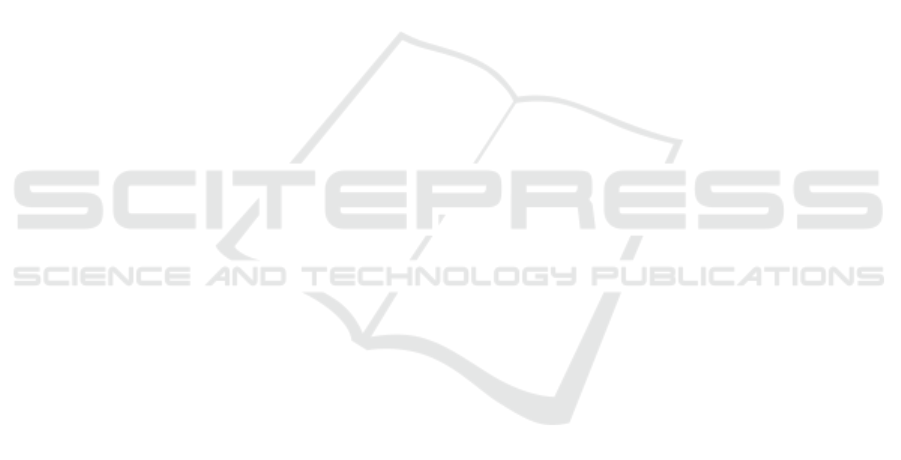
tions and Anomaly Detection method. But we can
conclude that a good cluster needs to contain intrusive
and normal traffic in order to optimize the threshold
cluster by cluster and is at best statistical independent
to reduce as much noise as possible. Our experiment
pointed out that a proficient clustering can uncover
abnormal traffic which is difficult to detect in the cu-
mulative data. Especially our manual SSH intrusion
attempt and the stealthy scans are normally difficult
to detect by Anomaly Detection methods, because
they do not transparently change volume or shape of
the network traffic. However, we were easily able
to detect them. It is likely that both, Anomaly De-
tection with and without clustering, could be equally
improved by using more complex detection methods.
There is a wide range of, here unused, Anomaly De-
tection schemes (e.g. Local Outlier Detection) and
metrics (packets per second) available, which proved
useful in other publications. However, we deliber-
ately wanted to keep the experiment simple and focus
on the effect of our preprocessing instead Anomaly
Detection. We are aware that our approach heav-
ily depends on the network characteristics. Our ex-
perimentation provided good results, because it was
possible to separate networks into sub-networks with
unique characteristics. It remains an open question
whether it is possible to always reach this goal with
any network. We belief that the task of finding signif-
icant features to split the traffic is event simpler when
the network is larger than the network used for our
experiments. In this work, we only performed tests
on a single data set and artifical data, because we
avoided the use of obsolete public data sets. As future
prospects we would like to extend our experiments to
a variaty of networks, evaluate more parameters and
extend our work with ways to cluster obfuscated pro-
tocols and encrypted traffic.
REFERENCES
Bouzida, Y., Cuppens, F., Cuppens-Boulahia, N., and Gom-
bault, S. (2004). Efficient intrusion detection using
principal component analysis. In 3
´
eme Conf
´
erence sur
la S
´
ecurit
´
e et Architectures R
´
eseaux (SAR), La Londe,
France.
Brauckhoff, D., Salamatian, K., and May, M. (2009). Ap-
plying pca for traffic anomaly detection: Problems
and solutions. In INFOCOM 2009, IEEE, pages
2866–2870. IEEE.
Chen, C. and Liu, L.-M. (1993). Joint estimation of model
parameters and outlier effects in time series. Journal
of the American Statistical Association, 88(421):284–
297.
Chhabra, P., Scott, C., Kolaczyk, E. D., and Crovella, M.
(2008). Distributed spatial anomaly detection. In
INFOCOM 2008. The 27th Conference on Computer
Communications. IEEE. IEEE.
Cretu-Ciocarlie, G. F., Stavrou, A., Locasto, M. E., and
Stolfo, S. J. (2009). Adaptive anomaly detection via
self-calibration and dynamic updating. In Recent Ad-
vances in Intrusion Detection, pages 41–60. Springer.
Davis, J. J. and Clark, A. J. (2011). Data preprocessing for
anomaly based network intrusion detection: A review.
Computers & Security, 30(6):353–375.
Denning, D. E. (1987). An intrusion-detection model. Soft-
ware Engineering, IEEE Transactions on, (2):222–
232.
Heady, R., Luger, G., Maccabe, A., and Servilla, M. (1990).
The architecture of a network-level intrusion detection
system. Department of Computer Science, College of
Engineering, University of New Mexico.
Izakian, H. and Pedrycz, W. (2014). Anomaly detection and
characterization in spatial time series data: A cluster-
centric approach.
Kim, J. and Bentley, P. J. (2002). Towards an artificial
immune system for network intrusion detection: an
investigation of dynamic clonal selection. In Evolu-
tionary Computation, 2002. CEC’02. Proceedings of
the 2002 Congress on, volume 2, pages 1015–1020.
IEEE.
Kim, M.-S., Kong, H.-J., Hong, S.-C., Chung, S.-H., and
Hong, J. W. (2004). A flow-based method for ab-
normal network traffic detection. In Network Op-
erations and Management Symposium, 2004. NOMS
2004. IEEE/IFIP, volume 1, pages 599–612. IEEE.
Leung, K. and Leckie, C. (2005). Unsupervised anomaly
detection in network intrusion detection using clus-
ters. In Proceedings of the Twenty-eighth Australasian
conference on Computer Science-Volume 38, pages
333–342. Australian Computer Society, Inc.
Li, Z., Gao, Y., and Chen, Y. (2005). Towards a high-speed
router-based anomaly/intrusion detection system.
Mahoney, M. and Chan, P. K. (2001). Phad: Packet header
anomaly detection for identifying hostile network traf-
fic.
M
¨
unz, G., Li, S., and Carle, G. (2007). Traffic anomaly de-
tection using k-means clustering. In GI/ITG Workshop
MMBnet.
Szmit, M., Adamus, S., Bugała, S., and Szmit, A. (2012).
Anomaly detection 3.0 for snort. Snort. AD Project.
SECRYPT 2016 - International Conference on Security and Cryptography
396