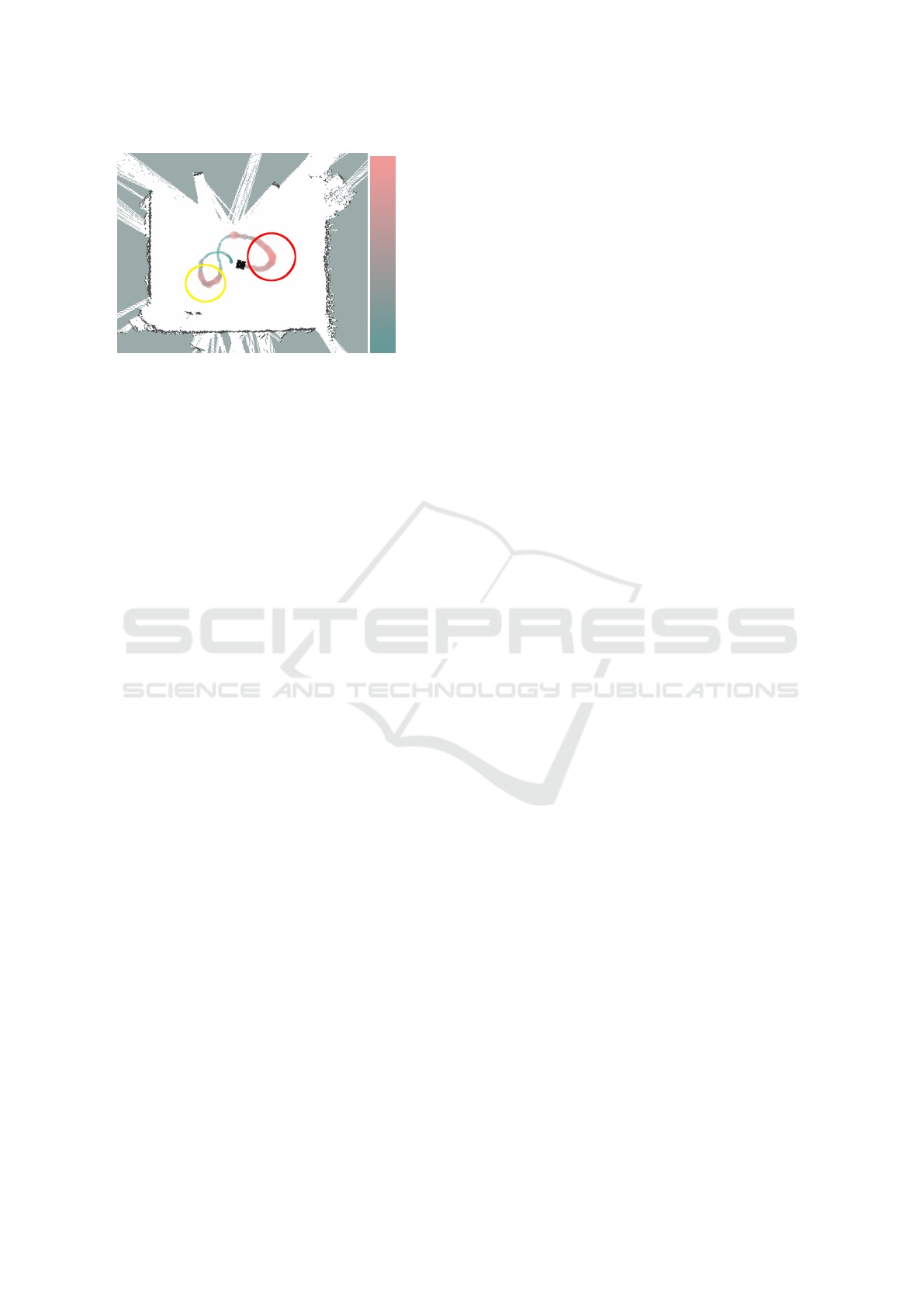
-0.1
-0.2
-0.3
-0.4
-0.5
LRR
Figure 9: This figure presents the LRR visualization during
robotic navigation.
Figure 9 shows a grid map, which was generated with
the fused scan and the ROS SLAM tool gmapping.
The outliers are caused by the MPR, but can be re-
duced by filtering the MPR scan, for example via ra-
dial outlier removal, before fusing it with the LiDAR.
The LRR is visualized along the robot path in the grid
map as well.
Equivalent to Figure 8, the yellow circle presents
the phase with operating fog machine and the red cir-
cle marks the phase, where the robot is too close to
the hedge. A map or trajectory containing LRR in-
formation can give the operator additional informa-
tion about an environment. The LRR can be used as
an alert signal for operators to make them control the
robot more carefully. It could be used to trigger the
measurement of gas sensors.
5 CONCLUSION
With this work we have shown that the MPR is a use-
ful scanning device and can be used in low visibility
environments. The MPR is suitable for applications
such as rescue robotics and field robotics. We have
developed a new fusion strategy in order to reduce the
influence of fog, smoke, dust, etc. on a single LiDAR
scan. Our approach enhances the use for scan regis-
tration based SLAM, where a clean scan is preferred.
Additionally, we introduced the LRR, which can be
used as a fast fog or smoke detector under certain con-
ditions. More data sets involving fog or smoke will be
needed, for further evaluation of our proposed meth-
ods. Future work will include gas and smoke sensors
to investigate correlation with the LRR, the integra-
tion of a data fusion through joint probability distri-
butions for case I and the investigation of outlier re-
duction methods for radar data.
ACKNOWLEDGMENT
This work has partly been supported within H2020-
ICT by the European Commission under grant agree-
ment number 645101 (SmokeBot).
REFERENCES
Adams, M. and Jose, E. (2012). Robotic navigation and
mapping with radar. Artech House.
Breunig, M. M., Kriegel, H.-P., Ng, R. T., and Sander, J.
(2000). Lof: Identifying density-based local outliers.
ACM SIGMOD International Conference on Manage-
ment of Data, 29(2):93–104.
Brooker, G., Scheding, S., Bishop, M., and Hennessy, R.
(2005). Development and application of millimeter
wave radar sensors for underground mining. IEEE
Sensors Journal, 5(6):12701280.
Clark, S. and Dissanayake, G. (1999). Simultaneous local-
isation and map building using milimetre wave radar
to extract natural features. Internation Conference on
Robotics and Automation.
Clark, S. and Whyte, H. D. (1998). The design of a high
performance mmw radar system for autonomous land
vehicle navigation. In Field and Service Robotics.
Detlefsen, J., Rozmann, M., and Lange, M. (1993). 94 hgz
3-d imaging radar sensor for industrial environments.
EARSeL ADVANCEA IN REMOTE SENSING.
Fritsche, P. and Wagner, B. (2015). Comparison of two
radar-based scanning-techniques for the use in robotic
mapping. In Informatics in Control, Automation and
Robotics (ICINCO), 2015 12th International Confer-
ence on, volume 01, pages 365–372.
Grisetti, G. (2005). Improving grid-based slam with rao-
blackwellized particle filters by adaptive proposals
and selective resampling.
Marck, J. W., Mohamoud, A., van Heijster, R., et al. (2013).
Indoor radar slam a radar application for vision and
gps denied environments. In Radar Conference (Eu-
RAD), 2013 European, pages 471–474. IEEE.
Salman, R., Willms, I., Sakamoto, T., Sato, T., and Yarovoy,
A. (2013). Environmental imaging with a mobile uwb
security robot for indoor localisation and positioning
applications. In Microwave Conference (EuMC), 2013
European, pages 1643–1646.
Vivet, D., Checchin, P., and Chapuis, R. (2013). Local-
ization and mapping using only a rotating fmcw radar
sensor. Sensors.
Willeke, K., Baron, P., and Martonen, T. (1993). Aerosol
Measurement: Principles, Techniques and Applica-
tions, volume 6. [New York, NY]: Mary Ann Liebert,
Inc., c1988-2007.
Yamauchi, B. (2010). Fusing ultra-wideband radar and li-
dar for small ugv navigation in all-weather conditions.
Proc. SPIE 7692, Unmanned Systems Technology XII.
ICINCO 2016 - 13th International Conference on Informatics in Control, Automation and Robotics
36