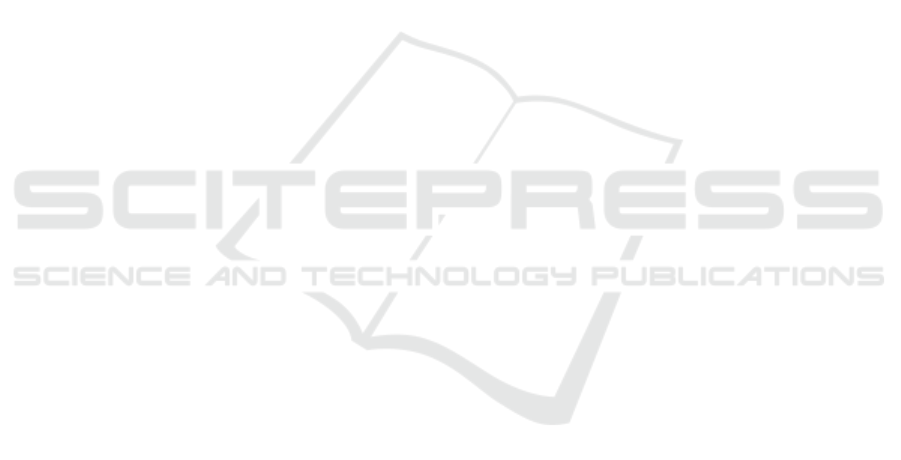
8.1 Mobile Application Studies
B
¨
ohmer et al. (2011) performed one of the first large
scale study on mobile app usage with a panel of An-
droid smartphone users. They utilized a similar con-
cept of app sessions defined by foregrounding and
backgrounding of apps. However they did not look
at the app usage concentration as their focus was
on sequential and temporal app patterns. Similarly
Soikkeli et al. (2013) analyzed smartphone app us-
age from a panel of Finnish smartphone users. They
detailed app usage statistics for several very popu-
lar apps but again do not look at usage concentration
among apps.
Falaki et al. (2010) analyzed smartphone app us-
age with two panels of users: one Windows Phone
and one Android based. They do illustrate and model
device-level app usage and find that exponential dis-
tributions fit most device app usage data well.
Closest to our work, Jung et al. (2014) examined
the aggregate app usage for a panel of Korean An-
droid smartphone users. They found highly concen-
trated usage though with lower Gini coefficient than
the coefficient for our smartphone data. They also
found differences in usage concentration between app
categories. However, they only examined aggregate
level app usage and did not examine device level app
usage. Furthermore, they did not examine app usage
concentration across multiple device types.
8.2 Multidevice Studies
Montanez et al. (2014) and Wang et al. (2013) exam-
ined multidevice usage but often only from the per-
spective of a single app (search) as the data was col-
lected from Microsoft’s search service (Bing).
Hintze et al. (2014) analyzed both smartphone and
tablet usage from the large Device Analyzer dataset.
Device Analyzer is a dataset based on a popular An-
droid device monitoring app from Cambridge Uni-
versity (Wagner et al., 2014). The dataset contains
both smartphone and tablet devices however corre-
lating usage of smartphone and tablet devices to a
single user is not possible thus comparing app us-
age between devices with the same user is infeasible.
Furthermore individual app names are not available
therefore app-specific insights are difficult to extract.
Finally, Google (2012) and Microsoft (2013) stud-
ied multidevice usage through combinations of sur-
veys, user diaries, and device meters but did not study
multidevice app usage concentration.
9 CONCLUSIONS
In this work we have analyzed app usage with a fo-
cus on usage concentration in a multidevice context
including smartphones, tablets, and personal comput-
ers. Furthermore, we analyze usage concentration on
both an aggregate (market) level and individual device
level. Thus we provide a thorough view of app usage
concentration.
We highlight a few key takeaways from our analy-
sis. Overall, we show that, on an aggregate level, app
usage concentration will vary significantly by device
type and thus future work, for example in modeling,
should take these differences into account to gain a
holistic view. Whereas, on an individual level, we find
significant diversity in app usage concentration even
for users with the same device type, therefore charac-
terizing a typical user is difficult. Finally, we show
that several app usage measures are not strongly cor-
related between devices of the same user, thus empha-
sizing the need to capture all of users devices rather
than simply extrapolating from a single device. In
other words, a multidevice approach is warranted.
REFERENCES
Alstott, J., Bullmore, E., and Plenz, D. (2014). powerlaw:
A python package for analysis of heavy-tailed distri-
butions. PLoS ONE, 9(1):e85777.
B
¨
ohmer, M., Hecht, B., Sch
¨
oning, J., Kr
¨
uger, A., and Bauer,
G. (2011). Falling asleep with angry birds, facebook
and kindle: A large scale study on mobile application
usage. In Proceedings of the 13th International Con-
ference on Human Computer Interaction with Mobile
Devices and Services, MobileHCI ’11, pages 47–56,
New York, NY, USA. ACM.
Church, K., Ferreira, D., Banovic, N., and Lyons, K. (2015).
Understanding the challenges of mobile phone usage
data. In Proceedings of the 17th International Con-
ference on Human-Computer Interaction with Mobile
Devices and Services, MobileHCI ’15, pages 504–
514, New York, NY, USA. ACM.
Clauset, A., Shalizi, C. R., and Newman, M. E. J. (2009).
Power-law distributions in empirical data. SIAM Re-
view, 51(4):661–703.
Cowell, F. A. and Flachaire, E. (2007). Income distribution
and inequality measurement: The problem of extreme
values. Journal of Econometrics, 141(2):1044–1072.
Cowell, F. A. and Flachaire, E. (2014). Statistical methods
for distributional analysis. In Atkinson, A. and Bour-
guignon, F., editors, Handbook of Income Distribution
SET vols. 2A-2B, Handbooks in economics. Elsevier
Science.
Falaki, H., Mahajan, R., Kandula, S., Lymberopoulos, D.,
Govindan, R., and Estrin, D. (2010). Diversity in
WINSYS 2016 - International Conference on Wireless Networks and Mobile Systems
50