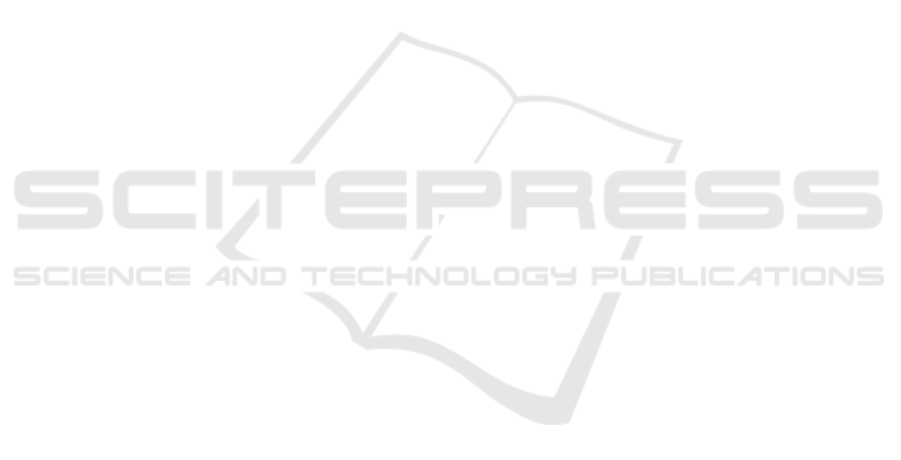
ridor (20, 21) and entrance (22) are caused by the in-
ability to enter the kitchen via the west door (25). Fi-
nally, the robot drives back to door (a) and completes
the map (26-29). T
Exp
= 83.8min,
k
ET
k
= 247.4m
and 30 NBVs represent a slightly better performance
than in the previous run. The total scan time is 33 min
45 s (no scan at vp 2, 16, 19, 25, 29), the minimum
travel time is 12 min and the time penalty for slow-
downs 38 min.
In sum, table 1 shows that the dynamic frontier-
based approach is always the fastest due to the time
needed to construct the ground plans for the other two
strategies. However, these two produce significantly
more complete maps, especially regarding the meet-
ing room, both in simulation and reality. All three
procedures need notably more time, more NBVs and
a longer trajectory for the real world scenario. They
all manage to avoid deadlocks and to create a suit-
able map within a reasonable amount of time. All
in all, in simulation the ground plan-based approach
provides the best trade-off between needed time and
map quality, whereas in reality the hybrid approach is
most promising.
5 CONCLUSION
The paper has analyzed the performance of three
NBV exploration strategies, based on representative
test runs in a simulated office scene and in its real
world counterpart. They all use the same weighted
sum of influence factors for scoring vps, online map
updates and adaptation of the exploration trajectory.
They differ in the kind of mapped features (2.5D
grid map vs. ground plan of floor and ceiling) and
corresponding estimation of information gain (fron-
tier length vs. area of difference polygons). The
dynamic frontier-based approach yields always the
fastest, but incomplete results, whereas the best trade-
off between needed time and map quality is provided
by the ground plan-based procedure in simulation and
by the hybrid strategy in reality.
Future work concentrates on a comparison with
state of the art approaches and on analyzing the in-
fluence of the layout of the environment on the re-
sults, in order to check that the strategies work in gen-
eral. Other topics are to find a generally optimal set
of scoring weights and to improve and evaluate the
room-wise exploration approach of (Schmidt, 2006)
in obstructed real world scenarios.
REFERENCES
Amigoni, F. (2008). Experimental evaluation of some ex-
ploration strategies for mobile robots. ICRA.
Amigoni, F., Caglioti, V., and Galtarossa, U. (2004). A mo-
bile robot mapping system with an information-based
exploration strategy. ICINCO.
Amigoni, F. and Gallo, A. (2005). A multi-objective explo-
ration strategy for mobile robots. ICRA.
Amigoni, F., Li, A. Q., and Holz, D. (2013). Evaluating the
impact of perception and decision timing on autono-
mous robotic exploration. ECMR.
Basilico, N. and Amigoni, F. (2011). Exploration strategies
based on multi-criteria decision making for search and
rescue autonomous robots. AAMAS.
Eliazar, A. and Parr, R. (2003). DP-SLAM: Fast, robust
simultaneous localization and mapping without pre-
determined landmarks. IJCAI.
Freda, L. and Oriolo, G. (2005). Frontier-based probabilis-
tic strategies for sensor-based exploration. ICRA.
Gonz
´
alez-Ba
˜
nos, H., Mao, E., Latombe, J.-C., Murali, T.
M., and Efrat, A. Planning robot motion strategies for
efficient model construction. ISRR.
Gonz
´
alez-Ba
˜
nos, H. and Latombe, J.-C. (2002). Navigation
strategies for exploring indoor environments. IJRR
21(10-11).
Holz, D., Basilico, N., Amigoni, F., and Behnke, S. (2011).
A comparative evaluation of exploration strategies and
heuristics to improve them. ECMR.
Maffei, R., Jorge, V. A. M., Prestes, E., and Kolberg, M.
(2014). Integrated exploration using time-based po-
tential rails. ICRA.
Makarenko, A., Williams, S., Bourgault, F., and Durrant-
Whyte, H. (2002). An experiment in integrated explo-
ration. IROS.
Oriolo, G., Vendittelli, M., Freda, L., and Troso, G. (2004).
The SRT method: randomized strategies for explo-
ration. ICRA.
Proetzsch, M., Luksch, T., and Berns, K. (2010). Develop-
ment of complex robotic systems using the behavior-
based control architecture iB2C. RAS 58(1).
Quinlan, S. and Khatib, O. (1993). Elastic bands: connect-
ing path planning and control. ICRA.
Schmidt, D., Luksch, T., Wettach, J., and Berns, K. (2006).
Autonomous behavior-based exploration of office en-
vironments. ICINCO.
Surmann, H., N
¨
uchter, A., and Hertzberg, J. (2003). An
autonomous mobile robot with a 3D laser range finder
for 3D exploration and digitalization of indoor envi-
ronments. RAS 45(3-4).
Wettach, J. and Berns, K. (2010). Dynamic fron-
tier based exploration with a mobile indoor robot.
ISR/ROBOTIK.
Wettach, J. and Berns, K. (2012). Ground plan based explo-
ration with a mobile indoor robot. ROBOTIK.
Wettach, J. and Berns, K. (2014). Combining dynamic fron-
tier based and ground plan based exploration: a hybrid
approach. ISR/ROBOTIK.
Wurm, K. M., Stachniss, C., and Burgard, W. (2008). Coor-
dinated multi-robot exploration using a segmentation
of the environment. IROS.
Yamauchi, B. (1997). A frontier-based approach for autono-
mous exploration. CIRA.
Experimental Evaluation of Some Indoor Exploration Strategies
287