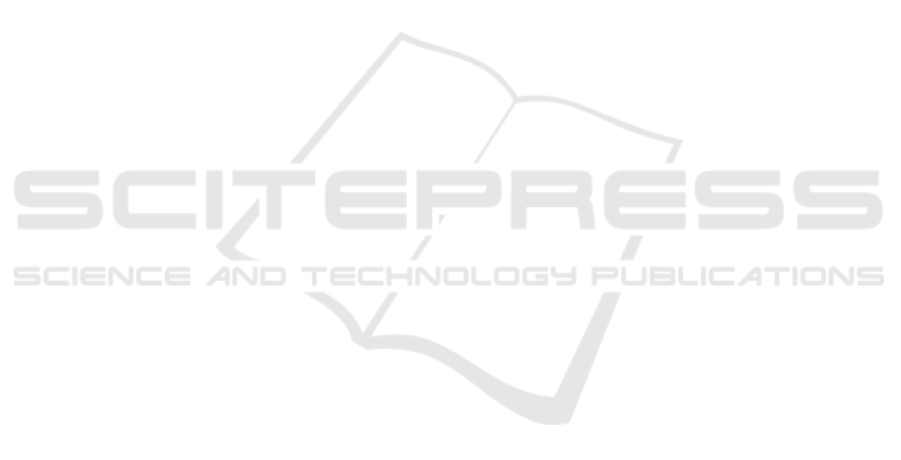
19%, respectively. In particular, the corresponding
average energy saving of the proposed scheduler com-
pared to the Lyapunov alternative is 76%; moreover,
its gap over the IDEAL scheduler remains limited to
30%.
5 CONCLUSION AND FUTURE
RESEARCH DIRECTIONS
The goal of this paper is to provide an adaptive
and online energy-aware resource provisioning and
scheduling of VMs in DVFS-enabled networked data
centers. Also, it is aimed at summarizing key
techniques and mathematical policies that minimize
the data center energy consumption, which is split
into three sub-problems subject to total computing
and communication time’s constraints, while meet-
ing given SLAs. In the process, we identified the
sources of energy consumptions in data centers and
presented a high-level solution to the related sub-
problems. The numerical results highlight that the
proposed approach can guarantee significant average
energy savings over the Standard and Lyapunov alter-
natives. Our proposed scheduler can manage not only
the online workloads, but also the inter-switching
costs among the active discrete frequencies for each
VM. An interesting achievement is that, when com-
munication costs are considered, our method is able
to approach the IDEAL algorithm significantly faster
than Lyapunov, Standard and NetDC models, respec-
tively. Under soft latency constraints, the energy effi-
ciency of the DVFS based systems could be, in prin-
ciple, improved by allowing multiple jobs to be tem-
porarily queued at the middleware layer of the cloud
systems. This paper is just a first effort in a new line
of research. Future extensions of the present work,
currently left as open issues, include: management
of the admission control using split workload estima-
tion, improved data center model that considers more
than one VM per physical server, and introduction of
economic aspects (such as variable VMs cost) in the
optimization problem.
ACKNOWLEDGEMENT
The first three authors acknowledge the support of the
University of Modena and Reggio Emilia through the
project SAMMClouds: Secure and Adaptive Manage-
ment of Multi-Clouds.
REFERENCES
Almeida, J., Almeida, V., Ardagna, D., Cunha,
´
I., Fran-
calanci, C., and Trubian, M. (2010). Joint admis-
sion control and resource allocation in virtualized
servers. Journal of Parallel and Distributed Comput-
ing, 70(4):344–362.
Azodolmolky, S., Wieder, P., and Yahyapour, R. (2013).
Cloud computing networking: challenges and oppor-
tunities for innovations. Communications Magazine,
IEEE, 51(7):54–62.
Baliga, J., Ayre, R. W., Hinton, K., and Tucker, R. (2011).
Green cloud computing: Balancing energy in process-
ing, storage, and transport. Proceedings of the IEEE,
99(1):149–167.
Canali, C. and Lancellotti, R. (2014). Exploiting ensem-
ble techniques for automatic virtual machine cluster-
ing in cloud systems. Automated Software Engineer-
ing, 21(3):319–344.
Canali, C. and Lancellotti, R. (2016). Parameter Tuning
for Scalable Multi-Resource Server Consolidation in
Cloud Systems. Communications Software and Sys-
tems, 11(4):172 – 180.
Chase, J. S., Anderson, D. C., Thakar, P. N., Vahdat, A. M.,
and Doyle, R. P. (2001). Managing energy and server
resources in hosting centers. ACM SIGOPS Operating
Systems Review, 35(5):103–116.
Cordeschi, N., Shojafar, M., Amendola, D., and Baccarelli,
E. (2014). Energy-efficient adaptive networked data-
centers for the qos support of real-time applications.
The Journal of Supercomputing, 71(2):448–478.
Cordeschi, N., Shojafar, M., and Baccarelli, E. (2013).
Energy-saving self-configuring networked data cen-
ters. Computer Networks, 57(17):3479–3491.
Cugola, G. and Margara, A. (2012). Processing flows of
information: From data stream to complex event pro-
cessing. ACM Computing Surveys (CSUR), 44(3):15.
Daniel Gmach, J. R. and Cherkasova, L. (2012). Sell-
ing t-shirts and time shares in the cloud. In Proc. of
12th IEEE/ACM International Symposium on Cluster,
Cloud and Grid Computing, CCGrid 2012, Ottawa,
Canada, May 13-16, 2012, pages 539–546.
Grant, M. and Boyd, S. (2015). Cvx: Matlab software for
disciplined convex programming.
Herbert, S. and Marculescu, D. (2007). Analysis
of dynamic voltage/frequency scaling in chip-
multiprocessors. In ISLPED, pages 38–43.
ACM/IEEE.
Kimura, H., Sato, M., Hotta, Y., Boku, T., and Takahashi, D.
(2006). Emprical study on reducing energy of parallel
programs using slack reclamation by dvfs in a power-
scalable high performance cluster. In IEEE CLUS-
TER’06, pages 1–10. IEEE.
Mathew, V., Sitaraman, R. K., and Shenoy, P. (2012).
Energy-aware load balancing in content delivery net-
works. In INFOCOM, 2012 Proceedings IEEE, pages
954–962. IEEE.
Mishra, A., Jain, R., and Durresi, A. (2012). Cloud com-
puting: networking and communication challenges.
Communications Magazine, IEEE, 50(9):24–25.
TEEC 2016 - Special Session on Tools for an Energy Efficient Cloud
396