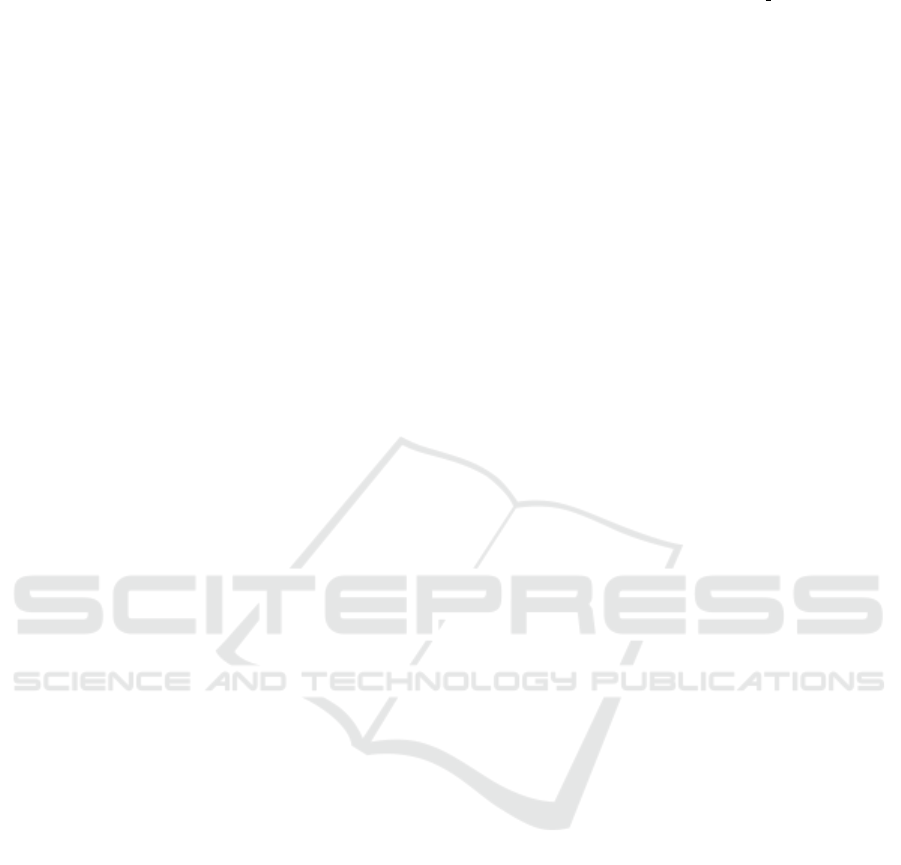
6 CONCLUSION
We have been shown that it is possible to find parame-
ters for the dynamics even if the model takes no flex-
ibility of the bearing into account, which moves the
whole body. To get more accurate results the solver
packs the bearing’s flexibility into the flexibility be-
tween joints and links. The predicted behavior of the
robot based on the identified model parameters has
deviations to the measured data. These deviations are
larger whenever the velocities of the second and third
bodies change direction, because the bearing’s flexi-
bility gets stimulated. The overall approximation is
fitting to the measurements well. However, while the
model is reasonably good and, at the same time, very
simple, we suspect it will not be good enough for our
controller.
By now, we are working on an extension of the
model presented herein, where the flexibility of the
bearing is part of the dynamic model. We believe that
this can be achieved by putting two new joints before
the first one, acting directly on the same coordinate
system as joint one. The bodies of the two inserted
joints will have no mass and no inertia. Their rotation
is about the x and y axes of joint one. The flexibility
is given by a spring acting between the origin and the
joints position. We hope that this will give us a better
model and separate the behavior of joint two and three
from the behavior of the bearing.
Additionally, we have to calibrate the cameras for
the ball tracking, too. We want to add this calibration
into the calibration we were stated herein. Moreover,
we can use the cameras to examine in some positions
the position of the links 2 and 3.
ACKNOWLEDGEMENT
This work has been supported by the Graduate School
SyDe, funded by the German Excellence Initiative
within the University of Bremen’s institutional strat-
egy.
REFERENCES
Albu-Schaeffer, A., Ott, C., and Hirzinger, G. (2007). A
unified passivity-based control framework for posi-
tion, torque and impedance control of flexible joint
robots. The International Journal of Robotics Re-
search, 26(1):23–39.
Cheng, P. and Oelmann, B. (2010). Joint-angle measure-
ment using accelerometers and gyroscopes — a sur-
vey. IEEE Transactions on Instrumentation and Mea-
surement, 59(2):404–414.
Featherstone, R. (2012). Spatial v2 (version 2).
http://royfeatherstone.org/spatial/v2/notice.html.
Grotjahn, M., Daemi, M., and Heimann, B. (2001). Fric-
tion and rigid body identification of robot dynam-
ics. International Journal of Solids and Structures,
38(1013):1889 – 1902.
Hertzberg, C. (2008). A framework for sparse, non-linear
least squares problems on manifolds. Diploma thesis,
Universit
¨
at Bremen.
Hertzberg, C., Wagner, R., and Frese, U. (2012). Tutorial
on quick and easy model fitting using the slom frame-
work. In Stachniss, C., Schill, K., and Uttal, D., ed-
itors, Spatial Cognition VIII, volume 7463 of Lecture
Notes in Computer Science, pages 128–142. Springer-
Verlag Berlin Heidelberg.
Hertzberg, C., Wagner, R., Frese, U., and Schr
¨
oder, L.
(2013). Integrating generic sensor fusion algorithms
with sound state representations through encapsula-
tion of manifolds. Information Fusion, 14(1):57–77.
Mata, V., Benimeli, F., Farhat, N., and Valera, A. (2005).
Dynamic parameter identification in industrial robots
considering physical feasibility. Advanced Robotics,
19(1):101–119.
Moberg, S. (2010). Modeling and Control of Flexible Ma-
nipulators. PhD thesis, Link
¨
oping University, Auto-
matic Control, The Institute of Technology.
Narendra, K. S. and Parthasarathy, K. (1990). Identifica-
tion and control of dynamical systems using neural
networks. IEEE Transactions on Neural Networks,
1(1):4–27.
Olsson, H., strm, K., de Wit, C. C., Gfvert, M., and Lischin-
sky, P. (1998). Friction models and friction compen-
sation. European Journal of Control, 4(3):176 – 195.
Sch
¨
uthe, D. and Frese, U. (2015). Optimal control with state
and command limits for a simulated ball batting task.
In IEEE/RSJ International Conference on Intelligent
Robots and Systems (IROS), 2015, pages 3988–3994.
Siciliano, B. and Khatib, O. (2008). Springer handbook of
robotics. Springer.
Staufer, P. and Gattringer, H. (2012). State estimation on
flexible robots using accelerometers and angular rate
sensors. Mechatronics, 22(8):1043 – 1049.
Vuong, N. D. and Ang Jr, M. H. (2009). Dynamic model
identification for industrial robots. Acta Polytechnica
Hungarica, 6(5):51–68.
Wu, J., Wang, J., and You, Z. (2010). An overview of
dynamic parameter identification of robots. Robotics
and Computer-Integrated Manufacturing, 26(5):414 –
419.
ICINCO 2016 - 13th International Conference on Informatics in Control, Automation and Robotics
346