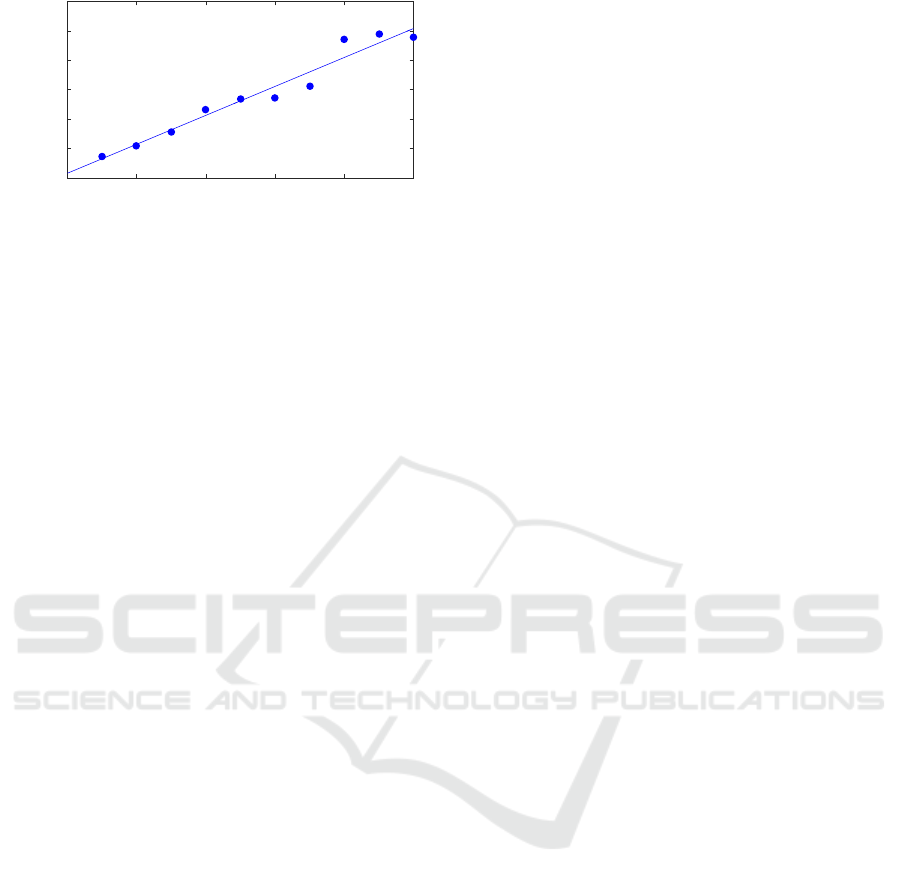
0 0.1 0.2 0.3 0.4 0.5
β
yx
0
0.02
0.04
0.06
0.08
0.1
−1/q
Figure 11: The inverse negative slope
−1/q
of the linear
portion of the curves in the top panel of Figure 10 as a
function of the coupling.
erroneous results. It was noted already by Sugihara
et al. (2012) that CCM does not correctly predict the
direction of causality when the coupling is so strong
that it results in synchronization of variables, as we
have also seen above. Further, our study shows that
the method seems very sensitive to the particular dy-
namics of the model system, and CCM also fails to
correctly predict the direction of causality in cases
where the coupling is weak to moderate. Interestingly,
we have shown that both types of cases where CCM
fails is associated with a failure to fit the observed val-
ues of cross-mapped correlations to the function
ρ(L)
in Eq. 5. It would therefore seem that the failure to
produce a good fit to
ρ(L)
is an indicator that CCM is
not applicable to the data.
Another aspect of CCM performance concerns con-
ditions of noise. Generally, we observe that CCM is
fairly robust to random noise and makes reliable infer-
ences at varying degrees of coupling under conditions
of lower noise levels. However, for higher levels of
noise CCM correlations drop linearly as a function
of added noise. For systems subject to noise our re-
sults suggest that the rate of convergence is a more
robust indicator of cross-mapping convergence and
hence of causality. In applications where noise can
be controlled, injecting noise into the system at differ-
ent noise levels presents an opportunity to gauge the
strength of coupling between variables.
Together our results warrant caution in the applica-
tion of CCM to real-world data for purposes of causal
inference, and care must be taken to look in detail at
the convergence properties of the correlations between
observed data and cross-mapped estimates. Our re-
sults suggest that fitting the correlation coefficients
as a function of library length can give an indication
of the applicability of CCM. When applied under the
right circumstances, the method has the potential not
only to inform the researcher about the causal direc-
tion of dynamics between coupled variables, but by
controlled injection of noise, we can also infer the
coupling strength between the variables.
ACKNOWLEDGEMENTS
We would like to acknowledge the Interacting Minds
Centre, Aarhus University, for providing the ideal en-
vironment for the authors’ collaboration.
REFERENCES
Abarbanel, H. (1996). Analysis of Observed Chaotic Data.
Springer New York.
Boccaletti, S., Kurths, J., Osipov, G., Valladares, D. L., and
Zhou, C. S. (2002). The synchronization of chaotic
systems. Physics Reports, 366(1–2):1–101.
BozorgMagham, A. E., Motesharrei, S., Penny, S. G., and
Kalnay, E. (2015). Causality Analysis: Identifying
the Leading Element in a Coupled Dynamical System.
PLOS ONE, 10(6):e0131226.
Ferretti, A. and Rahman, N. K. (1988). A study of coupled
logistic map and its applications in chemical physics.
Chemical Physics, 119(2–3):275–288.
Fraser, A. M. and Swinney, H. L. (1986). Independent coor-
dinates for strange attractors from mutual information.
Physical Review A, 33(2):1134–1140.
Granger, C. W. J. (1969). Investigating Causal Relations
by Econometric Models and Cross-spectral Methods.
Econometrica, 37(3):424–438.
Jespersen, S. N. (2013). Personal communication.
Kantz, H. and Schreiber, T. (1997). Nonlinear Time Series
Analysis. Cambridge University Press, New York,USA.
Lakoff, G. (2010). Why it Matters How we Frame the Envi-
ronment. Environmental Communication,4(1):70–81
Lloyd, A. L. (1995). The coupled logistic map: a sim-
ple model for the effects of spatial heterogeneity on
population dynamics. Journal of Theoretical Biology,
173(3):217–230.
Ma, H., Aihara, K., and Chen, L. (2014). Detecting Causality
from Nonlinear Dynamics with Short-term Time Series.
Scientific Reports, 4:7464.
Marwan, N., Carmen Romano, M., Thiel, M., and Kurths, J.
(2007). Recurrence plots for the analysis of complex
systems. Physics Reports, 438(5–6):237–329.
May, R. M. (1976). Simple mathematical models with very
complicated dynamics. Nature, 261(5560):459–467.
McBride, J. C., Zhao, X., Munro, N. B., Jicha, G. A.,
Schmitt, F. A., Kryscio, R. J., Smith, C. D., and Jiang,
Y. (2015). Sugihara causality analysis of scalp EEG for
detection of early Alzheimer’s disease. NeuroImage:
Clinical, 7:258–265.
McCracken, J. M. and Weigel, R. S. (2014). Conver-
gent cross-mapping and pairwise asymmetric inference.
Physical Review E, 90(6):062903.
Mønster, D. (2013). XMAP. https://github.com/danm0nster
/xmap.
Moran, P. (1953). The statistical analysis of the Canadian
Lynx cycle. Australian Journal of Zoology, 1(3):291–
298.
Inferring Causality from Noisy Time Series Data - A Test of Convergent Cross-Mapping
55