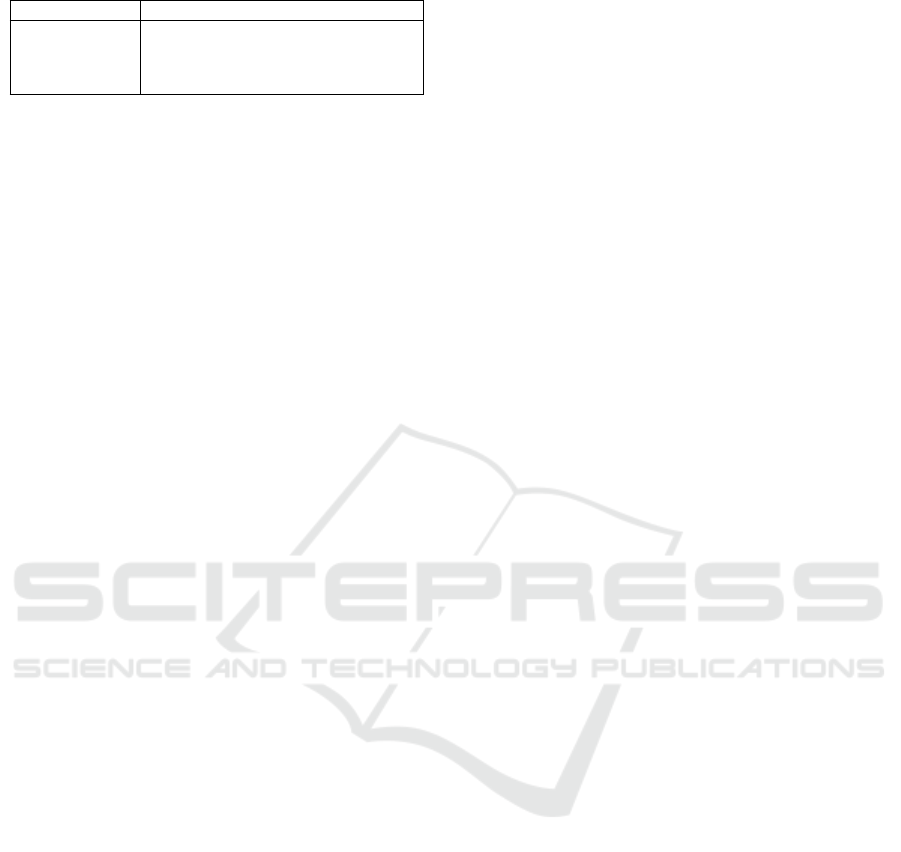
Table 4: Net daily gain of different tactics.
SO LC p
max
c
ebill
c
aging
c
soc
g
net
X MinPeaks 317.7 0.13 0.12 0.00 0.00
7 MinPeaks 7839 0.19 0.51 -0.03 -0.42
7 Opport. 8627 0.12 0.07 0.01 0.04
7 Secure 8775 0.24 0.00 0.00 0.00
LC: c
0
= 0.24 e.
On simulated days, we can see that the only ef-
ficient way to reduce the maximum power peak pur-
chased from the grid is the MinPeaks Controller fol-
lowing the strategy. That way, the purchasing peak is
less than 5% of the maximum possible power peak.
The tactics on their own do not absorb power peaks,
because a predictive strategy is necessary to achieve
such a goal. On the other hand, the MinPeaks Con-
troller following the strategy only succeeds to com-
pensate aging costs by a gain on the energy bill. Thus,
its net daily gain is null in this context. Please note
that, if the gap between on- and off-peak hourly cost
grows, the net daily gain of this tactic following the
strategy also increases.
If the MinPeaks LC is on its own (for example
during a long network failure), the net daily gain be-
comes negative but the number of (and the maximum)
power peaks stays below the other controllers alone.
However, if the goal is only to minimize the net
daily gain, without taking into account power peaks,
the Opportunistic LC alone is the best (as far as the
ratio between the high/low prices is moderate).
Finally, the Secure tactic does not use storage
units at all and thus, has a higher energy bill, but a
null aging cost.
6 CONCLUSIONS
In this paper we formalize what we call the sourc-
ing problem and its application to a multisource ele-
vator. We give a method to solve it, composed of a
Local Controller coupled with a Strategic Optimizer.
That allows us to tackle real-time issues while taking
into account long-term objectives based on forecasts.
A linear formulation allows us to compute a strategy
and a centralized rule-based algorithm gives us a tac-
tic. Three Local Controller parametrizations are il-
lustrated, and one will choose the most adapted to its
studied energy hub.
A legitimate criticism of this work could be that
gains in euros are very low. First, let us recall that
these results are for a unique elevator, while in prac-
tice several elevators could share the same battery.
Second, reducing power consumption peaks could be
very useful to be demand-response aware and to re-
spect future energy limitation laws. In those cases,
minimizing the energy bill is only an appreciable ad-
dition to the consumption peaks minimization. For
the multisource elevator use case, having storage units
allows the elevator to evacuate disabled people in case
of fire. Using these storage units to minimize the en-
ergy bill could amortize the investment. Third, en-
ergy prices are going to increase in future years, as
will storage units performances. That will also in-
crease the benefit of this solution. Finally, the pro-
posed solution can be applied to other multisource
systems where it can be much more profitable. In fact,
as the consumption increases, the profitability also in-
creases, especially if reselling energy is possible.
On the other hand, we will have to compare the
cost of maintaining our rather complex solution, re-
garding the customer value in each use case. If the
maximum power peak purchased from the grid is not
an issue and the energy tariff considered is a typi-
cal French peak/off-peak tariff, the Opportunistic LC
(or a similar classical rule-based controller) should be
used.
As future work, we will study our method robust-
ness to forecast uncertainties. This is a critical issue
and studying it could allow us to give performance
guarantees to potential customers. A sensibility study
of controller parameters will also be conducted. Fi-
nally, a real-life experiment, in a building equipped
with a BMS, would worth being conducted.
ACKNOWLEDGEMENTS
This work has been conducted as part of the Arrow-
head European project and has been partially funded
by the Artemis/Ecsel Joint Undertaking, supported by
the European Commission and French Public Author-
ities, under grant agreement number 332987.
REFERENCES
Bilbao, E. and Barrade, P. (2012). Optimal energy man-
agement of an improved elevator with energy storage
capacity based on dynamic programming. In Energy
Conversion Congress and Exposition (ECCE), 2012
IEEE, page 3479–3484.
Boutin, V., Desdouits, C., Louvel, M., Pacull, F., Vergara-
Gallego, M. I., Yaakoubi, O., Chomel, C., Crignon,
Q., Duhoux, C., Genon-Catalot, D., Lefevre, L.,
Pham, T. H., and Pham, V. T. (2014). Energy optimi-
sation using analytics and coordination, the example
of lifts. In Emerging Technology and Factory Automa-
tion (ETFA), 2014 IEEE, pages 1–8. IEEE.
Desdouits, C., Alamir, M., Boutin, V., and Pape, C. L.
(2015). Multi-source elevator energy optimization
The Sourcing Problem - Energy Optimization of a Multisource Elevator
29