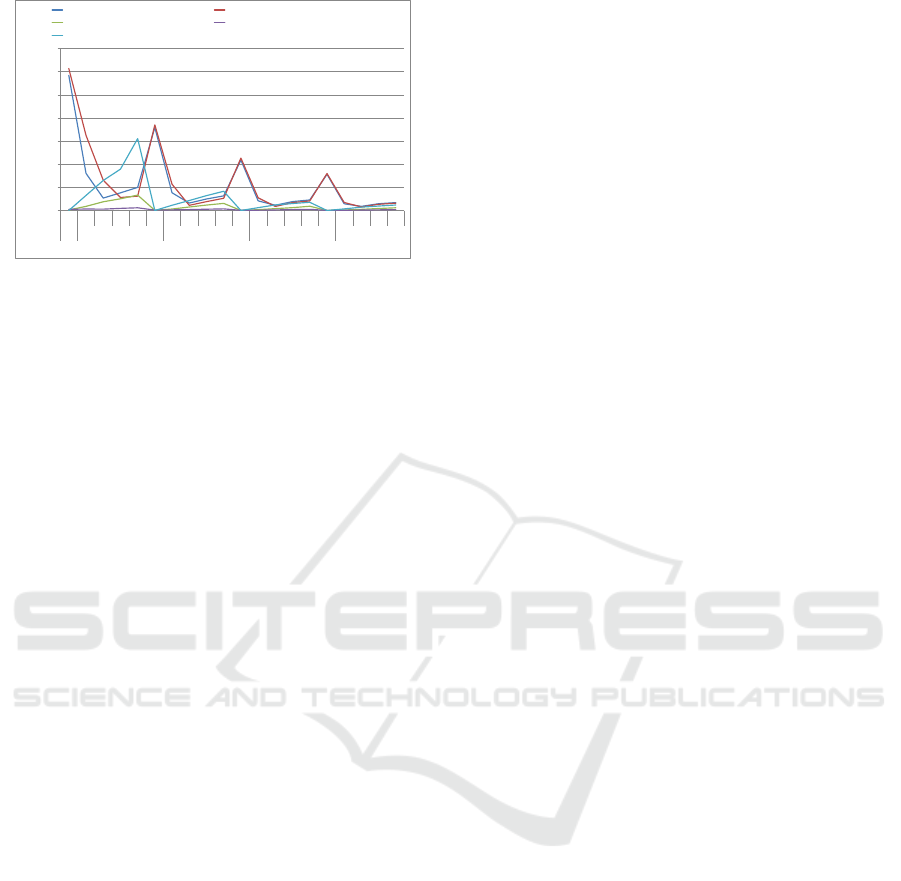
0.000
0.050
0.100
0.150
0.200
0.250
0.300
0.350
Beta 0.0 0.5 1.0 1.5 2.0 0.0 0.5 1.0 1.5 2.0 0.0 0.5 1.0 1.5 2.0 0.0 0.5 1.0 1.5
n 3 7 11 15
Error
Experimental Parameters
Original FEA Wang Normalization Modification
FEA with Centroid Defuzzification FEA while utilising only Mid Number
Eigen Vector Approach using Crisp Matrix
Figure 4: Experimental Analysis.
decreased and hence the final error term is also low
which depicts improvement in performance. There-
fore, this improved performance cannot be associated
with any of the FAHP algorithm.
As we increase the inconsistency factor, perfor-
mance of most of the algorithms is decreased except
for original FEA method and FEA method with mod-
ified normalization for which performance increases
as we increase the inconsistency. However, this in-
crease in performance is not enough and even at high
inconsistency levels, FEA with defuzzification using
mid number is the best performing algorithm.
5 CONCLUSIONS
In this paper, we introduced a novel experimental
analysis framework through which performance of
various FAHP technique can be analyzed. The analy-
sis revealed that the FEA method with defuzzification
using mid number outperformed the other techniques
in almost all experimental conditions.
Review of the existing literature on FAHP reveal
that there are many different algorithms proposed in
this domain. However, there is no throughout analysis
of these techniques which measure their performance
for different experimental conditions. Such a com-
parison would be invaluable for the researchers and
the practitioners of the field since it will hint which
technique might be more suitable for the problem that
they are facing.
In future we plan to conduct similar performance
analysis for other FAHP algorithms and through ex-
perimental analysis such as this, we plan to inves-
tigate differences between conventional AHP tech-
niques with Fuzzy AHP techniques.
REFERENCES
Ataei, M., Mikaeil, R., Hoseinie, S. H., and Hosseini, S. M.
(2012). Fuzzy analytical hierarchy process approach
for ranking the sawability of carbonate rock. Inter-
national Journal of Rock Mechanics and Mining Sci-
ences, 50:83–93.
Boender, C., De Graan, J., and Lootsma, F. (1989). Multi-
criteria decision analysis with fuzzy pairwise compar-
isons. Fuzzy sets and Systems, 29(2):133–143.
Buckley, J. J. (1985). Fuzzy hierarchical analysis. Fuzzy
sets and systems, 17(3):233–247.
B
¨
uy
¨
uk
¨
ozkan, G., Kahraman, C., and Ruan, D. (2004). A
fuzzy multi-criteria decision approach for software
development strategy selection. International Journal
of General Systems, 33(2-3):259–280.
Chang, D.-Y. (1996). Applications of the extent analysis
method on fuzzy ahp. European journal of opera-
tional research, 95(3):649–655.
Deng, H. (1999). Multicriteria analysis with fuzzy pair-
wise comparison. International Journal of Approxi-
mate Reasoning, 21(3):215–231.
Ding, Y., Yuan, Z., and Li, Y. (2008). Performance evalua-
tion model for transportation corridor based on fuzzy-
ahp approach. In Fuzzy Systems and Knowledge Dis-
covery, 2008. FSKD’08. Fifth International Confer-
ence on, volume 3, pages 608–612. IEEE.
Forman, E. H. and Gass, S. I. (2001). The analytic hi-
erarchy process-an exposition. Operations research,
49(4):469–486.
Golany, B. and Kress, M. (1993). A multicriteria evalua-
tion of methods for obtaining weights from ratio-scale
matrices. European Journal of Operational Research,
69(2):210–220.
Kahraman, C., Cebeci, U., and Ulukan, Z. (2003). Multi-
criteria supplier selection using fuzzy ahp. Logistics
information management, 16(6):382–394.
Kilic, K., Sproule, B. A., T
¨
urksen, I. B., and Naranjo, C. A.
(2004). Pharmacokinetic application of fuzzy struc-
ture identification and reasoning. Information Sci-
ences, 162(2):121–137.
Ross, T. J. (1995). Fuzzy Logic With Engineering Applica-
tions. Mcgraw-Hill College, first edition edition.
Saaty, T. L. (1980). The Analytic Hierarchy Process: Plan-
ning, Priority Setting, Resource Allocation (Decision
Making Series). Mcgraw-Hill (Tx).
Takagi, T. and Sugeno, M. (1985). Fuzzy identification
of systems and its applications to modeling and con-
trol. Systems, Man and Cybernetics, IEEE Transac-
tions on, (1):116–132.
Tang, Y.-C., Beynon, M. J., et al. (2005). Application
and development of a fuzzy analytic hierarchy pro-
cess within a capital investment study. Journal of Eco-
nomics and Management, 1(2):207–230.
Tsaur, S.-H., Chang, T.-Y., and Yen, C.-H. (2002). The
evaluation of airline service quality by fuzzy mcdm.
Tourism management, 23(2):107–115.
Uncu, O., Kilic, K., and Turksen, I. (2004). A new fuzzy
inference approach based on mamdani inference us-
ing discrete type 2 fuzzy sets. In Systems, Man and
ICEIS 2016 - 18th International Conference on Enterprise Information Systems
178