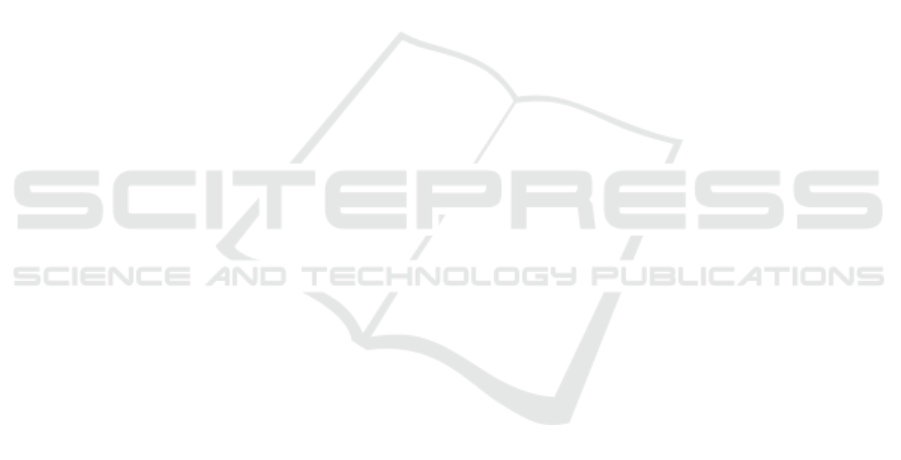
Micro-WNOp: A gold standard for the evaluation of
automatically compiled lexical resources for opinion
mining. Franco Angeli Editore.
Dave, K., Lawrence, S., and Pennock, D. M. (2003). Mining
the peanut gallery: Opinion extraction and semantic
classification of product reviews. In Proceedings of
the 12th international conference on World Wide Web
(WWW ’03), pages 519–528.
Dinu, L. P. and Iuga, I. (2012). The naive bayes classifier
in opinion mining: In search of the best feature set.
In 13th International Conference on Intelligent Text
Processing and Computational Linguistics (CICLing
2012), pages 556–567.
Domingos, P. and Pazzani, M. J. (1997). On the optimality
of the simple bayesian classifier under zero-one loss.
Machine Learning, 29:103–130.
Dragut, E. C., Yu, C., Sistla, P., and Meng, W. (2010). Con-
struction of a sentimental word dictionary. In ACM
International Conference on Information and Knowl-
edge Management (CIKM 2010), pages 1761–1764.
Duda, R. O. and Hart, P. E. (1973). Pattern Classification
and Scene Analysis. John Wiley & Sons, New York.
Esuli, A., Sebastiani, F., and Baccianella, S. (2010). Senti-
wordnet 3.0: An enhanced lexical resource for sen-
timent analysis and opinion mining. In Proceed-
ings of the 7th Conference on International Language
Resources and Evaluation (LREC ’10), pages 2200–
2204.
Fellbaum, C. (1998). WordNet: An Electronic Lexical
Database. MIT Press, Cambridge, MA.
Garcia, M. and Gamallo, P. (2014). Citius: A naive-bayes
strategy for sentiment analysis on english tweets. In
Proceedings of the 8th International Workshop on Se-
mantic Evaluation (SemEval 2014), pages 171–175.
Hassan, A., Korashy, H., and Medhat, W. (2014). Sentiment
analysis algorithms and applications - a survey. Ain
Shams Engineering Journal, 5(4):1093–1113.
Hu, M. and Liu, B. (2004). Mining and summarizing cus-
tomer reviews. In Proceedings of the ACM Interna-
tional Conference on Knowledge Discovery & Data
Mining (SIGKDD), pages 168–177.
Kanayama, H. and Nasukawa, T. (2006). Fully auto-
matic lexicon expansion for domain-oriented senti-
ment analysis. In In Proceedings of the Conference
on Empirical Methods in Natural Language Process-
ing (EMNLP ’06), pages 355–363.
Kennedy, A. and Inkpen, D. (May 2006). Sentiment clas-
sification of movie reviews using contextual valence
shifters. Computational Intelligence, 22(2):110–125.
Kim, S.-M. and Hovy, E. (2006). Identifying and analyzing
judgment opinions. In Proceedings of the Joint Hu-
man Language Technology/North American Chapter
of the ACL Conference (HLT-NAACL-06), pages 200–
207.
Lewis, D. D. (1998). Naive (bayes) at forty: The in-
dependence assumption in information retrieval. In
Proceedings of the European Conference on Machine
Learning (ECML-98), pages 4–15.
Littman, P. and M.L., T. (2002). Unsupervised learning
of semantic orientation from a hundred-billion-word
corpus. Technical report, National Research Council
Canada, Institute for Information Technology.
Liu, B. (2012). Sentiment Analysis and Opinion Mining.
Morgan & Claypool Publishers, San Rafael, US.
Liu, L. and
¨
Ozsu, M. T. (2009). Encyclopedia of Database
Systems. Springer.
Meyer, D., Leisch, F., and Hornik, K. (2003). The support
vector machine under test. Neurocomputing, 55(1–
2):169–186.
Mihalcea, R., Banea, C., and Wiebe, J. (2007). Learn-
ing multilingual subjective language via cross-lingual
projections. In Proceedings of the Association for
Computational Linguistics (ACL 2007), pages 976–
983.
Pang, B., Lee, L., and Vaithyanathan, S. (2002). Thumbs
up? sentiment classification using machine learn-
ing techniques. In Proceedings of Conference on
Empirical Methods in Natural Language Processing
(EMNLP-2002), pages 79–86.
Polanyi, L. and Zaenen, A. (2006). Contextual valence
shifters. In Croft, W. B., Shanahan, J., Qu, Y., and
Wiebe, J., editors, Computing Attitude and Affect in
Text: Theory and Applications, volume 20 of The
Information Retrieval Series, chapter 1, pages 1–10.
Springer Netherlands.
Shanmuganathan, P. and Sakthivel, C. (2015). An effi-
cient naive bayes classification for sentiment analysis
on twitter. Data Mining and Knowledge Engineering,
7(5).
Strapparava, C. and Valitutti, A. (2004). Wordnet-affect: an
affective extension of wordnet. In Proceedings of the
4th International Conference on Language Resources
and Evaluation (LREC 2004), pages 1083–1086.
Taboada, M., Brooke, J., Tofiloski, M., Voll, K., and Stede,
M. (2011). Lexicon-based methods for sentiment
analysis. Computational Linguistics, 37(2):267–307.
Takamura, H., Inui, T., and Okumura, M. (2006). Latent
variable models for semantic orientations of phrases.
In Proceedings of the 11th Conference of the Euro-
pean Chapter of the Association for Computational
Linguistics (EACL 2006), pages 201–208.
Vossen, P. (1998). Introduction to eurowordnet. Computers
and the Humanities, 32(2):73–89.
Wilson, T., Wiebe, J., and Hoffmann, P. (2005). Recog-
nizing contextual polarity in phrase-level sentiment
analysis. In Proceedings of the conference on human
language technology and empirical methods in natu-
ral language processing (HLT/EMNLP 2005), pages
347–354.
Yi, H., Jianyong, D., Xiaoming, C., and Bingzhen, Pei an-
dRuzhan, L. (2005). A new method for sentiment clas-
sification in text retrieval. In Proceedings of the Sec-
ond International Joint Conference on Natural Lan-
guage Processing (IIJCNLP-05), pages 1–9.
Zagibalov, T. and Carroll, J. (2008). Automatic seed word
selection for unsupervised sentiment classfication of
chinese text. In Proceedings of the 22nd International
Conference on Computational Linguistics (COLING
’08), pages 1073–1080.
Zanchetta, E. and Baroni, M. (2005). Morph-it! a free
corpus-based morphological resource for the italian
language. In Proceedings of Corpus Linguistics 2005.
WEBIST 2016 - 12th International Conference on Web Information Systems and Technologies
170