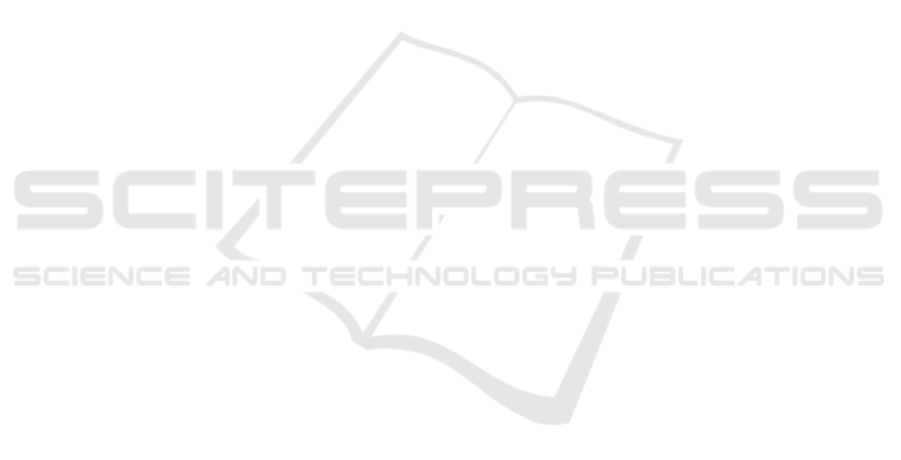
of all, the techniques should be modified so to be
implementable using recursive estimation schemes.
Moreover, by following a classical training-testing
approach, the techniques above present some limi-
tations. Different sensors may in fact differ even if
nominally being constructed in the same way. More-
over sensors may change their statistical behavior in
time, due to aging or mechanical shocks. This means
that techniques based on results from a controlled en-
vironment on just one sensor and just once are even-
tually not entirely meaningful.
A robust approach must indeed perform contin-
uous learning for each sensor independently in a
non-controlled environment by performing informa-
tion fusion steps, e.g., combining also information
from other sensors like odometry, ultrasonic and ac-
celerometers.
This information-fusion continuous-learning al-
gorithm nonetheless must be based on some prelim-
inary results on what are the statistical models of tri-
angulation Lidars and on how inference can be per-
formed on them. This paper can thus be seen as the
first step towards more evolved strategies.
REFERENCES
Alhashimi, A., Varagnolo, D., and Gustafsson, T. (2015).
Joint temperature-lasing mode compensation for time-
of-flight lidar sensors. Sensors, 15(12):31205–31223.
Anderson, D., Herman, H., and Kelly, A. (2005). Experi-
mental characterization of commercial flash ladar de-
vices. In International Conference of Sensing and
Technology, volume 2.
Andreasson, H., Triebel, R., and Burgard, W. (2005).
Improving plane extraction from 3d data by fusing
laser data and vision. In Intelligent Robots and
Systems, 2005.(IROS 2005). 2005 IEEE/RSJ Interna-
tional Conference on, pages 2656–2661. IEEE.
Atanacio-Jiménez, G., González-Barbosa, J.-J., Hurtado-
Ramos, J. B., Ornelas-Rodríguez, F. J., Jiménez-
Hernández, H., García-Ramirez, T., and González-
Barbosa, R. (2011). Lidar velodyne hdl-64e calibra-
tion using pattern planes. International Journal of Ad-
vanced Robotic Systems, 8(5):70–82.
Blais, F. (2004). Review of 20 years of range sensor devel-
opment. Journal of Electronic Imaging, 13(1).
Brown, D. C. (1964). An advanced reduction and calibra-
tion for photogrammetric cameras. Technical report,
DTIC Document.
Campos, D., Santos, J., Gonçalves, J., and Costa, P. (2016).
Modeling and simulation of a hacked neato xv-11
laser scanner. In Robot 2015: Second Iberian Robotics
Conference, pages 425–436. Springer.
Chen, C.-Y. and Chien, H.-J. (2012). On-site sensor re-
calibration of a spinning multi-beam lidar system us-
ing automatically-detected planar targets. Sensors,
12(10):13736–13752.
Croarkin, C. and Tobias, P. (2006). Nist/sematech
e-handbook of statistical methods.
NIST/SEMATECH, July. Available online:
http://www.itl.nist.gov/div898/handbook.
Duane, C. B. (1971). Close-range camera calibration. Pho-
togrammetric engineering, 37(8):855–866.
Glennie, C. (2012). Calibration and kinematic analysis of
the velodyne hdl-64e s2 lidar sensor. Photogrammet-
ric Engineering & Remote Sensing, 78(4):339–347.
Glennie, C. and Lichti, D. D. (2010). Static calibration and
analysis of the velodyne hdl-64e s2 for high accuracy
mobile scanning. Remote Sensing, 2(6):1610–1624.
Glennie, C. and Lichti, D. D. (2011). Temporal stability
of the velodyne hdl-64e s2 scanner for high accuracy
scanning applications. Remote Sensing, 3(3):539–
553.
Gong, X., Lin, Y., and Liu, J. (2013). 3d lidar-camera ex-
trinsic calibration using an arbitrary trihedron. Sen-
sors, 13(2):1902–1918.
Gordon, M. and Meidow, J. (2013). Calibration of a multi-
beam laser system by using a tls-generated reference.
ISPRS Annals of Photogrammetry, Remote Sensing
and Spatial Information Sciences II-5 W, 2:85–90.
Gustafsson, F. (2010). Statistical sensor fusion. Studentlit-
teratur,.
Jokinen, O. (1999). Self-calibration of a light striping sys-
tem by matching multiple 3-d profile maps. In 3-
D Digital Imaging and Modeling, 1999. Proceedings.
Second International Conference on, pages 180–190.
IEEE.
Kneip, L., Tâche, F., Caprari, G., and Siegwart, R. (2009).
Characterization of the compact hokuyo urg-04lx 2d
laser range scanner. In Robotics and Automation,
2009. ICRA’09. IEEE International Conference on,
pages 1447–1454. IEEE.
Konolige, K., Augenbraun, J., Donaldson, N., Fiebig, C.,
and Shah, P. (2008). A low-cost laser distance sen-
sor. In Robotics and Automation, 2008. ICRA 2008.
IEEE International Conference on, pages 3002–3008.
IEEE.
Kümmerle, R., Grisetti, G., and Burgard, W. (2011). Si-
multaneous calibration, localization, and mapping.
In Intelligent Robots and Systems (IROS), 2011
IEEE/RSJ International Conference on, pages 3716–
3721. IEEE.
Lee, K.-H. and Ehsani, R. (2008). Comparison of two 2d
laser scanners for sensing object distances, shapes,
and surface patterns. Computers and electronics in
agriculture, 60(2):250–262.
Lima, J., Gonçalves, J., and Costa, P. J. (2015). Modeling of
a low cost laser scanner sensor. In CONTROLO’2014–
Proceedings of the 11th Portuguese Conference on
Automatic Control, pages 697–705. Springer.
McIvor, A. M. (1999). Calibration of a laser stripe profiler.
In 3-D Digital Imaging and Modeling, 1999. Proceed-
ings. Second International Conference on, pages 92–
98. IEEE.
ICINCO 2016 - 13th International Conference on Informatics in Control, Automation and Robotics
316