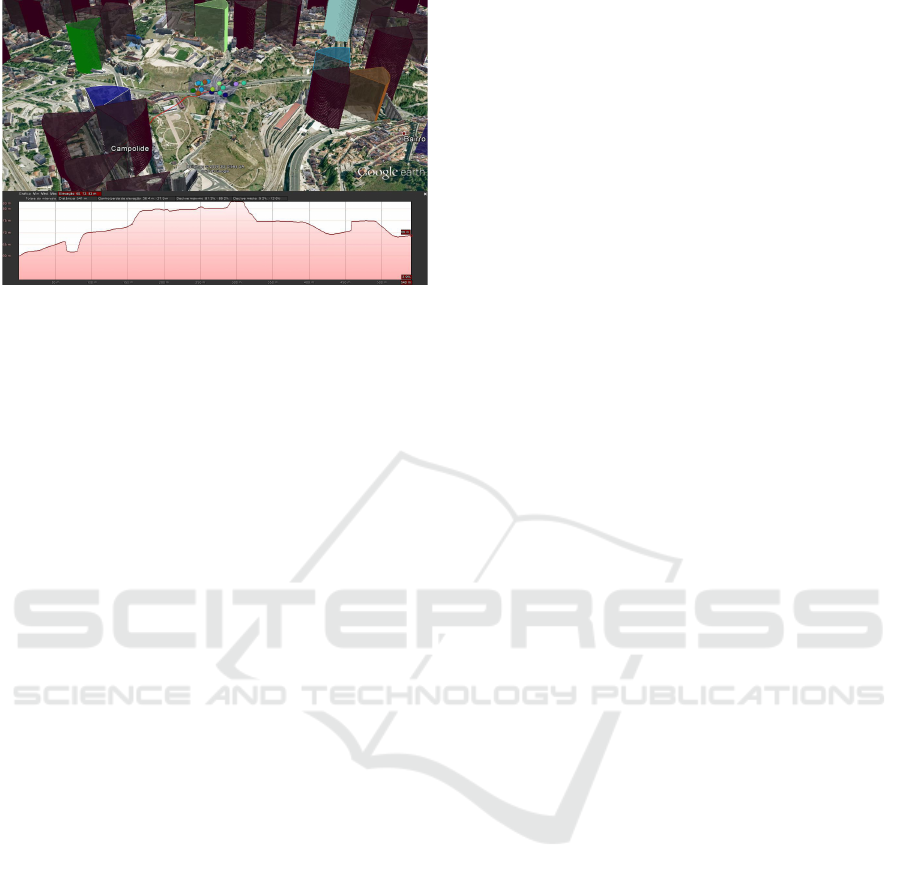
Figure 12: Pilot pollution cluster terrain profile.
pilot pollution H
cell
index, compared to the overshoot-
ing harshness index.
By definition, pilot pollution areas display an ex-
cessive number of pilot signals. Sometimes, this num-
ber is high enough, that enables the algorithm to sug-
gest which cell(s) are being victimized. In this sce-
nario, the detection is done, but insufficient informa-
tion retains the algorithm from the harshness evalua-
tion and the affected cell identification.
6 CONCLUSIONS
This paper presented a new approach for automatic
detection of low coverage and high interference sce-
narios (overshooting and pilot pollution) in UMTS
/LTE networks. These algorithms, based on period-
ically extracted DT measurements, identify the prob-
lematic cluster locations and compute harshness met-
rics, at cluster and cell level, quantifying the extent of
the problem.
The proposed algorithms were validated for a live
network urban scenario. 830 3G cells were self-
diagnosed and performance metrics were computed.
The results showed that for an urban area and with
a high site density, the main optimization efforts rely
on the interference mitigation and not coverage opti-
mization. Moreover, the pilot pollution scenarios are
the most prevalent.
The cluster division, with a RF correlation dis-
tance limitation, provides a simplification for the an-
tenna physical parameter optimization algorithms, in
the sense that, the optimization process can be re-
duced to the cluster’s centroids.
Future work is in motion by adding self-
optimization capabilities to the algorithms, which will
automatically suggest physical and parameter opti-
mization actions, based on the already developed
harshness metrics.
ACKNOWLEDGEMENTS
This work was supported by the Instituto de
Telecomunicac¸
˜
oes (IT) and the Portuguese Founda-
tion for Science and Technology (FCT) under project
PEst-OE/EEI/LA0008/2013.
REFERENCES
Duarte, D., Vieira, P., Rodrigues, A. J., and Silva, N. (2015).
A new approach for crossed sector detection in live
mobile networks based on radio measurements. In
Wireless Personal Multimedia Communications Symp.
- WPMC, volume 1.
Ericsson (2015). Ericsson mobility report. Technical report,
ERiCSSON.
Izenman, A. J. (2008). Modern Multivariate Statistical
Techniques: Regression, Classification, and Manifold
Learning. Springer Publishing Company, Incorpo-
rated, 1 edition.
Kysti, P., Meinil, J., Hentil, L., Zhao, X., Jms, T., Schnei-
der, C., Narandzi, M., Milojevi, M., Hong, A., Ylitalo,
J., Holappa, V., Alatossava, M., Bultitude, R., Jong,
Y., and Rautiainen, T. (2007). Ist-4-027756 winner ii
d1.1.2 v1.2. Technical report, EBITG, TUI, UOULU,
CU/CRC, NOKIA.
Sallent, O., Perez-Romero, J., Sanchez-Gonzalez, J.,
Agusti, R., Diaz-Guerra, M., Henche, D., and Paul,
D. (2011). Automatic detection of sub-optimal perfor-
mance in umts networks based on drive-test measure-
ments. In Network and Service Management (CNSM),
2011 7th International Conference on, pages 1–4.
Sanchez-Gonzalez, J., Sallent, O., P
´
erez-Romero, J., and
Agust
´
ı, R. (2013). A multi-cell multi-objective self-
optimisation methodology based on genetic algo-
rithms for wireless cellular networks. Int. Journal of
Network Management, 23(4):287–307.
Sousa, M., Martins, A., Vieira, P., Oliveira, N., and Ro-
drigues, A. (2015). Caracterizacao da fiabilidade
de medidas r
´
adio em larga escala para redes auto-
otimizadas. In 9. Congresso do Comit
´
e Portugu
ˆ
es da
URSI - ”5G e a Internet do futuro”.
Vieira, P., Silva, N., Fernandes, N., Rodrigues, A. J.,
and Varela, L. (2014). Improving accuracy for otd
based 3G geolocation in real urban/suburban environ-
ments. In Wireless Personal Multimedia Communica-
tions Symp. - WPMC, volume 1.
Witten, I. H. and Frank, E. (2005). Data Mining: Practi-
cal Machine Learning Tools and Techniques, Second
Edition (Morgan Kaufmann Series in Data Manage-
ment Systems). Morgan Kaufmann Publishers Inc.,
San Francisco, CA, USA.
Zheng, N. and Xue, J. (2009). Statistical Learning and Pat-
tern Analysis for Image and Video Processing. Ad-
vances in Pattern Recognition. Springer.
Self-Diagnosing Low Coverage and High Interference in 3G/4G Radio Access Networks based on Automatic RF Measurement Extraction
39