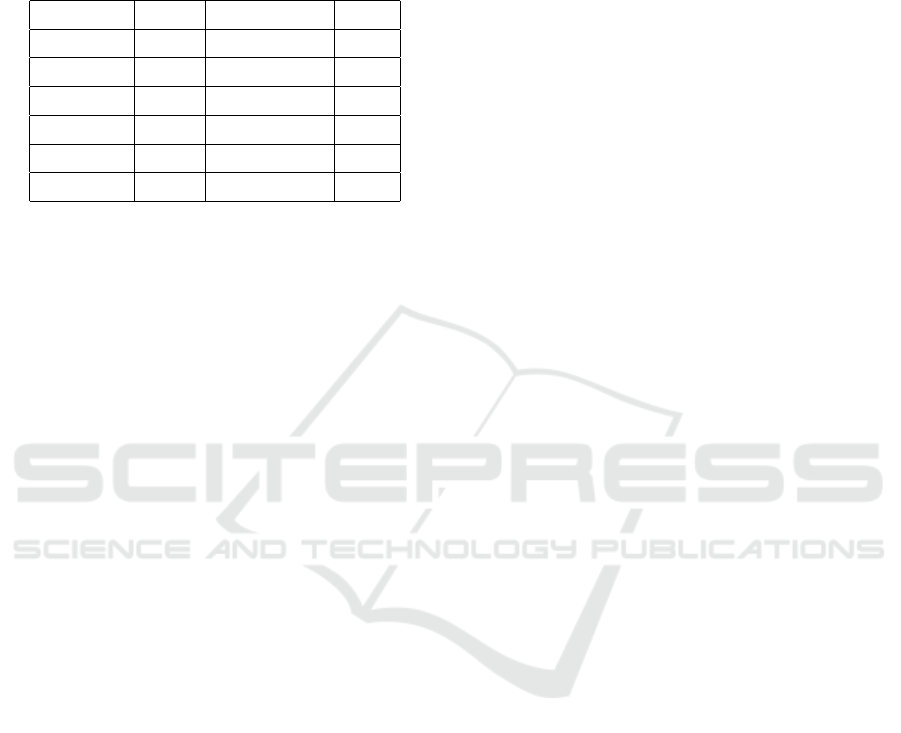
Table 3: Mean and standard deviation normalized entropies
from six iterations of training and testing, where the datasets
from two task types were used for training, and the rest data
were presented for inference. The total datasets are col-
lected from performing four task types. The MICAD mea-
surements of the two classifiers in each test iteration are also
listed.
Test Task Types Classifier
Normalized Entropy
µ ± std.err.
MICAD
OI and WF
SOGP
SVM
0.703 ± 0.402
0.498 ± 0.351
1.30
1.52
OB and WF
SOGP
SVM
0.900 ± 0.232
0.151 ± 0.290
1.44
2.08
OB and OI
SOGP
SVM
0.796 ± 0.369
0.354 ± 0.215
1.52
1.56
DC and WF
SOGP
SVM
0.550 ± 0.331
0.218 ± 0.286
1.00
1.05
DC and OB
SOGP
SVM
0.535 ± 0.367
0.304 ± 0.332
1.01
1.12
DC and OI
SOGP
SVM
0.857 ± 0.271
0.641 ± 0.263
1.41
1.56
5 CONCLUSION
This paper reported an unsupervised approach for
learning and recognizing human motion patterns per-
forming various contextual task types from unla-
belled demonstrations, attempting to facilitate auton-
omy sharing to assist mobile robot teleoperation. The
motion patterns were described with a set of intu-
itive, compact and salient task features. The DPGMM
was employed to cluster the motion patterns based
on the task feature data, where the number of poten-
tial motion components was inferred from the data it-
self instead of being manually specified a priori or
estimated through model selection. Moreover, both
overlaps and distinctions of the task execution pat-
terns can be discovered through clustering, which is
used as a knowledge base for interpreting the query
patterns later on. Post clustering, the SOGP classi-
fier was used to recognize which motion pattern the
human operator executes during operation, taking ad-
vantage of its outstanding confidence estimation when
making predictions and scalability to large datasets.
Extensive evaluations were carried out in indoor sce-
narios with a holonomic mobile robot. The exper-
imental results from the real data verified that, the
proposed approach serves as a generic framework for
representing and exploiting the knowledge of the hu-
man motion patterns performing various contextual
task types without manual annotations, which is not
only able to recognize the task types seen during train-
ing, but also generalizable to appropriately interpret
the motion patters of task types not used for training,
and more importantly, the proposed approach is capa-
ble of detecting unknown motion patterns distinctive
from those used in the training set, due to the superior
introspective capability of the SOGP classifier, hence
provides a significant step towards a life-long adap-
tive assistive robot.
REFERENCES
Blei, D. M., Jordan, M. I., et al. (2006). Variational infer-
ence for dirichlet process mixtures. Bayesian analysis,
1(1):121–143.
Chang, C.-C. and Lin, C.-J. (2011). Libsvm: a library for
support vector machines. ACM Transactions on Intel-
ligent Systems and Technology (TIST), 2(3):27.
Csat
´
o, L. and Opper, M. (2002). Sparse on-line gaussian
processes. Neural computation, 14(3):641–668.
Dragan, A. and Srinivasa, S. (2012). Formalizing assistive
teleoperation. R: SS.
Fong, T., Thorpe, C., and Baur, C. (2001). Advanced inter-
faces for vehicle teleoperation: Collaborative control,
sensor fusion displays, and remote driving tools. Au-
tonomous Robots, pages 77–85.
Gao, M., Oberl
¨
ander, J., Schamm, T., and Z
¨
ollner, J. M.
(2014). Contextual Task-Aware Shared Autonomy for
Assistive Mobile Robot Teleoperation. In Intelligent
Robots and Systems (IROS), 2014 IEEE/RSJ Interna-
tional Conference on. IEEE.
Gao, M., Schamm, T., and Z
¨
ollner, J. M. (2016). Contex-
tual Task Recognition to Assist Mobile Robot Teleop-
eration with Introspective Estimation using Gaussian
Process. In Autonomous Robot Systems and Compe-
titions (ARSC), 2016 IEEE International Conference
on. IEEE.
Grimmett, H., Paul, R., Triebel, R., and Posner, I. (2013).
Knowing when we don’t know: Introspective clas-
sification for mission-critical decision making. In
Robotics and Automation (ICRA), 2013 IEEE Inter-
national Conference on, pages 4531–4538. IEEE.
Hauser, K. (2013). Recognition, prediction, and plan-
ning for assisted teleoperation of freeform tasks. Au-
tonomous Robots, 35(4):241–254.
Hughes, M. C. and Sudderth, E. (2013). Memoized on-
line variational inference for dirichlet process mixture
models. In Advances in Neural Information Process-
ing Systems, pages 1133–1141.
Okada, Y., Nagatani, K., Yoshida, K., Tadokoro, S.,
Yoshida, T., and Koyanagi, E. (2011). Shared au-
tonomy system for tracked vehicles on rough terrain
based on continuous three-dimensional terrain scan-
ning. Journal of Field Robotics, 28(6):875–893.
Sa, I., Hrabar, S., and Corke, P. (2015). Inspection of pole-
like structures using a visual-inertial aided vtol plat-
form with shared autonomy. Sensors, 15(9):22003–
22048.
Sheridan, T. B. (1992). Telerobotics, automation and human
supervisory control. The MIT press.
Stefanov, N., Peer, A., and Buss, M. (2010). Online in-
tention recognition in computer-assisted teleoperation
systems. In Haptics: Generating and Perceiving Tan-
gible Sensations, pages 233–239. Springer.
Unsupervised Contextual Task Learning and Recognition for Sharing Autonomy to Assist Mobile Robot Teleoperation
245