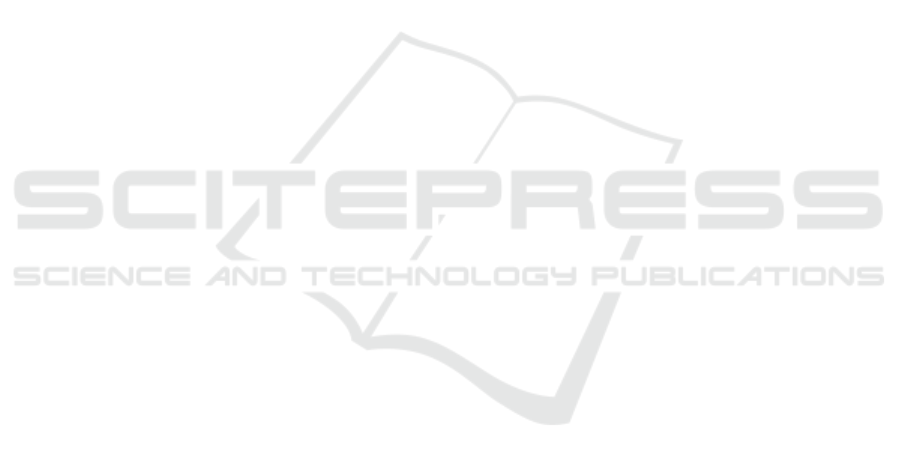
in cloud infrastructures. Future Gener. Comput. Syst.,
28(7):1017–1029.
Fatema, K., Emeakaroha, V. C., Healy, P. D., Morrison, J. P.,
and Lynn, T. (2014). A survey of cloud monitoring
tools: Taxanomy, capabilities and objectives. Jour-
nal of Parallel and Distributed Computing, 74:2918–
2933.
Gander, M., Felderer, M., Katt, B., Tolbaru, A., Breu, R.,
and Moschitti, A. (2013). Anomaly detection in the
cloud: Detecting security incidents via machine learn-
ing. In Trustworthy Eternal Systems via Evolving Soft-
ware, Data and Knowledge, pages 103–116. Springer.
Guan, Q. and Fu, S. (2013a). Adaptive anomaly identi-
fication by exploring metric subspace in cloud com-
puting infrastructures. In Reliable Distributed Sys-
tems (SRDS), 2013 IEEE 32nd International Sympo-
sium on, pages 205–214.
Guan, Q. and Fu, S. (2013b). Wavelet-based multi-scale
anomaly identification in cloud computing systems. In
Global Communications Conference (GLOBECOM),
2013 IEEE, pages 1379–1384.
Guan, Q., Fu, S., DeBardeleben, N., and Blanchard,
S. (2013). Exploring time and frequency domains
for accurate and automated anomaly detection in
cloud computing systems. In Dependable Computing
(PRDC), 2013 IEEE 19th Pacific Rim International
Symposium on, pages 196–205. IEEE.
Gul, I. and Hussain, M. (2011). Distributed cloud intrusion
detection model. International Journal of Advanced
Science and Technology, 34:71–82.
Hodge, V. J. and Austin, J. (2004). A survey of outlier de-
tection methodologies. Artificial Intelligence Review,
22(2):85–126.
Ibidunmoye, O., Hern
´
andez-Rodriguez, F., and Elmroth, E.
(2015). Performance anomaly detection and bottle-
neck identification. ACM Comput. Surv., 48(1):4:1–
4:35.
Lin, M., Yao, Z., Gao, F., and Li, Y. (2015). Toward
anomaly detection in iaas cloud computing platforms.
International Journal of Security and Its Applications,
9(12):175 – 188.
Liu, A., Chen, J. X., and Wechsler, H. (2015). Real-time
timing channel detection in an software-defined net-
working virtual environment. Intelligent Information
Management, 7(06):283.
Mi, H., Wang, H., Yin, G., Cai, H., Zhou, Q., Sun, T.,
and Zhou, Y. (2011a). Magnifier: Online detection of
performance problems in large-scale cloud computing
systems. In Services Computing (SCC), 2011 IEEE
International Conference on, pages 418–425.
Mi, H., Wang, H., Yin, G., Cai, H., Zhou, Q., Sun, T.,
and Zhou, Y. (2011b). Magnifier: Online detection of
performance problems in large-scale cloud computing
systems. In Services Computing (SCC), 2011 IEEE
International Conference on, pages 418–425.
Penn, B. S. (2005). Using self-organizing maps to visual-
ize high-dimensional data. Computers & Geosciences,
31(5):531 – 544.
Reynolds, P., Killian, C., Wiener, J. L., Mogul, J. C., Shah,
M. A., and Vahdat, A. (2006). Pip: Detecting the
unexpected in distributed systems. In Proceedings of
the 3rd Conference on Networked Systems Design &
Implementation - Volume 3, NSDI’06, Berkeley, CA,
USA. USENIX Association.
Sha, W., Zhu, Y., Chen, M., and Huang, T. (2015). Statisti-
cal learning for anomaly detection in cloud server sys-
tems: A multi-order markov chain framework. Cloud
Computing, IEEE Transactions on.
Song, X., Wu, M., Jermaine, C., and Ranka, S. (2007). Con-
ditional anomaly detection. IEEE Trans. on Knowl.
and Data Eng., 19(5):631–645.
Videla, A. and Williams, J. J. (2012). RabbitMQ in Action:
Distributed Messaging for Everyone. Manning Publi-
cations Company.
Wang, C., Talwar, V., Schwan, K., and Ranganathan, P.
(2010). Online detection of utility cloud anomalies us-
ing metric distributions. In Network Operations and
Management Symposium (NOMS), 2010 IEEE, pages
96–103.
Wang, C., Viswanathan, K., Choudur, L., Talwar, V., Sat-
terfield, W., and Schwan, K. (2011). Statistical
techniques for online anomaly detection in data cen-
ters. In Integrated Network Management (IM), 2011
IFIP/IEEE International Symposium on, pages 385–
392.
Welford, B. P. (1962). Note on a method for calculating cor-
rected sums of squares and products. Technometrics,
4(3):419–420.
Zhang, Z., Wang, Y., and Wang, K. (2013). Fault diag-
nosis and prognosis using wavelet packet decomposi-
tion, Fourier transform and artificial neural network.
J. Intell. Manuf., 24(6):1213–1227.
A Wavelet-inspired Anomaly Detection Framework for Cloud Platforms
117