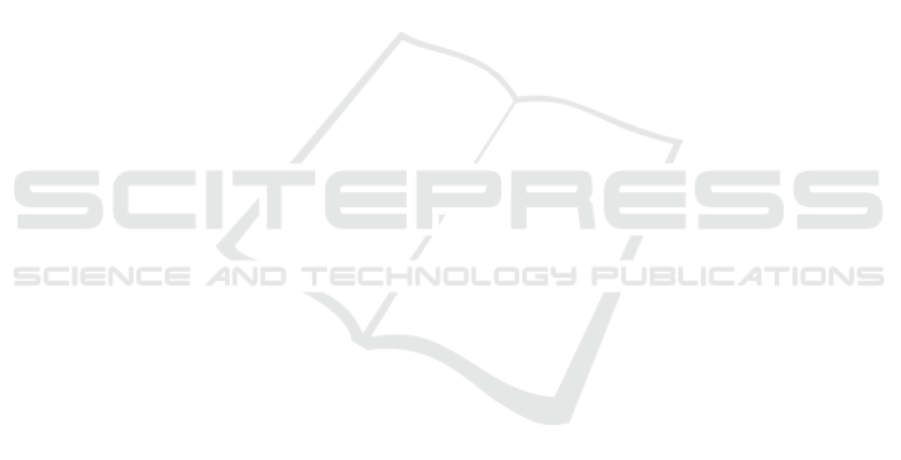
REFERENCES
Yu, J. (2012). A nonlinear kernel Gaussian mixture model
based inferential monitoring approach for fault detec-
tion and diagnosis of chemical processes, Chemical
Engineering Science, vol. 68, 1, p. 506–519.
Yu, J. (2012). A particle filter driven dynamic Gaussian
mixture model approach for complex process moni-
toring and fault diagnosis, Journal of Process Control,
vol. 22, 4 , p. 778–788.
Yu, Jianbo. (2011). Fault detection using principal
components-based Gaussian mixture model for semi-
conductor manufacturing processes, IEEE Transac-
tions on Semiconductor Manufacturing, vol. 24, 3, p.
432–444.
Larose, D. T. (2005). Discovering Knowledge in Data. An
Introduction to Data Mining. Willey.
Han, J., Kamber, M., Pei, J. (2011). Data Mining: Con-
cepts and Techniques, 3rd ed. (The Morgan Kaufmann
Series in Data Management Systems). Morgan Kauf-
mann.
Zaki, M.J., Meira Jr.. W. (2014). Data Mining and Anal-
ysis: Fundamental Concepts and Algorithms. Cam-
bridge University Press.
Calders, T., Verwer, S. (2010). Three naive Bayes ap-
proaches for discrimination-free classification. Data
Mining and Knowledge Discovery. 21(2), p. 277–292.
Zhang, G. P. (2000). Neural Networks for Classification:
A Survey. In: IEEE Transactions on System, Man,
and Cybernetics – Part C: Applications and Reviews.
30(4), November, p. 451–462.
Ishibuchi, H., Nakashima, T., Nii, M. (2000). Fuzzy If-Then
Rules for Pattern Classification. In: The Springer In-
ternational Series in Engineering and Computer Sci-
ence. 553, p. 267–295.
Berkhin, P. (2006). A Survey of Clustering Data Mining
Techniques. In: Grouping Multidimensional Data.
Eds.: J. Kogan, C. Nicholas, M. Teboulle. Springer
Berlin Heidelberg, p.25–71.
Jain, A. K. (2010). Data clustering: 50 years beyond K-
means. Pattern Recognition Letters. 31(8), p. 651–
666.
Ester, M., Kriegel, H.-P., Sander, J., Xu, X. (1996). A
density-based algorithm for discovering clusters in
large spatial databases. In: Proc. 1996 Int. Conf.
Knowledge Discovery and Data Mining (KDD’96),
Portland, OR, August, p. 226–231.
Bouveyron, C., Brunet-Saumard, C. (2014). Model-based
clustering of high-dimensional data: A review. Com-
putational Statistics & Data Analysis. 71(0), p. 52–78.
Zeng, H., Cheung, Y. (2014). Learning a mixture model
for clustering with the completed likelihood minimum
message length criterion. Pattern Recognition. 47(5),
p. 2011–2030.
Ng, S.K., McLachlan, G.J. (2014). Mixture models for clus-
tering multilevel growth trajectories. Computational
Statistics & Data Analysis. 71(0), p. 43–51.
Gupta, M. R. , Chen, Y. (2011). Theory and use of the EM
method. In: Foundations and Trends in Signal Pro-
cessing, vol. 4, 3, p. 223–296.
Boldea, O., Magnus, J. R. (2009). Maximum likelihood
estimation of the multivariate normal mixture model,
Journal of The American Statistical Association, vol.
104, 488, p. 1539–1549.
Wang, H.X., Luo, B., Zhang, Q. B., Wei, S. (2004). Estima-
tion for the number of components in a mixture model
using stepwise split-and-merge EM algorithm, Pattern
Recognition Letters, vol. 25, 16, p. 1799–1809.
McGrory, C. A., Titterington, D. M. (2009). Variational
Bayesian analysis for hidden Markov models, Aus-
tralian & New Zealand Journal of Statistics, vol. 51,
p. 227–244.
ˇ
Sm
´
ıdl, V., Quinn, A. (2006). The Variational Bayes Method
in Signal Processing, Springer-Verlag Berlin Heidel-
berg.
Fr
¨
uhwirth-Schnatter, S. (2006). Finite Mixture and Markov
Switching Models, Springer-Verlag New York.
Doucet, A., Andrieu, C. (2001). Iterative algorithms for
state estimation of jump Markov linear systems. IEEE
Transactions on Signal Processing, vol. 49, 6, p.
1216–1227.
Chen, R., Liu, J.S. (2000). Mixture Kalman filters. Journal
of the Royal Statistical Society: Series B (Statistical
Methodology), vol. 62, p. 493–508.
K
´
arn
´
y, M., Kadlec, J., Sutanto, E.L. (1998). Quasi-Bayes
estimation applied to normal mixture, In: Preprints
of the 3rd European IEEE Workshop on Computer-
Intensive Methods in Control and Data Processing
(eds. J. Roj
´
ı
ˇ
cek, M. Vale
ˇ
ckov
´
a, M. K
´
arn
´
y, K. War-
wick), CMP’98 /3./, Prague, CZ, p. 77–82.
Peterka, V. (1981). Bayesian system identification. In:
Trends and Progress in System Identification (ed. P.
Eykhoff), Oxford, Pergamon Press, 1981, p. 239–304.
K
´
arn
´
y, M., B
¨
ohm, J., Guy, T. V., Jirsa, L., Nagy, I., Ne-
doma, P., Tesa
ˇ
r, L. (2006). Optimized Bayesian Dy-
namic Advising: Theory and Algorithms, Springer-
Verlag London.
Nagy, I., Suzdaleva, E., K
´
arn
´
y, M., Mlyn
´
a
ˇ
rov
´
a, T. (2011).
Bayesian estimation of dynamic finite mixtures. Int.
Journal of Adaptive Control and Signal Processing,
vol. 25, 9, p. 765–787.
Suzdaleva, E., Nagy, I., Mlyn
´
a
ˇ
rov
´
a, T. (2015). Recursive
Estimation of Mixtures of Exponential and Normal
Distributions. In: Proceedings of the 8th IEEE In-
ternational Conference on Intelligent Data Acquisi-
tion and Advanced Computing Systems: Technology
and Applications, Warsaw, Poland, September 24–26,
p.137–142.
Yang, L., Zhou, H., Yuan, H. (2013). Bayes Estima-
tion of Parameter of Exponential Distribution under
a Bounded Loss Function. Research Journal of Math-
ematics and Statistics, vol.5, 4, p.28–31.
Casella, G., Berger R.L. (2001). Statistical Inference, 2nd
ed., Duxbury Press.
Nagy, I., Suzdaleva, E., Mlyn
´
a
ˇ
rov
´
a, T. (2016). Mixture-
based clustering non-gaussian data with fixed bounds.
In: Proceedings of the IEEE International conference
Intelligent systems IS’16, Sofia, Bulgaria, September
4–6, accepted.
ICINCO 2016 - 13th International Conference on Informatics in Control, Automation and Robotics
534