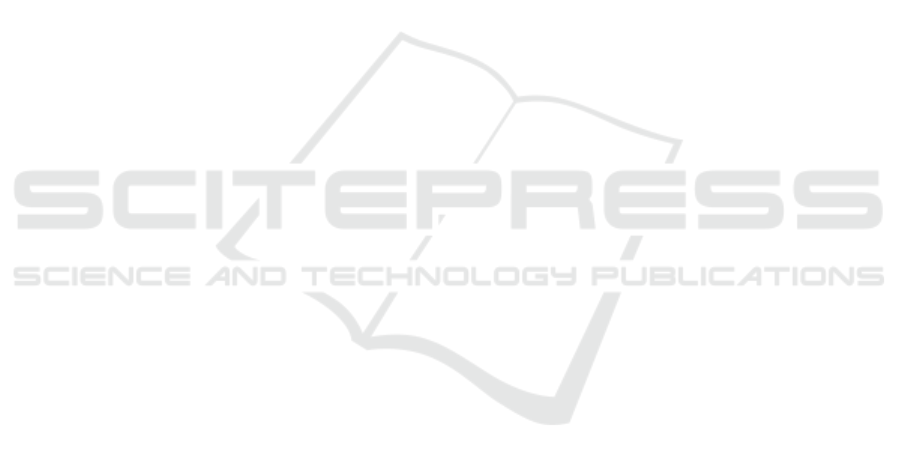
ule the consumption profiles of their users for maxi-
mizing the self-consumption and the economics sav-
ings. DSOs can use consumption profile information
for network balancing, active network management or
for ancillary services. Finally, electricity consump-
tion data are used to evaluate the integration of PV
system in the selected area considering also the distri-
bution network constraints.
In many areas, smart-meters are still not deployed
and data regarding users consumption load profiles
are not available. To give flexibility to our methodol-
ogy, we have integrated a load profile simulation mod-
ule that is able to estimate load profile with a good
accuracy as reported in our previous work (Bottacci-
oli et al., 2015). The load profile simulation module
is able to simulate the consumption profiles for differ-
ent users. For residential users, the module requires
information regarding the size of the houses in square
meters and the number of inhabitants for each house-
hold. For industrial and commercial customers, nor-
malized standard load profiles are used. Those stan-
dard profiles are rescaled with respect to total yearly
or monthly electricity consumption.
5 CONCLUSION
In this paper, we presented a methodology for the de-
velopment of a distributed software infrastructure for
simulating PV system behaviours and evaluating their
integration in a Smart City context. Combining re-
alistic radiation modelling framework and electricity
consumption data, our infrastructure can offer to users
detailed information of PV energy production in real-
sky conditions. With such detailed results, different
users can take the optimal decisions in defining the
structure and the architecture of a solar plant in an ur-
ban context, spanning all scales starting from single
building up to block, district and city.
REFERENCES
Boland, J., Huang, J., and Ridley, B. (2013). Decomposing
global solar radiation into its direct and diffuse com-
ponents. Renew. Sustainable Energy Rev., 28:749–
756.
Bottaccioli, L., Patti, E., Acquaviva, A., Macii, E., Jarre,
M., and Noussan, M. (2015). A tool-chain to foster
a new business model for photovoltaic systems inte-
gration exploiting an energy community approach. In
Proc. of IEEE ETFA2015. IEEE.
Camargo, L. R., Zink, R., Dorner, W., and Stoeglehner, G.
(2015). Spatio-temporal modeling of roof-top photo-
voltaic panels for improved technical potential assess-
ment and electricity peak load offsetting at the munic-
ipal scale. Comput. Environ. Urban Syst., 52:58–69.
De Amicis, R., Conti, G., Patti, D., Ford, M., and Elisei,
P. (2012). I-Scope-Interoperable Smart City Services
through an Open Platform for Urban Ecosystems. na.
de Sousa, L., Eykamp, C., Leopold, U., Baume, O., and
Braun, C. (2012). iguess-a web based system integrat-
ing urban energy planning and assessment modelling
for multi-scale spatial decision making. In Porc. of
iEMSs 2012.
Erbs, D., Klein, S., and Duffie, J. (1982). Estimation
of the diffuse radiation fraction for hourly, daily
and monthly-average global radiation. Solar Energy,
28(4):293–302.
Freitas, S., Catita, C., Redweik, P., and Brito, M. (2015).
Modelling solar potential in the urban environment:
State-of-the-art review. Renew. Sustainable Energy
Rev., 41:915–931.
Ganu, T., Seetharam, D. P., Arya, V., Kunnath, R., Hazra, J.,
Husain, S. A., De Silva, L. C., and Kalyanaraman, S.
(2012). nplug: a smart plug for alleviating peak loads.
In Proc. of e-Energy 2012, page 30. ACM.
Jakubiec, J. A. and Reinhart, C. F. (2013). A method for pre-
dicting city-wide electricity gains from photovoltaic
panels based on lidar and gis data combined with
hourly daysim simulations. Solar Energy, 93:127–
143.
Luka, N., Seme, S., laus, D., tumberger, G., and alik, B.
(2014). Buildings roofs photovoltaic potential assess-
ment based on lidar (light detection and ranging) data.
Energy, 66:598–609.
Mapdwell Solar System. http://www.mapdwell.com.
Marotta, A., Ciccazzo, A., and Rinaudo, S. (2011). Mod-
eling of a smart photovoltaic panel integrated self-
powered and high efficiency DC-DC Boost converter.
PCIM Europe 2011.
Orgill, J. and Hollands, K. (1977). Correlation equation for
hourly diffuse radiation on a horizontal surface. Solar
Energy, 19(4):357–359.
Patti, E., Pons, E., Martellacci, D., Castagnetti, F. B., Ac-
quaviva, A., and Macii, E. (2015). multiflex: Flex-
ible multi-utility, multi-service smart metering archi-
tecture for energy vectors with active prosumers. In
Proc. of SMARTGREENS 2015, pages 288–293.
Patti, E., Syrri, A. L. A., Jahn, M., Mancarella, P., Acqua-
viva, A., and Macii, E. Distributed software infras-
tructure for general purpose services in smart grid.
Ronzino, A., Osello, A., Patti, E., Bottaccioli, L., Danna,
C., Lingua, A. M., Acquaviva, A., Macii, E., Grosso,
M., Messina, G., and Rascon, G. The energy effi-
ciency management at urban scale by means of inte-
grated modelling. In Proc.of SEB-15. Elsevier.
Suri, M., Huld, T., Dunlop, E., and Cebecauer, T. (2008).
Geographic aspects of photovoltaics in europe: con-
tribution of the pvgis website. J-STARS, IEEE Journal
of, 1(1):34–41.
Weather Underground. http://www.wunderground.com/.
Zoha, A., Gluhak, A., Imran, M. A., and Rajasegarar, S.
(2012). Non-intrusive load monitoring approaches
for disaggregated energy sensing: A survey. Sensors,
12(12):16838–16866.
SMARTGREENS 2016 - 5th International Conference on Smart Cities and Green ICT Systems
362