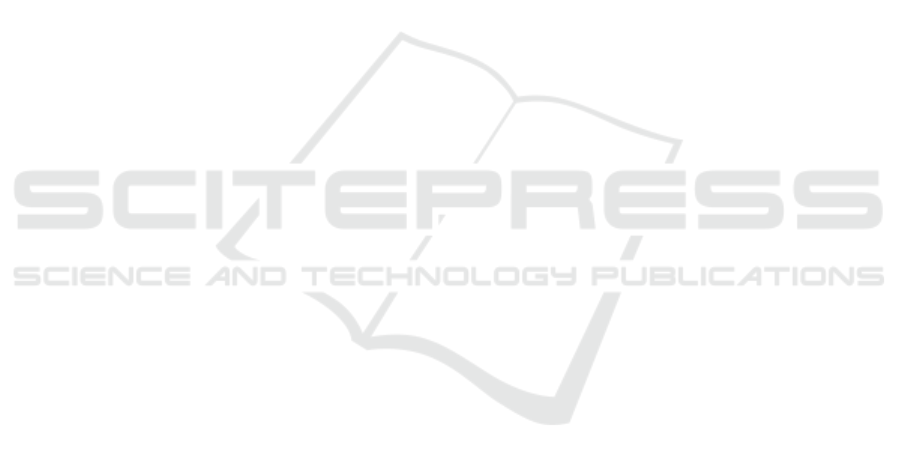
number turns to be 3007. Among the 5 bad bags, two
have size 3, two have size 4, and one has size 5.
Step 2. Put the 3007 good bags in constraint (9b),
solve (9), after 5 iterations, the bad bags number turns
to be 0. The optimal objective value is 2.6406 × 10
6
.
3.7 Polish-3120sp System
Polish-3120sp system represents the Polish networks
during summer 2008 morning peak, with 3120 buses,
505 generators and 3693 transmission line, which
lead to 2615 nonlinear constraints in (2b). Thus, there
are 3120 × 3121/2 = 4868760 complex scalar vari-
ables in the W matrix. We apply the Large-Scale NOA
as follow,
Initialization. Following (Madani et al., 2015a)
to decompose the 3120 buses into 3120 overlapped
bags.The largest bag size is 25, while the smallest bag
size is 1. After the bags decomposition, the total num-
ber of the complex decision variables in (8) is 32637
vs 4868760 complex decision variables in (3). Solve
(8) over the 3120 bags, we find that there are 21 bad
bags,The largest bad bag size is 7, while the smallest
bad bag size is 2. which means the rank of the 21 bags
are more than 1. Other 3099 good bags are all rank-1.
Then go to next step.
Step 1. Set ε
tol
= 10
−5
and put the 3099 good bags
in constraint (9b), solve (9), after 5 iterations, only 2
bad bags with size 2 are still bad, while other bags
turn good.
Step 2. Put the 3118 good bags in constraint (9b),
solve (9), after 4 iterations, the bad bags number turns
to be 0. The optimal objective value is 2.1778 × 10
6
.
It should be noted that, in step 2, the penalized
term of bag-81 was multiplied by 2 to increase the
convergence speed.
4 CONCLUSIONS
OPF over power transmission networks is a difficult
nonconvex optimization problem with numerous non-
linear equality and inequality constraints. We have
developed a large-scale nonsmooth optimization al-
gorithm to compute its optimal solution, which is
efficient and practical for networks with reasonably
large numbers of buses. Applications of NOA to
OPF over three-phase power transmission networks
are currently under consideration.
REFERENCES
Andersen, M. S., Hansson, A., and Vandenberghe, L.
(2014). Reduced-complexity semidefinite relaxations
of optimal power flow problems. IEEE Trans. Power
Systems, 29(4):1855–1863.
Bai, X., Wei, H., Fujisawa, K., and Wang, Y. (2008).
Semidefinite programming for optimal power flow
problems. Elect. Power Energy Syst., 30(6-7):383–
392.
Bonnans, J. F., Gilbert, J. C., Lemarechal, C., and Sagastiz-
abal, C. (2006). Numerical Optimization Theoretical
and Practical Aspects (second edition). Springer.
Bukhsh, W., Grothey, A., McKinnon, K., and Trodden, P.
(2013). Local solutions of the optimal power flow
problem. IEEE Trans. Power Systems, 28(4):4780–
4788.
Carpentier, J. (1962). Contribution to the economic dispatch
problem. Bulletin Society Francaise Electriciens,
3(8):431–447.
Huneault, M. and Galiana, F. D. (1991). A survey of the op-
timal power flow literature. IEEE Trans. Power Sys-
tems, 6(2):762–770.
Lavaei, J. and Low, S. H. (2012). Zero duality gap in opti-
mal power flow problem. IEEE Trans. Power Systems,
27(1):92–107.
Madani, R., Ashraphijuo, M., and Lavaei, J. (2015a).
Promises of conic relaxation for contigency-
constrained optimal power flow problem. IEEE
Trans. Power Systems, 30.
Madani, R., Sojoudi, S., and Lavaei, J. (2015b). Convex
relaxation for optimal power flow problem: Mesh net-
works. IEEE Trans. Power Systems, 30:199–211.
Molzahn, D. K., Holzer, J. T., Lesieutre, B. C., and De-
Marco, C. L. (2013). Implementation of a large-scale
optimal power flow solver based on semidefinite pro-
gramming. IEEE Trans. Power Systems, 28:3987–
3998.
Momoh, J. A., El-Hawary, M. E., and Adapa, R. (1999).
A review of selected optimal power flow literature to
1993. part I: Nonlinear and quadratic programming
approaches. Solar Energy, 14(1):96–104.
Pandya, K. S. and Joshi, S. K. (2008). A survey of optimal
power flow methods. J. of Theoretical and Applied
Information Technology, 4(5):450–458.
Shi, Y., Tuan, H. D., Su, S., and Tam, H. H. M. (2015).
Nonsmooth optimization for optimal power flow over
transmission networks. In Proc. 2015 IEEE Global
Conf. on Signal and Information Processing (Global-
SIP).
Zimmerman, R. D., Murillo-Sanchez, C. E., and Thomas,
R. J. (2011). MATPOWER: steady-state operations,
planning, and analysis tools for power systems re-
search and education. IEEE Trans. Power Systems,
26(1):12–19.
Multiple Matrix Rank Constrained Optimization for Optimal Power Flow over Large Scale Transmission Networks
389