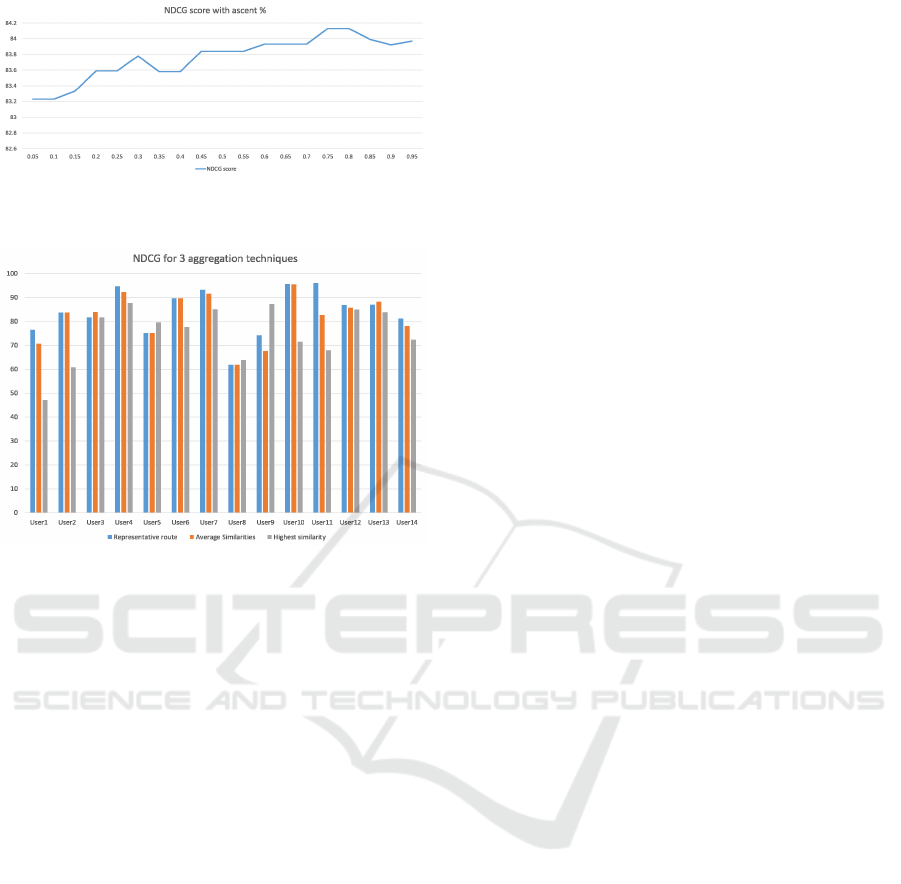
Figure 10: Effect of Varying the Weight of Ascents-to-
Descents (α) on the Overall Quality of Recommendations.
Figure 11: Comparison of the Effect of the different Route-
to-User Similarity Methods.
pants, with a score of 84.13%. The Average Route-to-
User Similarity approach came in second place with
a score of 81.93%. Finally, the Highest Similarity
Pair method produced the lowest result of 75.14%.
Notably, and as indicated in Section 4.2.3, the Rep-
resentative Route approach has the best performance
among the three considered approaches as it does not
require computing all the pair-wise similarities among
all the user routes and location routes.
6 CONCLUSIONS AND FUTURE
WORK
In this research, a classification of running routes
based on route’s nature, performance and visual fea-
tures is introduced. The classification enables filter-
ing the vast amount of running routes available on the
web according to the user’s preferences. Using the
same features of a route, a recommender system is
built to learn the user’s preferences from her previous
recorded runs and provide recommendations of suit-
able running routes in the user’s location of choice.
The recommendations are tested using active runners
history data and annotations and attained a recom-
mendation accuracy of 84.13%.
To further extend the capabilities of the system,
additional data from sensors included in fitness track-
ers and smartphones are to be utilized by the system.
Such data can provide more information about the
surface of the route and the running styles of people.
Additionally, providing recommendations for other
types of activities such as cycling or skiing forms a
potential future use case for this research.
ACKNOWLEDGEMENTS
This work was partially funded by the BMBF project
Multimedia Opinion Mining (MOM: 01WI15002)
and is part of the project SERVICEFACTORY.
REFERENCES
Barber, C. B., Dobkin, D. P., and Huhdanpaa, H. (1996).
The quickhull algorithm for convex hulls. ACM Trans.
Math. Softw., 22(4):469–483.
Chen, Y., Bell, M., and Bogenberger, K. (2007). Reli-
able pretrip multipath planning and dynamic adapta-
tion for a centralized road navigation system. Intel-
ligent Transportation Systems, IEEE Transactions on,
8(1):14–20.
Douglas, D. (1973). Algorithms for the reduction of the
number of points required to represent a line or its a
caricature. The Canadian Cartographer, 10(2):112–
122.
Hirsch, J. A., James, P., Robinson, J. R. M., Eastman,
K. M., Conley, K. D., Evenson, K. R., and Laden,
F. (2014). Using mapmyfitness to place physical ac-
tivity into neighborhood context. Frontiers in Public
Health, 2(19).
Issa, H., Shafaee, A., Agne, S., Baumann, S., and Dengel,
A. (2015). User-sentiment based evaluation for market
fitness trackers - evaluation of fitbit one, jawbone up
and nike+ fuelband based on amazon.com customer
reviews. In ICT4AgeingWell 2015 - Proceedings of
the 1st International Conference on Information and
Communication Technologies for Ageing Well and e-
Health, Lisbon, Portugal, 20-22 May, 2015., pages
171–179.
J
¨
arvelin, K. and Kek
¨
al
¨
ainen, J. (2000). IR evaluation meth-
ods for retrieving highly relevant documents. In SI-
GIR, pages 41–48.
Knoch, S., Chapko, A., Emrich, A., Werth, D., and Loos, P.
(2012). A context-aware running route recommender
learning from user histories using artificial neural net-
works. In Database and Expert Systems Applications
(DEXA), 2012 23rd International Workshop on, pages
106–110.
Pang, G., Takahashi, K., Yokota, T., and Takenaga, H.
(1995). Adaptive route selection for dynamic route
guidance system based on fuzzy-neural approaches.
In Vehicle Navigation and Information Systems Con-
ference, 1995. Proceedings. In conjunction with the
Preference based Filtering and Recommendations for Running Routes
145